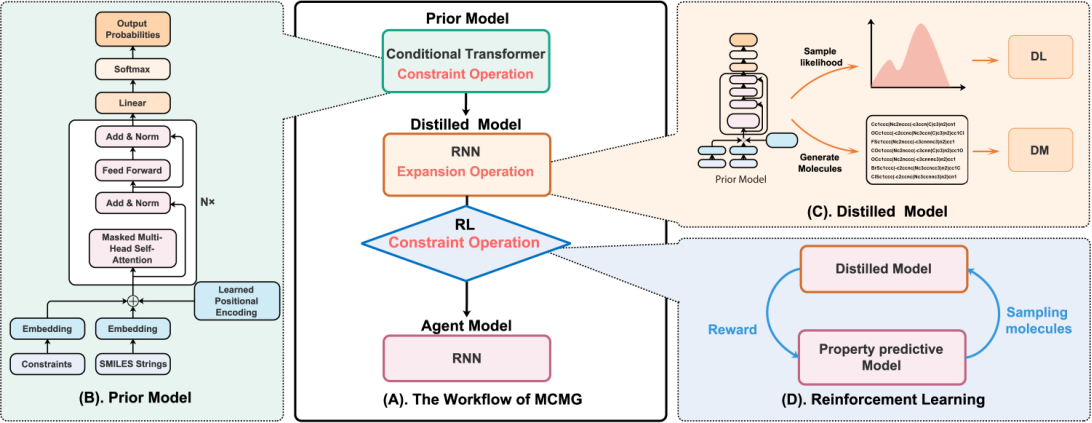
2024-基于人工智能的药物设计方法研究-AIDD
*图 2. ADMETlab 2.0的预测结果展示页面。
·
AIDD docx
基于人工智能的药物设计方法研究
- AI作为一种强大的数据挖掘和分析技术已经涉及新药研发的各个阶段,有望推动创新药物先导分子的筛选、设计和发现,但基于AI的数据驱动式创新药物设计和筛选方法仍存在若干亟待解决的问题。我们课题组的核心研究方向是围绕药物设计中的难点问题,应用大数据和AI技术发展高精度及高效的活性分子虚拟筛选方法、成药性预测方法、全新结构设计方法、逆合成预测方法以及靶标预测方法等,并开发整合的高精度药物设计和筛选软件平台。例如,我们开发了基于知识图谱和推荐系统的药物-靶标相互作用预测方法KGE-NFM,其在多个基准数据集上比传统方法表现出更优的预测精度 ;发展了基于Transformer、知识蒸馏(knowledge distillation)和强化学习的多约束分子生成新方法MCMG,其可以高效遍历复杂的化学空间以寻找满足多种性质约束的新型化合物,为先导结构的发现提供了功能强大的计算工具(图1);发展了一种新型的基于图表示学习的蛋白-小分子相互作用的打分方法IGN,其性能显著优于同类机器学习打分方法和分子对接程序 ;系统研究了机器学习打分函数中的若干关键问题,开发了蛋白-小分子相互作用特征提取、个性化打分函数构建以及虚拟筛选在线计算平台ASFP;创造性提出了计算生物靶标谱(CBFP)的概念,并将其作为新型分子表征实现骨架跃迁的新思路,成功发现具有全新骨架的强效PARP1抑制剂和DprE1抑制剂,证明其与传统化学表征相比具有更优的新骨架发现能力。
图 1. MCMG方法的工作流程图。
- 开发出一系列ADMET和成药性理论预测方法和模型,包括水溶性(logS)、油/水分配系数(logP)、Caco-2渗透性、肠吸收、口服生物利用度、hERG心脏毒性、血脑屏障(BBB)、P-gp蛋白转运、孕烷X受体激活、呼吸毒性、泌尿系统毒性、急性毒性、频繁命中化合物、类药性等 [11~16];针对小样本成药性数据预测精度差的难题,运用迁移学习、多任务学习、自监督学习等新型AI技术发展了快速精准的成药性预测方法(如多任务图注意力框架MGA和基于预训练的图网络模型MG-BERT和K-BERT),有效提升了多个成药性任务的预测精度,特别是对于样本较少的任务提升幅度更为显著 [17~19];在长期方法学研究基础上,开发了关键成药性参数在线软件平台ADMETLab2.0,可实现17种物化性质、13种药物化学性质、24种成药性参数、27种毒性性质及8种毒性团规则的系统性评价,是目前国际上成药性参数评价最多、速度最快、预测精度最为优秀的免费在线计算平台之一 。
**图 2. ADMETlab 2.0的预测结果展示页面。**https://admetmesh.scbdd.com/
相关论文:
- Qing Ye, Chang-Yu Hsieh, Ziyi Yang, Yu Kang, Jiming Chen, Dongsheng Cao*, Shibo He*, Tingjun Hou*, A unified drug-target interaction prediction framework based on knowledge graph and recommendation system, Nature Communications, 2021, 12, 6775.
- Jike Wang, Chang-Yu Hsieh, Mingyang Wang, Xiaorui Wang, Zhenxing Wu, Dejun Jiang, Benben Liao, Xujun Zhang, Bo Yang, Qiaojun He, Dongsheng Cao*, Xi Chen*, Tingjun Hou*, Multi-constraint molecular generation based on conditional transformer, knowledge distillation and reinforcement learning, Nature Machine Intelligence, 2021, 3, 914-922.
- Mingyang Wang, Zhe Wang, Huiyong Sun, Jike Wang, Chao Shen, Gaoqi Weng, Xin Chai, Honglin Li*, Dongsheng Cao*, Tingjun Hou*, Deep learning approaches for de novo drug design: an overview, Current Opinion in Structural Biology, 2022, 72, 135-144.
- Dejun Jiang, Chang-Yu Hsieh, Zhenxing Wu, Yu Kang, Jike Wang, Ercheng Wang, Ben Liao, Chao Shen, Lei Xu, Jian Wu*, Dongsheng Cao*, Tingjun Hou*, InteractionGraphNet: a novel and efficient deep graph representation learning framework for accurate protein-ligand interaction predictions, Journal of Medicinal Chemistry, 2021, 64, 18209-18232.
- Chao Shen, Ye Hu, Zhe Wang, Xujun Zhang, Haiyang Zhong, Gaoang Wang, Xiaojun Yao, Lei Xu, Dongsheng Cao*, Tingjun Hou*, Can machine learning consistently improve the scoring power of classical scoring functions? Insights into the role of machine learning in scoring functions, Briefings in Bioinformatics, 2021, 22, 497-514.
- Chao Shen, Ye Hu, Zhe Wang, Xujun Zhang, Jinping Pang, Gaoang Wang, Haiyang Zhong, Lei Xu, Dongsheng Cao*, Tingjun Hou*, Beware of the generic machine learning-based scoring functions in structure-based virtual screening, Briefings in Bioinformatics, 2021, 22, bbaa070.
- Chao Shen, Junjie Ding, Zhe Wang, Dongsheng Cao, Xiaoqin Ding, Tingjun Hou*, From machine learning to deep learning: advances in scoring functions for computational docking, WIRES Computational Molecular Science, 2020, 10, e1429.
- Xujun Zhang, Chao Shen, Xueying Guo, Zhe Wang, Gaoqi Weng, Qing Ye, Gaoang Wang, Qiaojun He, Bo Yang*, Dongsheng Cao*, Tingjun Hou*, ASFP (Artificial Intelligence based Scoring Function Platform): a web server for the development of customized scoring functions, Journal of Cheminformatics, 2021, 13, 6.
- Guoli Xiong, Yue Zhao, Lu Liu, Zhongye Ma, Aiping Lu, Yan Cheng, Tingjun Hou*, Dongsheng Cao*, Computational bioactivity fingerprint similarities to navigate the discovery of novel scaffolds, Journal of Medicinal Chemistry, 2021, 64, 7544-7554.
- Xueping Hu, Liu Yang, Xin Chai, Yixuan Lei, Md Shah Alam, Lu Liu, Chao Shen, Dejun Jiang, Zhe Wang, Zhiyong Liu, Lei Xu, Kanglin Wan, Tianyu Zhang, Yuelan Yin, Dongsheng Cao*, Dan Li*, Tingjun Hou*, Discovery of novel DprE1 inhibitors via computational bioactivity fingerprints and structure-based virtual screening, Acta Pharmacologica Sinica, 2022, in press.
- Sheng Tian, Junmei Wang, Youyong Li, Dan Li, Lei Xu, Tingjun Hou*, The application of in silico drug-likeness predictions in pharmaceutical research, Advanced Drug Delivery Reviews, 2015, 86, 2-10.
-
- Tailong Lei, Huiyong Sun, Yu Kang, Feng Zhu. Hui Liu, Wenfang Zhou, Zhe Wang, Dan Li, Youyong Li, Tingjun Hou*, ADMET evaluation in drug discovery. 18. Reliable prediction of chemical-induced urinary tract toxicity by boosting machine learning approaches, Molecular Pharmaceutics,2017, 14, 3935-3953.
- Tailong Lei, Fu Chen, Hui Liu, Huiyong Sun, Yu Kang, Dan Li, Youyong Li, Tingjun Hou*, ADMET evaluation in drug discovery. 17. Development of quantitative and qualitative prediction models for chemical-induced respiratory toxicity, Molecular Pharmaceutics, 2017, 14, 2407-2421.
- Zhenxing Wu, Tailong Lei, Chao Shen, Zhe Wang, Dongsheng Cao, Tingjun Hou*, ADMET Evaluation in Drug Discovery. 19. Reliable Prediction of Human Cytochrome P450 Inhibition Using Artificial Intelligence Approaches, Journal of Chemical Information and Modeling, 2019, 59, 4587-4601.
-
- Ziyi Yang, Junhong He, Aiping Lu, Tingjun Hou*, Dongsheng Cao*, The Application of Negative Design to Design More Desirable Virtual Screening Library, Journal of Medicinal Chemistry, 2020, 63, 4411-4429.
- Tailong Lei, Huiyong Sun, Yu Kang, Feng Zhu. Hui Liu, Wenfang Zhou, Zhe Wang, Dan Li, Youyong Li, Tingjun Hou*, ADMET evaluation in drug discovery. 18. Reliable prediction of chemical-induced urinary tract toxicity by boosting machine learning approaches, Molecular Pharmaceutics, 2017, 14, 3935-3953.
- Zhenxing Wu, Dejun Jiang, Jike Wang, Chang-Yu Hsieh*, Dongsheng Cao*, Tingjun Hou*, Mining Toxicity Information from Large Amounts of Toxicity Data, Journal of Medicinal Chemistry, 2021, 64, 6924-6936.
- Xiaochen Zhang, Chengkun Wu, Zhijiang Yang, Zhenxing Wu, Jiacai Yi, Changyu Hsieh, Tingjun Hou*, Dongsheng Cao*, MG-BERT: leveraging unsupervised atomic representation learning for molecular property prediction, Briefings in Bioinformatics, 2021, 22, bbab152.
- Zhenxing Wu, Dejun Jiang, Jike Wang, Xujun Zhang, Hongyan Du, Lurong Pan, Changyu Hsieh*, Dongsheng Cao*, Tingjun Hou*, Knowledge-based BERT: a method to extract molecular features like computational chemists, Briefings in Bioinformatics, 2022, bbac131.
- Guoli Xiong, Zhenxing Wu, Jiacai Yi, Li Fu, Zhijiang Yang, Changyu Hsieh, Mingzhu Yin, Xiangxiang Zeng, Chengkun Wu, Aiping Lu, Xiang Chen, Tingjun Hou*, Dongsheng Cao*, ADMETlab 2.0: an integrated online platform for accurate and comprehensive predictions of ADMET properties, Nucleic Acids Research, 2021, 49(W1), W5-W14.
1. 浙江大学侯廷军教授-基于AI的药物发现:机遇与挑战
- 地址 - https://www.bilibili.com/video/BV1oG411K7Ly/?buvid=XX2866E2E16E062B48BF8F07298697EEE792C&from_spmid=search.search-result.0.0&is_story_h5=false&mid=WD4dPZBHv0EX229RJlaJhw%3D%3D&p=1&plat_id=114&share_from=ugc&share_medium=android&share_plat=android&share_session_id=f32f0330-61fb-4c78-a80d-54c101e3f796&share_source=QQ&share_tag=s_i&spmid=united.player-video-detail.0.0×tamp=1712726140&unique_k=hQDWEoF&up_id=347009606&vd_source=862ff6877c847eb289270ce386f12850
- 实验室-http://cadd.zju.edu.cn/
- 软件开发-http://cadd.zju.edu.cn/program
2. 蒋华良院士在首届AI药物研发研讨会上的汇报
- 地址:https://www.bilibili.com/video/BV1HP4y1W7aT/?buvid=XX2866E2E16E062B48BF8F07298697EEE792C&from_spmid=search.search-result.0.0&is_story_h5=false&mid=WD4dPZBHv0EX229RJlaJhw%3D%3D&p=1&plat_id=114&share_from=ugc&share_medium=android&share_plat=android&share_session_id=29fbfec8-5b65-4450-a7af-35754687a7cf&share_source=QQ&share_tag=s_i&spmid=united.player-video-detail.0.0×tamp=1712726182&unique_k=KgSOCR9&up_id=383170833&vd_source=862ff6877c847eb289270ce386f12850
3. Drug Design
- 地址:https://www.icourse163.org/learn/FUDAN1-1461262164?tid=1472329514#/learn/content?type=detail&id=1258207208&sm=1
4. 药物设计学
- 地址:https://www.icourse163.org/learn/FUDAN-1003316002?tid=1472102559#/learn/content?type=detail&id=1257573178&sm=1
5. 计算机辅助药物设计
- 地址:https://www.icourse163.org/learn/JNU-1207113806?tid=1472080457#/learn/content?type=detail&id=1257317530&cid=1290679998
数据库 | |
---|---|
PROTAC-DB | 描述: 第一个可通过网络访问的 PROTAC 数据库链接:http://cadd.zju.edu.cn/protacdb/引文:核酸研究,2021,49(D1),D1381-D1387;核酸研究, 2023, 51(D1), D1367-D1372 |
共价InDB | 描述: 最大的共价抑制剂和相关靶标的网络可访问资源链接:http://cadd.zju.edu.cn/cidb/引文:核酸研究,49(D1),D1122–D1129 |
国际米兰 | 描述:专门针对药物间相互作用的全面、专业、开放访问的数据库链接:http://ddinter.scbdd.com/引文:核酸研究,2022,50(D1):D1200-D1207 |
VGSC | 描述: 最大的专业电压门控钠通道在线数据库链接:http://cadd.zju.edu.cn/vgsc/引用: J Cheminformatics , 2022, 14, 75 |
PKKB | 描述:最广泛的免费数据库,用于收集 ADME(吸收、分布、代谢和排泄)和毒性特性链接:http://cadd.zju.edu.cn/pkkb/引用:化学信息与建模杂志, 2012, 52(5), 1132-1137 |
分子表征 | |
生物医学研究 | 描述:用于生物医学研究中集成数据分析管道的 R/CRAN 包链接:https://cran.r-project.org/web/packages/BioMedR/引文:生物信息学简报,2021, 22(1): 474-484 |
窥探 | 描述:一个集成的负设计 Python 库,用于理想的 HTS/VS 数据库设计链接:https://github.com/kotori-y/Scopy/引文:生物信息学简报,2021,22(3):bbaa194 |
皮斯马什 | 描述:用于代表性子结构生成和应用的Python包和单独的可执行程序链接:https://github.com/kotori-y/pySmash/引文:生物信息学简报,2021,22(5):bbab017 |
QSAR建模 | |
米伽 | 描述:从大量毒性数据中挖掘毒性信息链接:https://github.com/wzxxxx/MGA/引文:药物化学杂志, 2021, 64(10), 6924-6936 |
禁止 | 描述: Learning to SMILES:基于 BAN 的策略来改进分子的潜在表示学习链接:https://github.com/zhang-xuan1314/SMILES-BiLSTM-attention-network/引用:生物信息学简报,2021, 22(6), bbab327 |
MG-BERT | 描述:利用无监督原子表示学习进行分子特性预测链接:https://github.com/zhang-xuan1314/Molecular-graph-BERT/引用:生物信息学简报,2021,22(6),bbab152 |
HRGCN+ | 描述:双曲关系图卷积网络plus:一种简单但高效的QSAR建模方法链接:https://quantum.tencent.com/hrgcn/引用:生物信息学简报,2021,22(5),bbab112 |
成药性预测 | |
ADMET实验室2.0 | 描述:广泛使用的 ADMETlab 的增强版,用于系统评估 ADMET 特性以及一些理化特性和药物化学友好性链接:https://admetmesh.scbdd.com/引文:核酸研究,2021, 49, W5-W14 |
化学聚合物 | 描述:一个免费的网络服务器,可用于轻松过滤掉潜在先导分子中的聚合器链接:https://admet.scbdd.com/ChemAGG/index/引文:化学信息与建模杂志,2019, 59(9), 3714-3726 |
化学荧光 | 描述:一个公共网络服务器,可用于标记大型数据集中的蓝色和绿色荧光化合物链接:https://admet.scbdd.com/chemfluo/index/引用:生物信息学简报,2021, 22(4), bbaa282 |
竞赛 | 描述:一个免费的网络服务器,用于基于图注意力的综合可访问性评估(GASA)链接:http://cadd.zju.edu.cn/gasa/引文:化学信息与建模杂志,2022, 62(12), 2973-2986 |
药物记录 | 描述: 免费向公众分发的用于估计水溶性的程序链接:http://modem.ucsd.edu/adme/引用:化学信息与计算机科学杂志, 2004, 44(1), 266-275 |
蛋白-配体打分 | |
金属ProGNet | 描述:用于金属蛋白-配体相互作用预测的基于结构的深度图模型链接:https://github.com/zjujdj/MetalProGNet/引文:化学科学, 2023, 14(8), 2054-2069 |
RTMS评分 | 描述:基于残基原子距离似然势和图形转换器的蛋白质配体评分函数链接:https://github.com/sc8668/RTMScore/引文:药物化学杂志, 2022, 65(15), 10691-10706 |
IGN | 描述:用于蛋白质-配体相互作用预测的深度图表示学习框架链接:https://github.com/zjujdj/InteractionGraphNet/tree/master/引文:药物化学杂志, 2021, 64(24), 18209-18232 |
ASFP | 描述:用于开发定制评分功能的网络服务器链接:http://cadd.zju.edu.cn/plic/引文:化学信息学杂志,2021, 13(1), 6 |
结合自由能预测 | |
咖啡店 | 描述:一个 VMD 插件,用于使用端点自由能方法进行结合亲和力预测链接:https://github.com/HuiLiuCode/CaFE_Plugin/引文:生物信息学,2016, 32(14), 2216-2218 |
快速DRH | 描述:基于分子对接和 MM/PB(GB)SA 计算来预测和分析蛋白质-配体复合物的网络服务器链接:http://cadd.zju.edu.cn/fastdrh/overview/引用:生物信息学简报,2022,23(5),bbac201 |
全新药物设计 | |
MCMG | 描述:基于条件变换器和强化学习的多约束分子生成方法链接:https://github.com/jkwang93/MCMG/引文:《自然·机器智能》,2021, 3(10), 914-922 |
化学家GA | 描述:用于现实世界药物发现的化学合成可访问分子生成算法链接:https://github.com/jkwang93/ChemistGA/引文:药物化学杂志, 2022, 65(18), 12482-12496 |
关系 | 描述:基于结构的从头药物设计的深度生成模型链接:https://github.com/micahwang/RELATION/引用:药物化学杂志, 2022, 65(13), 9478-9492 |
蛋白-蛋白对接 | |
鹰坞 | 描述:用于蛋白质-蛋白质复合物结构预测和分析的网络服务器链接:http://cadd.zju.edu.cn/hawkdock/引文:核酸研究,2019, 47(W1), W322-W330 |
分子图片识别 | |
ABC网 | 描述:用于从分子图像识别微笑的深度学习架构链接:https://github.com/zhang-xuan1314/ABC-Net/引用:生物信息学简报,2022, 23(2), bbac033 |
米赛尔 | 描述:用于分子图像描述的预训练编码器-解码器架构链接:https://github.com/Jiacai-Yi/MICER/引文:生物信息学,2022, 38(19), 4562-4572 |
药物设计工具 | |
基普 | 描述:一个网络服务器,可同时预测小分子对 204 种蛋白激酶的抑制活性。链接:http://cadd.zju.edu.cn/kip/引用文献:药学学报B , 2023, 13(1), 54-67 |
远期PPI | 描述: 通过 MM/PB(GB)SA 方法准确预测小分子 PPI 抑制剂的蛋白质-配体结合结构的网络服务器链接:http://cadd.zju.edu.cn/farppi/引文:生物信息学,2019, 35(10), 1777-1779 |
化学TB | 描述:一个网络服务器,通过人工智能识别抗结核分枝杆菌的活性分子链接:http://cadd.zju.edu.cn/chemtb/引用:生物信息学简报,2021, 22(5), bbab068 |
深度充电预测器 | 描述:一个网络服务器,用于通过最先进的机器学习算法预测基于 QM 的原子电荷链接:http://cadd.zju.edu.cn/deepchargepredictor/引文:生物信息学,2021, 37(22), 4255-4257 |
PROTAC-型号 | 描述: PROTAC 介导的三元复合物的综合建模链接:https://github.com/gaoqiweng/PROTAC-Model/引文:药物化学杂志, 2021, 64(21), 16271-16281 |
引文版本:
数据库 | |
---|---|
PROTAC-DB | Description: The first web-accessible PROTACs databaseLink: http://cadd.zju.edu.cn/protacdb/Citation: Nucleic Acids Research, 2021, 49(D1), D1381-D1387; Nucleic Acids Research, 2023, 51(D1), D1367-D1372 |
CovalentInDB | Description: The largest web-accessible resource for covalent inhibitors and related targetsLink: http://cadd.zju.edu.cn/cidb/Citation: Nucleic acids research, 49(D1), D1122–D1129 |
DDInter | Description: A comprehensive, professional, and open-access database specific to drug-drug interactionsLink: http://ddinter.scbdd.com/Citation: Nucleic acids research, 2022, 50(D1): D1200-D1207 |
VGSC | Description: The largest professional online database of voltage-gated sodium channelsLink: http://cadd.zju.edu.cn/vgsc/Citation: J Cheminformatics, 2022, 14, 75 |
PKKB | Description: The most extensive freely available database for collecting ADME (Absorption, Distribution, Metabolism, and Excretion) and Toxic propertiesLink: http://cadd.zju.edu.cn/pkkb/Citation: Journal of Chemical Information and Modeling, 2012, 52(5), 1132-1137 |
分子表征 | |
BioMedR | Description: An R/CRAN package for integrated data analysis pipeline in biomedical studyLink: https://cran.r-project.org/web/packages/BioMedR/Citation: Briefings in bioinformatics, 2021, 22(1): 474-484 |
Scopy | Description: An integrated negative design Python library for desirable HTS/VS database designLink: https://github.com/kotori-y/Scopy/Citation: Briefings in Bioinformatics, 2021, 22(3): bbaa194 |
PySmash | Description: Python package and individual executable program for representative substructure generation and applicationLink: https://github.com/kotori-y/pySmash/Citation: Briefings in Bioinformatics, 2021, 22(5): bbab017 |
QSAR建模 | |
MGA | Description: Mining Toxicity Information from Large Amounts of Toxicity DataLink: https://github.com/wzxxxx/MGA/Citation: Journal of Medicinal Chemistry, 2021, 64(10), 6924-6936 |
BAN | Description: Learning to SMILES: BAN-based strategies to improve latent representation learning from moleculesLink: https://github.com/zhang-xuan1314/SMILES-BiLSTM-attention-network/Citation: Briefings in Bioinformatics, 2021, 22(6), bbab327 |
MG-BERT | Description: Leveraging unsupervised atomic representation learning for molecular property predictionLink: https://github.com/zhang-xuan1314/Molecular-graph-BERT/Citation: Briefings in Bioinformatics, 2021, 22(6), bbab152 |
HRGCN+ | Description: Hyperbolic relational graph convolution networks plus: a simple but highly efficient QSAR-modeling methodLink: https://quantum.tencent.com/hrgcn/Citation: Briefings in Bioinformatics, 2021, 22(5), bbab112 |
成药性预测 | |
ADMETlab 2.0 | Description: An enhanced version of the widely used ADMETlab for systematical evaluation of ADMET properties, as well as some physicochemical properties and medicinal chemistry friendlinessLink: https://admetmesh.scbdd.com/Citation: Nucleic Acids Research, 2021, 49, W5-W14 |
ChemAGG | Description: A free web server that could be used to easily filter out aggregators from potential lead moleculesLink: https://admet.scbdd.com/ChemAGG/index/Citation: Journal of Chemical Information and Modeling, 2019, 59(9), 3714-3726 |
ChemFluo | Description: A public web server which could be useful to flag blue and green fluorescent compounds in large datasetLink: https://admet.scbdd.com/chemfluo/index/Citation: Briefings in Bioinformatics, 2021, 22(4), bbaa282 |
GASA | Description: A free web server for Graph Attention-based assessment of Synthetic Accessibility (GASA)Link: http://cadd.zju.edu.cn/gasa/Citation: Journal of Chemical Information and Modeling, 2022, 62(12), 2973-2986 |
Drug-logS | Description: A program freely distributed to the public for the estimation of aqueous solubilityLink: http://modem.ucsd.edu/adme/Citation: Journal of chemical information and computer sciences, 2004, 44(1), 266-275 |
蛋白-配体打分 | |
MetalProGNet | Description: A structure-based deep graph model for metalloprotein-ligand interaction predictionsLink: https://github.com/zjujdj/MetalProGNet/Citation: Chemical Science, 2023, 14(8), 2054-2069 |
RTMScore | Description: A protein-ligand scoring function based on residue-atom distance likelihood potential and graph transformerLink: https://github.com/sc8668/RTMScore/Citation: Journal of Medicinal Chemistry, 2022, 65(15), 10691-10706 |
IGN | Description: A deep graph representation learning framework for protein−ligand interaction predictionsLink: https://github.com/zjujdj/InteractionGraphNet/tree/master/Citation: Journal of Medicinal Chemistry, 2021, 64(24), 18209-18232 |
ASFP | Description: A web server for the development of customized scoring functionsLink: http://cadd.zju.edu.cn/plic/Citation: Journal of Cheminformatics, 2021, 13(1), 6 |
结合自由能预测 | |
CaFE | Description: A VMD plugin for binding affinity prediction using end-point free energy methodsLink: https://github.com/HuiLiuCode/CaFE_Plugin/Citation: Bioinformatics, 2016, 32(14), 2216-2218 |
fastDRH | Description: A web server to predict and analyze protein-ligand complexes based on molecular docking and MM/PB(GB)SA computationLink: http://cadd.zju.edu.cn/fastdrh/overview/Citation: Briefings in Bioinformatics, 2022, 23(5), bbac201 |
全新药物设计 | |
MCMG | Description: A multi-constraints molecular generation approach based on conditional transformer and reinforcement learningLink: https://github.com/jkwang93/MCMG/Citation: Nature Machine Intelligence, 2021, 3(10), 914-922 |
ChemistGA | Description: A Chemical Synthesizable Accessible Molecular Generation Algorithm for Real-World Drug DiscoveryLink: https://github.com/jkwang93/ChemistGA/Citation: Journal of Medicinal Chemistry, 2022, 65(18), 12482-12496 |
RELATION | Description: A Deep Generative Model for Structure-based De Novo Drug DesignLink: https://github.com/micahwang/RELATION/Citation: Journal of Medicinal Chemistry, 2022, 65(13), 9478-9492 |
蛋白-蛋白对接 | |
HawkDock | Description: A web server for the structural prediction and analysis of protein-protein complexLink: http://cadd.zju.edu.cn/hawkdock/Citation: Nucleic Acids Research, 2019, 47(W1), W322-W330 |
分子图片识别 | |
ABC-Net | Description: A deep learning architecture for SMILES recognition from molecular imagesLink: https://github.com/zhang-xuan1314/ABC-Net/Citation: Briefings in Bioinformatics, 2022, 23(2), bbac033 |
MICER | Description: A pre-trained encoder-decoder architecture for molecular image captioningLink: https://github.com/Jiacai-Yi/MICER/Citation: Bioinformatics, 2022, 38(19), 4562-4572 |
药物设计工具 | |
KIP | Description: A web server to predict the inhibitory activity of small molecules against 204 protein kinases simultaneously.Link: http://cadd.zju.edu.cn/kip/Citation: Acta Pharmaceutica Sinica B, 2023, 13(1), 54-67 |
farPPI | Description: A web server for accurate prediction of protein-ligand binding structures for small-molecule PPI inhibitors by MM/PB(GB)SA methodsLink: http://cadd.zju.edu.cn/farppi/Citation: Bioinformatics, 2019, 35(10), 1777-1779 |
ChemTB | Description: A web server to identify active molecules against mycobacterium tuberculosis through artificial intelligenceLink: http://cadd.zju.edu.cn/chemtb/Citation: Briefings in Bioinformatics, 2021, 22(5), bbab068 |
DeepChargPredictor | Description: A web server for predicting QM-based atomic charges via state-of-the-art machine-learning algorithmsLink: http://cadd.zju.edu.cn/deepchargepredictor/Citation: Bioinformatics, 2021, 37(22), 4255-4257 |
PROTAC-Model | Description: Integrative modeling of PROTAC-mediated ternary complexesLink: https://github.com/gaoqiweng/PROTAC-Model/Citation: Journal of Medicinal Chemistry, 2021, 64(21), 16271-16281 |
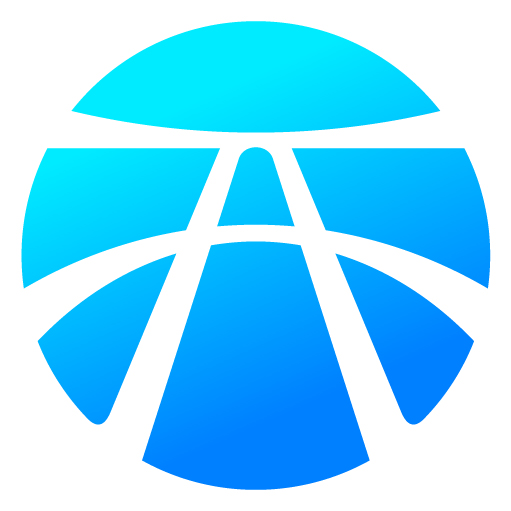
开放原子开发者工作坊旨在鼓励更多人参与开源活动,与志同道合的开发者们相互交流开发经验、分享开发心得、获取前沿技术趋势。工作坊有多种形式的开发者活动,如meetup、训练营等,主打技术交流,干货满满,真诚地邀请各位开发者共同参与!
更多推荐
所有评论(0)