数据挖掘中的大数据量分批增量训练
aa
1、lightgbm
提升机器算法LightGBM(图解+理论+增量训练python代码+lightGBM调参方法)
增量训练python代码
这个我好像代码在另一个电脑上,待更吧。。。星期一把代码完善一下。。。先简单介绍一下什么叫增量训练,就是他一下子吃不了那么多数据,内存会爆掉,但是需要读怎么办,就有一个流式读取的方法,本质上是个迭代器。。。
每次读取文件的一部分,用于训练模型,并保存模型的训练结果;然后读取文件的另一部分,再对模型进行更新训练;迭代读取全部数据完毕,最终完成整个文件数据的训练过程。
1. 文件的流式读取
-
def iter_minibatches(minibatch_size=1000):
-
'''
-
迭代器
-
给定文件流(比如一个大文件),每次输出minibatch_size行,默认选择1k行
-
将输出转化成numpy输出,返回X, y
-
'''
-
X = []
-
y = []
-
cur_line_num = 0
-
train_data, train_label, train_weight, test_data, test_label, test_file = load_data()
-
train_data, train_label = shuffle(train_data, train_label, random_state=0) # random_state=0用于记录打乱位置 保证每次打乱位置不变
-
print(type(train_label), train_label)
-
for data_x, label_y in zip(train_data, train_label):
-
X.append(data_x)
-
y.append(label_y)
-
cur_line_num += 1
-
if cur_line_num >= minibatch_size:
-
X, y = np.array(X), np.array(y) # 将数据转成numpy的array类型并返回
-
yield X, y
-
X, y = [], []
-
cur_line_num = 0
2. lightgbm(LGB)增量训练过程
-
def lightgbmTest():
-
import lightgbm as lgb
-
# 第一步,初始化模型为None,设置模型参数
-
gbm = None
-
params = {
-
'task': 'train',
-
'application': 'regression', # 目标函数
-
'boosting_type': 'gbdt', # 设置提升类型
-
'learning_rate': 0.01, # 学习速率
-
'num_leaves': 50, # 叶子节点数
-
'tree_learner': 'serial',
-
'min_data_in_leaf': 100,
-
'metric': ['l1', 'l2', 'rmse'], # l1:mae, l2:mse # 评估函数
-
'max_bin': 255,
-
'num_trees': 300
-
}
-
# 第二步,流式读取数据(每次10万)
-
minibatch_train_iterators = iter_minibatches(minibatch_size=10000)
-
for i, (X_, y_) in enumerate(minibatch_train_iterators):
-
# 创建lgb的数据集
-
# y_ = list(map(float, y_)) # 将numpy.ndarray转变为list
-
X_train, X_test, y_train, y_test = train_test_split(X_, y_, test_size=0.1, random_state=0)
-
y_train = y_train.ravel()
-
lgb_train = lgb.Dataset(X_train, y_train)
-
lgb_eval = lgb.Dataset(X_test, y_test, reference=lgb_train)
-
# 第三步:增量训练模型
-
# 重点来了,通过 init_model 和 keep_training_booster 两个参数实现增量训练
-
gbm = lgb.train(params,
-
lgb_train,
-
num_boost_round=1000,
-
valid_sets=lgb_eval,
-
init_model=gbm, # 如果gbm不为None,那么就是在上次的基础上接着训练
-
# feature_name=x_cols,
-
early_stopping_rounds=10,
-
verbose_eval=False,
-
keep_training_booster=True) # 增量训练
-
print("{} time".format(i)) # 当前次数
-
# 输出模型评估分数
-
score_train = dict([(s[1], s[2]) for s in gbm.eval_train()])
-
print('当前模型在训练集的得分是:mae=%.4f, mse=%.4f, rmse=%.4f'
-
% (score_train['l1'], score_train['l2'], score_train['rmse']))
-
return gbm
3. lightgbm(LGB)调用过程以及保存训练结果模型
-
'''lightgbm增量训练'''
-
print('lightgbm增量训练')
-
train_data, train_label, train_weight, test_data, test_label, test_file = load_data()
-
print(train_label.shape,train_data.shape)
-
train_X, test_X, train_Y, test_Y = train_test_split(train_data, train_label, test_size=0.1, random_state=0)
-
# train_X, train_Y = shuffle(train_data, train_label, random_state=0) # random_state=0用于记录打乱位置 保证每次打乱位置不变
-
gbm = lightgbmTest()
-
pred_Y = gbm.predict(test_X)
-
print('compute_loss:{}'.format(compute_loss(test_Y, pred_Y)))
-
# gbm.save_model('lightgbmtest.model')
-
# 模型存储
-
joblib.dump(gbm, 'loan_model.pkl')
-
# 模型加载
-
gbm = joblib.load('loan_model.pkl')
2、catboost
fit - CatBoostClassifier | CatBoost
fit(X, y=None, cat_features=None, text_features=None, embedding_features=None, sample_weight=None, baseline=None, use_best_model=None, eval_set=None, verbose=None, logging_level=None plot=False, column_description=None, verbose_eval=None, metric_period=None, silent=None, early_stopping_rounds=None, save_snapshot=None, snapshot_file=None, snapshot_interval=None, init_model=None, log_cout=sys.stdout, log_cerr=sys.stderr)
init_model
Description
The description is different for each group of possible types.
Possible types
The model to continue learning from.
Note
The initial model must have the same problem type as the one being solved in the current training (binary classification, multiclassification or regression/ranking).
None (incremental learning is not used)CPU
{{ [catboost.CatBoost](../concepts/python-reference_catboost.md), catboost.CatBoostClassifier](../concepts/python-reference_catboostclassifier.md) }}
The initial model object.
string
The path to the input file that contains the initial model.
Default value
None (incremental learning is not used)
Supported processing units
CPU
3、XGBoost
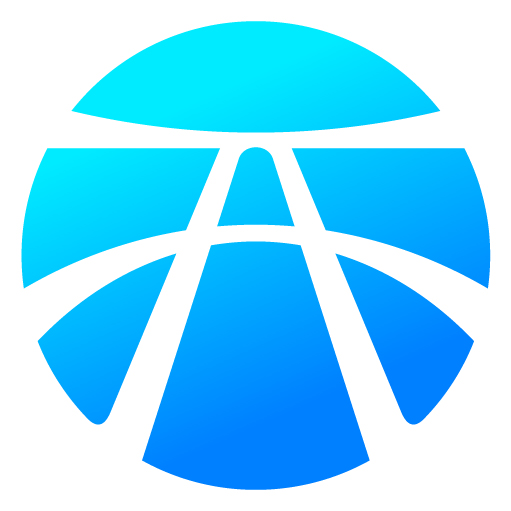
开放原子开发者工作坊旨在鼓励更多人参与开源活动,与志同道合的开发者们相互交流开发经验、分享开发心得、获取前沿技术趋势。工作坊有多种形式的开发者活动,如meetup、训练营等,主打技术交流,干货满满,真诚地邀请各位开发者共同参与!
更多推荐
所有评论(0)