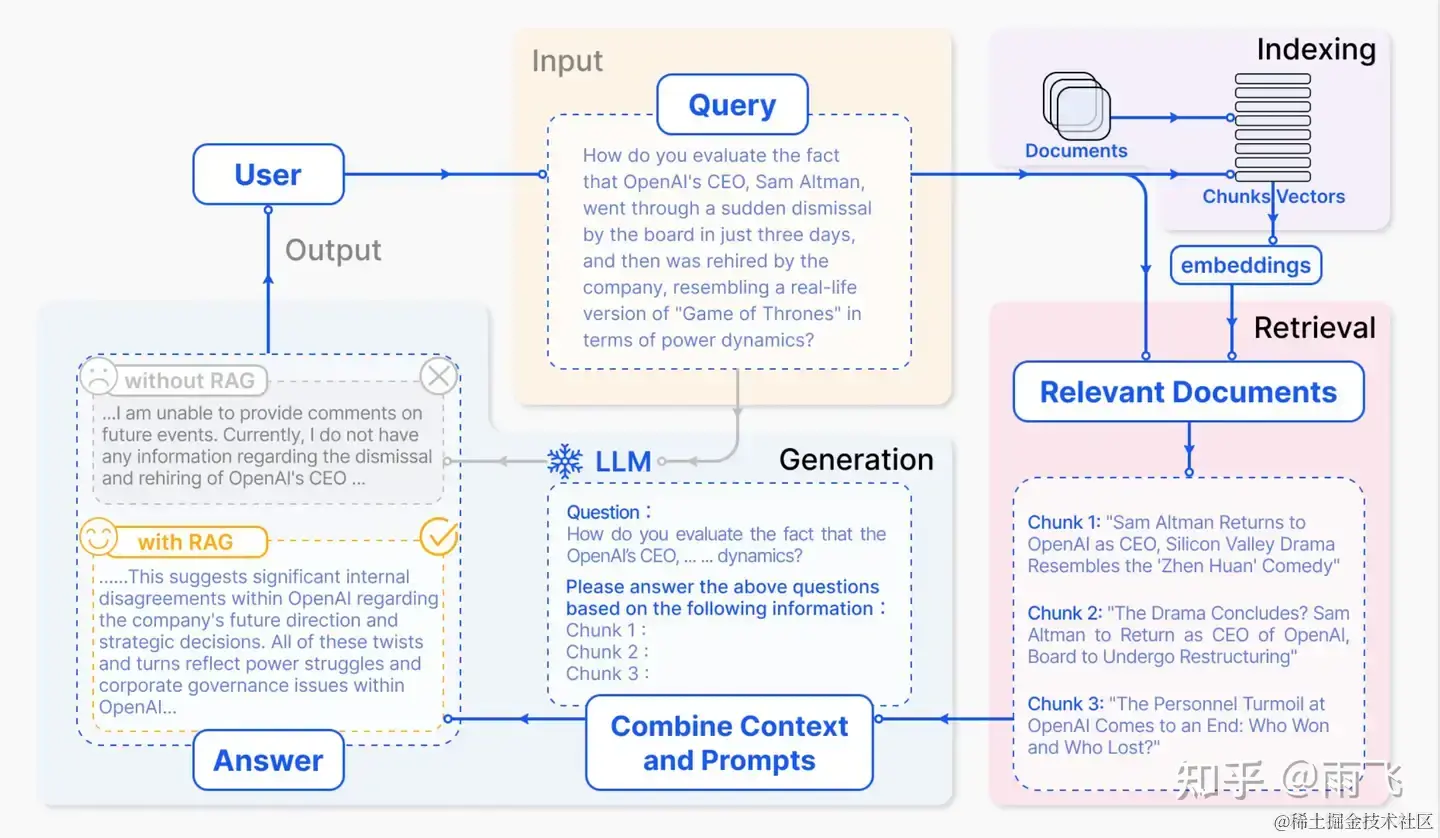
从零开始学 langchain 之搭建最小的 RAG 系统
RAG 可以说是 23 年以来到现在,最为火热的大模型应用技术了,很多人都有了很多经典的研究。而对于新人来说,有些代码十分复杂,导致只看表象并不理解其原理。今天,就利用 langchain 和大家一起搭建一个最简单的 RAG 系统,一起来学习一下吧。
RAG 可以说是 23 年以来到现在,最为火热的大模型应用技术了,很多人都有了很多经典的研究。而对于新人来说,有些代码十分复杂,导致只看表象并不理解其原理。今天,就利用 langchain 和大家一起搭建一个最简单的 RAG 系统,一起来学习一下吧。
langchain 安装
目前,langchain 的版本已经更新到 0.1.X,建议使用最新的稳定版本,不然之前的代码会出现兼容性的问题。
RAG 原理解析
RAG 的原理已经有很多文章都提到了,这里我们再复习一下,下面是从论文中截取的图,欢迎查看这篇原文。
Retrieval-Augmented Generation for Large Language Models: A Survey
从图中,我们进行进一步的拆解,可以看到,主要分为下面几个步骤:
1、索引建立,将文本数据通过向量化的模型导入到向量数据库进行存储
2、检索,根据用户的输入去检索最相关的 n 个片段
3、生成,将上下文和用户问题拼接成提示词,输入给大模型,得到最后的答案。
索引建立
我们使用 chroma 作为向量数据库去存储用户数据,并调用 BGE 的向量去完成向量化的操作。原始的数据,为了方便展示,使用了 markdown 格式的数据,可以直接用 textloader 进行加载。
详细代码如下:
python
import os
from langchain.embeddings.huggingface import HuggingFaceBgeEmbeddings
from langchain_community.document_loaders import TextLoader
from langchain.prompts import ChatPromptTemplate
from langchain.text_splitter import RecursiveCharacterTextSplitter
from langchain_community.vectorstores import Chroma
from langchain_core.output_parsers import StrOutputParser
from langchain_core.runnables import RunnablePassthrough
from model_factory import yi_llm
BGE_MODEL_PATH = "BAAI/bge-large-zh"
root_dir = "./zsxq"
def extract_file_dirs(directory):
file_paths = []
for root, dirs, files in os.walk(directory):
for file in files:
if file.endswith(".md"):
fp = os.path.join(root, file)
file_paths.append(fp)
return file_paths
files = extract_file_dirs(root_dir)
print(files)
loaders = [TextLoader(f) for f in files]
docs = []
for l in loaders:
docs.extend(l.load())
text_splitter = RecursiveCharacterTextSplitter(chunk_size=150, chunk_overlap=20)
documents = text_splitter.split_documents(docs)
huggingface_bge_embedding = HuggingFaceBgeEmbeddings(model_name=BGE_MODEL_PATH)
vectorstore = Chroma.from_documents(documents, huggingface_bge_embedding, persist_directory="./vectorstore")
query="在知识星球里,怎么快速找到最有价值的内容?"
result = vectorstore.similarity_search(query, k=3)
for doc in result:
print(doc.page_content)
print("********")
检索
我们很容易的使用下面这个语句,将向量数据库转为检索器进行使用。然后可以调用检索器的get_relevant_documents
方法去检索得到相似的文本片段,然后就可以使用 langchain 的 LCEL 语言去调用了。
python
retriever = vectorstore.as_retriever()
docs = retriever.get_relevant_documents(query)
生成
我们首先定义一个简单的提示词,将检索得到的上下文片段和用户的问题进行拼接,然后输入给大模型进行回答。为了方便最后对比各种方法的效果,我们使用了 StrOutputParser
去提取最后输出的文本。
代码如下:
python
template = """Answer the question based only on the following context:
{context}
Question: {question},请用中文输出答案。
"""
prompt = ChatPromptTemplate.from_template(template)
model = yi_llm
def format_docs(docs):
return "\n\n".join([d.page_content for d in docs])
chain = (
{"context": retriever | format_docs, "question": RunnablePassthrough()}
| prompt
| model
| StrOutputParser()
)
response = chain.invoke(query)
print(response)
print(yi_llm.invoke(query))
结果对比
RAG 输出结果
LLM 输出结果
原文
分析,从输出的结果上看,RAG 的输出命中了原文的搜索功能,但是增加了关注订阅,推荐这些原文没有提到的内容,仍然会存在幻觉。
LLM 输出的结果,看起来是对的,但实际上和原文并不相符,应该是用的自己内部的知识,也存在幻觉问题。
我们只是搭建了一个简单的示例,因此,RAG 的结果,是还有待改进的,不能立马满足我们的要求。
python
import os
from langchain.embeddings.huggingface import HuggingFaceBgeEmbeddings
from langchain_community.document_loaders import TextLoader
from langchain.prompts import ChatPromptTemplate
from langchain.text_splitter import RecursiveCharacterTextSplitter
from langchain_community.vectorstores import Chroma
from langchain_core.output_parsers import StrOutputParser
from langchain_core.runnables import RunnablePassthrough
from model_factory import yi_llm
BGE_MODEL_PATH = "BAAI/bge-large-zh"
root_dir = "./zsxq"
def extract_file_dirs(directory):
file_paths = []
for root, dirs, files in os.walk(directory):
for file in files:
if file.endswith(".md"):
fp = os.path.join(root, file)
file_paths.append(fp)
return file_paths
files = extract_file_dirs(root_dir)
print(files)
loaders = [TextLoader(f) for f in files]
docs = []
for l in loaders:
docs.extend(l.load())
text_splitter = RecursiveCharacterTextSplitter(chunk_size=150, chunk_overlap=20)
documents = text_splitter.split_documents(docs)
huggingface_bge_embedding = HuggingFaceBgeEmbeddings(model_name=BGE_MODEL_PATH)
vectorstore = Chroma.from_documents(documents, huggingface_bge_embedding, persist_directory="./vectorstore")
query="在知识星球里,怎么快速找到最有价值的内容?"
result = vectorstore.similarity_search(query, k=3)
for doc in result:
print(doc.page_content)
print("********")
retriever = vectorstore.as_retriever()
template = """Answer the question based only on the following context:
{context}
Question: {question},请用中文输出答案。
"""
prompt = ChatPromptTemplate.from_template(template)
model = yi_llm
def format_docs(docs):
return "\n\n".join([d.page_content for d in docs])
chain = (
{"context": retriever | format_docs, "question": RunnablePassthrough()}
| prompt
| model
| StrOutputParser()
)
response = chain.invoke(query)
print("RAG 输出结果:",response)
print("LLM 输出结果:",yi_llm.invoke(query).content)
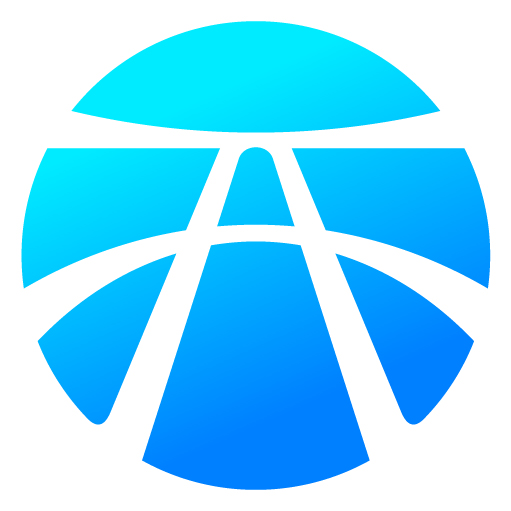
开放原子开发者工作坊旨在鼓励更多人参与开源活动,与志同道合的开发者们相互交流开发经验、分享开发心得、获取前沿技术趋势。工作坊有多种形式的开发者活动,如meetup、训练营等,主打技术交流,干货满满,真诚地邀请各位开发者共同参与!
更多推荐
所有评论(0)