【数据库】——faiss ubuntu安装与简单测试python
简介: Faiss是Facebook AI团队开源的针对聚类和相似性搜索库,为稠密向量提供高效相似度搜索和聚类,支持十亿级别向量的搜索,是目前最为成熟的近似近邻搜索库。它包含多种搜索任意大小向量集(备注:向量集大小由RAM内存决定)的算法,以及用于算法评估和参数调整的支持代码。Faiss用C++编写,并提供与Numpy完美衔接的Python接口。除此以外,对一些核心算法提供了GPU实现。应用场景:
简介: Faiss是Facebook AI团队开源的针对聚类和相似性搜索库,为稠密向量提供高效相似度搜索和聚类,支持十亿级别向量的搜索,是目前最为成熟的近似近邻搜索库。它包含多种搜索任意大小向量集(备注:向量集大小由RAM内存决定)的算法,以及用于算法评估和参数调整的支持代码。Faiss用C++编写,并提供与Numpy完美衔接的Python接口。除此以外,对一些核心算法提供了GPU实现。
应用场景: 人脸识别,以图搜图 …
作者: Facebook
1. 新建一个conda环境
这里新建一个conda环境,用于安装各个软件,并做相应的测试。
- 新建conda环境
conda create -n faiss python==3.7.7
- 安装faiss-cpu
官方给的安装方案pytorch源下的安装。但是这速度很慢,其次容易出现:Intel MKL FATAL ERROR: Cannot load libmkl_avx.so or libmkl_def.so。这里用清华的pytorch源
conda install faiss-cpu -c pytorch
- 用清华的pytorch源安装
source activate faiss
conda install faiss-cpu --channel https://mirrors.tuna.tsinghua.edu.cn/anaconda/cloud/pytorch/
可能问题
- Intel MKL FATAL ERROR: Cannot load libmkl_avx.so or libmkl_def.so
用清华源安装faiss-cpu
3. 测试
硬件
- 用命令看cpu型号,cat /proc/cpuinfo
Intel® Core™ i7-8700K CPU @ 3.70GHz
code
import faiss
import numpy as np
import time
d = 64
nb = 100000
nq = 10000
xb = np.random.random((nb, d)).astype("float32")
xb[:, 0] += np.arange(nb) / 1000.
xq = np.random.random((nq, d)).astype("float32")
xq[:, 0] += np.arange(nq) / 1000.
import faiss # make faiss available
index = faiss.IndexFlatL2(d) # build the index
print(index.is_trained)
index.add(xb) # add vectors to the index
print(index.ntotal)
k = 4 # we want to see 4 nearest neighbors
start_t = time.time()
D, I = index.search(xb[[1,10,20,30,40]], k) # D:匹配的索引,I:D索引对应的L2距离
print("use time:",time.time() - start_t)
print(I)
print(D)
start_t = time.time()
D, I = index.search(xq, k) # actual search
print("use time:",time.time() - start_t)
print(I[:5]) # neighbors of the 5 first queries
print(I[-5:]) # neighbors of the 5 last queries
True
100000
use time: 0.0044291019439697266
[[ 1 762 167 1115]
[ 10 819 522 388]
[ 20 319 754 50]
[ 30 553 628 707]
[ 40 91 736 692]]
[[0. 5.8098564 7.0517216 7.0524645]
[0. 5.7370405 6.1728783 6.3311534]
[0. 5.8789406 6.379945 6.4565234]
[0. 6.3657794 6.4845867 6.486966 ]
[0. 6.7513127 6.7710495 6.922102 ]]
use time: 0.803516149520874
[[710 724 700 707]
[ 4 39 643 516]
[ 13 358 618 169]
[290 264 424 37]
[463 790 401 356]]
[[ 9201 9618 9177 9079]
[ 9240 10550 9507 9225]
[ 9665 9903 9887 10331]
[ 9758 9663 10844 9799]
[ 9926 8750 9507 9940]]
解析
- 看代码中的注释
- 在10w的底库中,检索1w,才0.8s。
reference
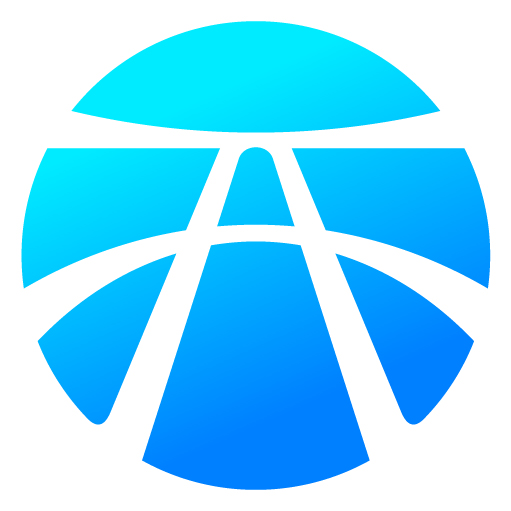
开放原子开发者工作坊旨在鼓励更多人参与开源活动,与志同道合的开发者们相互交流开发经验、分享开发心得、获取前沿技术趋势。工作坊有多种形式的开发者活动,如meetup、训练营等,主打技术交流,干货满满,真诚地邀请各位开发者共同参与!
更多推荐
所有评论(0)