pytorch model.train() 和model.eval() 对 BN 层的影响
代码】pytorch model.train() 和model.eval() 对 BN 层的影响。
·
model.train()
- BN做归一化时,使用的均值和方差是当前这个Batch的
- 如果这时
track_running_stats=True
, 则会更新running_mean
和running_var
- 但是,
running_mean
和running_var
不用在训练阶段
model.eval()
- BN 做归一化时,使用的均值和方差是BN存储的
running_mean
和running_var
- 不管这时
track_running_stats
是 True 还是 False, 都不会更新running_mean
和running_var
感兴趣可以在以下测试代码下调整测试
'''
Author: Chae Luv
Date: 2022-08-17 22:40:13
LastEditors: Chae Luv
LastEditTime: 2022-08-17 23:15:22
FilePath: /re-record-audio-watermark/10-base_model/test_bn.py
Description:
Copyright (c) 2022 by Chae Luv/USTC, All Rights Reserved.
'''
import torch
import torch.nn as nn
def create_inputs():
return torch.randn(8, 3, 20, 20)
def simulated_bn_forward(x, bn_weight, bn_bias, eps, mean_val=None, var_val=None):
if mean_val is None:
mean_val = x.mean([0, 2, 3])
if var_val is None:
var_val = x.var([0, 2, 3], unbiased=False)
x = x - mean_val[None, ..., None, None]
x = x / torch.sqrt(var_val[None, ..., None, None] + eps)
x = x * bn_weight[..., None, None] + bn_bias[..., None, None]
return mean_val, var_val, x
pytorch_bn = nn.BatchNorm2d(num_features=3, momentum=None)
running_mean = torch.zeros(3)
running_var = torch.ones_like(running_mean)
# 切换到eval模式
pytorch_bn.train(mode=False)
test_input = create_inputs()
print(f'pytorch_bn running_mean is {pytorch_bn.running_mean}')
print(f'pytorch_bn running_var is {pytorch_bn.running_var}')
bn_outputs = pytorch_bn(test_input)
print(f'Now pytorch_bn running_mean is {pytorch_bn.running_mean}')
print(f'Now pytorch_bn running_var is {pytorch_bn.running_var}')
# 用之前统计的running_mean和running_var替代输入的running_mean和running_var
_, _, simulated_outputs = simulated_bn_forward(
test_input, pytorch_bn.weight,
pytorch_bn.bias, pytorch_bn.eps,
running_mean, running_var)
assert torch.allclose(simulated_outputs, bn_outputs)
# 关闭track_running_stats后,即使在eval模式下,也会去计算输入的mean和var
pytorch_bn.train(mode=True)
pytorch_bn.track_running_stats = False
bn_outputs_notrack = pytorch_bn(test_input)
_, _, simulated_outputs_notrack = simulated_bn_forward(
test_input, pytorch_bn.weight,
pytorch_bn.bias, pytorch_bn.eps)
print(torch.sum(simulated_outputs_notrack - bn_outputs_notrack))
assert torch.allclose(simulated_outputs_notrack, bn_outputs_notrack)
assert not torch.allclose(bn_outputs, bn_outputs_notrack)
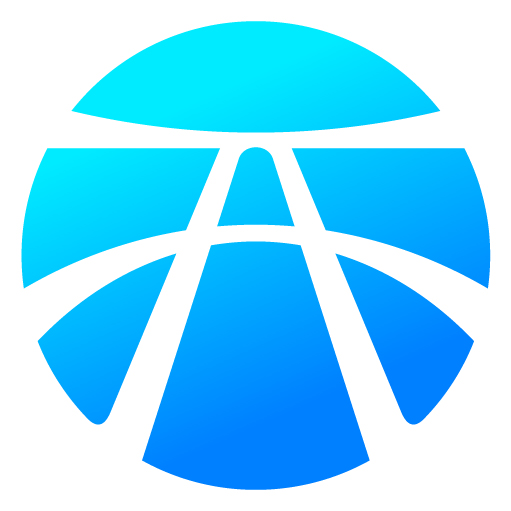
开放原子开发者工作坊旨在鼓励更多人参与开源活动,与志同道合的开发者们相互交流开发经验、分享开发心得、获取前沿技术趋势。工作坊有多种形式的开发者活动,如meetup、训练营等,主打技术交流,干货满满,真诚地邀请各位开发者共同参与!
更多推荐
所有评论(0)