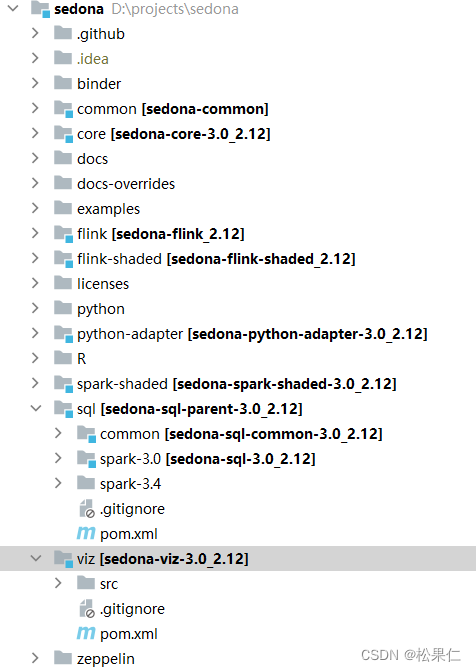
GIS大数据处理框架sedona(塞多纳)编程入门指导
是一个用于处理大规模空间数据的集群计算系统。Sedona扩展了现有的集群计算系统,如Apache Spark和Apache Flink,使用一组开箱即用的分布式空间数据集和空间SQL,可以有效地加载、处理和分析跨机器的大规模空间数据。
·
GIS大数据处理框架sedona(塞多纳)编程入门指导
简介
Apache Sedona™是一个用于处理大规模空间数据的集群计算系统。Sedona扩展了现有的集群计算系统,如Apache Spark和Apache Flink,使用一组开箱即用的分布式空间数据集和空间SQL,可以有效地加载、处理和分析跨机器的大规模空间数据。码云镜像 码云sedona文档持续更新中
代码结构
- common java核心包,对底层JTS、geotools坐标系转换等操作方法的接口包装,并提供了circle(扩展JTS功能),距离计算方法:Haversine方式,Spheroid椭球;WKT,GeoJSON等格式转换;索引支持QUADTREE,RTREE;geohash计算;供spark、flink等上层应用调用使用
- core 与spark适配核心包,封装提供基础对象SpatialRDD,PointRDD,LineStingRDD,CircleRDD,PolygonRDD;几何链接操作joinJudgement(通过几何拓扑关系),knnJudgement(几何距离),rangeJudgement(treeIndex索引范围查询);数据读取转换formatMapper:cvs,wkt,geoJson,shapefile,netcdf;spatialPartitioning分区器:QuadtreePartitioning,KDBTreePartitioner等
- flink flink适配,调用common下的functions里面提供的函数方法
- python-adapter python适配,调用common下的functions里面提供的函数方法
- sql spark-sql适配,调用common下的functions里面提供的函数方法
使用说明
在spark下面的使用说明
1.安装
<dependency>
<groupId>org.apache.sedona</groupId>
<artifactId>sedona-spark-shaded-3.0_2.12</artifactId>
<version>1.4.0</version>
</dependency>
<dependency>
<groupId>org.apache.sedona</groupId>
<artifactId>sedona-viz-3.0_2.12</artifactId>
<version>1.4.0</version>
</dependency>
<!-- Optional: https://mvnrepository.com/artifact/org.datasyslab/geotools-wrapper -->
<dependency>
<groupId>org.datasyslab</groupId>
<artifactId>geotools-wrapper</artifactId>
<version>1.4.0-28.2</version>
</dependency>
2.初始化SparkSession
SparkSession sparkSession = SparkSession.builder()
.master("local[*]") // Delete this if run in cluster mode
.appName("readTestScala") // Change this to a proper name
// Enable Sedona custom Kryo serializer
.config("spark.serializer", KryoSerializer.class.getName) // org.apache.spark.serializer.KryoSerializer
.config("spark.kryo.registrator", SedonaKryoRegistrator.class.getName)
.getOrCreate() // org.apache.sedona.core.serde.SedonaKryoRegistrator
3.安装函数
SedonaSQLRegistrator.registerAll(sparkSession)
4.使用例子
4.1 dataFrame方式加载数据
4.1.1 从文件加载数据
假设有一个WKT数据格式的tsv文件,存储位置/Download/usa-county.tsv
POLYGON (..., ...) Cuming County
POLYGON (..., ...) Wahkiakum County
POLYGON (..., ...) De Baca County
POLYGON (..., ...) Lancaster County
加载
Dataset<Row> rawDf = sparkSession.read.format("csv").option("delimiter", "\t").option("header", "false").load("/Download/usa-county.tsv")
rawDf.createOrReplaceTempView("rawdf")
rawDf.show()
结果展示
| _c0|_c1|_c2| _c3| _c4| _c5| _c6|_c7|_c8| _c9|_c10| _c11|_c12|_c13| _c14| _c15| _c16| _c17|
+--------------------+---+---+--------+-----+-----------+--------------------+---+---+-----+----+-----+----+----+----------+--------+-----------+------------+
|POLYGON ((-97.019...| 31|039|00835841|31039| Cuming| Cuming County| 06| H1|G4020|null| null|null| A|1477895811|10447360|+41.9158651|-096.7885168|
|POLYGON ((-123.43...| 53|069|01513275|53069| Wahkiakum| Wahkiakum County| 06| H1|G4020|null| null|null| A| 682138871|61658258|+46.2946377|-123.4244583|
|POLYGON ((-104.56...| 35|011|00933054|35011| De Baca| De Baca County| 06| H1|G4020|null| null|null| A|6015539696|29159492|+34.3592729|-104.3686961|
|POLYGON ((-96.910...| 31|109|00835876|31109| Lancaster| Lancaster County| 06| H1|G4020| 339|30700|null| A|2169240202|22877180|+40.7835474|-096.6886584|
4.1.1 通过ST_函数
SELECT ST_GeomFromWKT(_c0) AS countyshape, _c1, _c2
4.1.3 从GeoJSON文件读取
String schema = "type string, crs string, totalFeatures long, features array<struct<type string, geometry string, properties map<string, string>>>";
sparkSession.read.schema(schema).json(geojson_path)
.selectExpr("explode(features) as features") // Explode the envelope to get one feature per row.
.select("features.*") // Unpack the features struct.
.withColumn("geometry", expr("ST_GeomFromGeoJSON(geometry)")) // Convert the geometry string.
.printSchema();
4.1.4 从数据库读取
// For any JDBC data source, inluding Postgis.
Dataset<Row> df = sparkSession.read().format("jdbc")
// Other options.
.option("query", "SELECT id, ST_AsBinary(geom) as geom FROM my_table")
.load()
.withColumn("geom", expr("ST_GeomFromWKB(geom)"))
// This is a simplified version that works for Postgis.
Dataset<Row> df = sparkSession.read().format("jdbc")
// Other options.
.option("dbtable", "my_table")
.load()
.withColumn("geom", expr("ST_GeomFromWKB(geom)"))
4.2 CRS(坐标系)转换
SELECT ST_Transform(countyshape, "epsg:4326", "epsg:3857") AS newcountyshape, _c1, _c2, _c3, _c4, _c5, _c6, _c7
FROM spatialdf
4.3 地理空间查询
4.3.1 范围查询
ST_Contains, ST_Intersects, ST_Within
SELECT *
FROM spatialdf
WHERE ST_Contains (ST_PolygonFromEnvelope(1.0,100.0,1000.0,1100.0), newcountyshape)
4.3.2 距离查询
ST_Distance
SELECT countyname, ST_Distance(ST_PolygonFromEnvelope(1.0,100.0,1000.0,1100.0), newcountyshape) AS distance
FROM spatialdf
ORDER BY distance DESC
LIMIT 5
4.3.3 关联查询
SELECT *
FROM polygondf, pointdf
WHERE ST_Contains(polygondf.polygonshape,pointdf.pointshape)
SELECT *
FROM polygondf, pointdf
WHERE ST_Intersects(polygondf.polygonshape,pointdf.pointshape)
SELECT *
FROM pointdf, polygondf
WHERE ST_Within(pointdf.pointshape, polygondf.polygonshape)
SELECT *
FROM pointdf1, pointdf2
WHERE ST_Distance(pointdf1.pointshape1,pointdf2.pointshape2) < 2
5 存储
已入postgis为例
my_postgis_db# create table my_table (id int8, geom geometry);
df.withColumn("geom", expr("ST_AsEWKB(geom)")
.write.format("jdbc")
.option("truncate","true") // Don't let Spark recreate the table.
// Other options.
.save()
// If you didn't create the table before writing you can change the type afterward.
my_postgis_db# alter table my_table alter column geom type geometry;
6 SpatialRDD与DataFrame相好转换
6.1 SpatialRDD转DataFrame
Dataset<Row> spatialDf = Adapter.toDf(spatialRDD, sparkSession)
6.2 DataFrame转SpatialRDD
val schema = StructType(Array(
StructField("county", GeometryUDT, nullable = true),
StructField("name", StringType, nullable = true),
StructField("price", DoubleType, nullable = true),
StructField("age", IntegerType, nullable = true)
))
val spatialDf = Adapter.toDf(spatialRDD, schema, sparkSession)
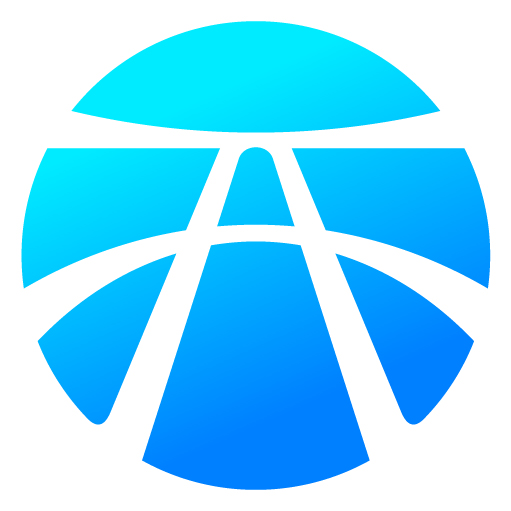
开放原子开发者工作坊旨在鼓励更多人参与开源活动,与志同道合的开发者们相互交流开发经验、分享开发心得、获取前沿技术趋势。工作坊有多种形式的开发者活动,如meetup、训练营等,主打技术交流,干货满满,真诚地邀请各位开发者共同参与!
更多推荐
所有评论(0)