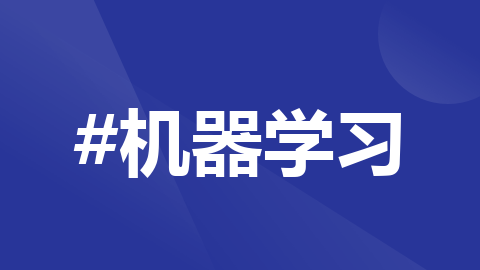
运行torchsummary报错
torchsummary报错,建议使用print(model)。
如果你想使用torchsummary打印模型结构,但是报错,那么其实笔者便已经不推荐使用torchsummary
了,你可以尝试测试代码的第一种方法,他的方法更加通用,而且不依赖别的库。
测试代码的两种方式
模仿dataset.py, train.py加print(model)测试网络结构
但是这里你需要注意的是print(model)打印的只是继承自nn.Module的子类并且打印的是__init__
方法中全部的继承自nn.Module的对象。
from torch.utils.data import DataLoader
from torch.utils.data.dataset import Dataset
#from torchsummary import summary
class TrainSet(Dataset):
def __init__(self, X, Y):
# 定义好 image 的路径
self.X, self.Y = X, Y
def __getitem__(self, index):
return self.X[index], self.Y[index]
def __len__(self):
return len(self.X)
def main():
X_tensor = torch.ones((4,1,32, 256, 256))
Y_tensor = torch.zeros((4,1,32, 256, 256))
mydataset = TrainSet(X_tensor, Y_tensor)
train_loader = DataLoader(mydataset, batch_size=2, shuffle=True)
net=Net()
print(net)
import torch.nn as nn
loss_fn = nn.MSELoss()
optimizer = torch.optim.SGD(net.parameters(), lr=1e-3)
# 3) Training loop
for epoch in range(10):
for i, (X, y) in enumerate(train_loader):
# predict = forward pass with our model
pred = net(X)
loss = loss_fn(pred, y)
optimizer.zero_grad()
loss.backward()
optimizer.step()
print('epoch={},i={}'.format(epoch,i))
if __name__ == '__main__':
main()
使用torchsummary
def torch_summary():
from torchsummary import summary
import os, sys
os.environ["CUDA_VISIBLE_DEVICES"] = '3'
device = torch.device("cuda" if torch.cuda.is_available() else "cpu")
model = Net()
model = model.to(device=device)
input_tensor: tuple = (1, 32, 256, 256)
summary(model, input_size=input_tensor)
if __name__ == '__main__':
torch_summary()
torchsummary报错
torchsummary报错,建议使用print(model)。以下是集中在运行torchsummary时候常见错误:
未将model放入GPU上
报错内如如下:“RuntimeError: Input type (torch.cuda.FloatTensor) and weight type (torch.FloatTensor) should be the same”
由于input_size会自动将Tensor放入GPU中,所以当报这个错误时,那是你没有将生成的model放入GPU,需要添加如下代码:
device = torch.device("cuda" if torch.cuda.is_available() else "cpu")
model = model.to(device=device)
summary(model, input_size=(3, 224, 224))
GPU指定错误
报错内容如下:“RuntimeError: Expected all tensors to be on the same device, but found at least two devices, cuda:0 and cuda:5! (when checking argument for argument weight in method wrapper__cudnn_convolution)”
或者 “RuntimeError: CUDA error: out of memory
CUDA kernel errors might be asynchronously reported at some other API call,so the stacktrace below might be incorrect.”
或者 “RuntimeError: CUDA error: invalid device ordinal
CUDA kernel errors might be asynchronously reported at some other API call,so the stacktrace below might be incorrect.
For debugging consider passing CUDA_LAUNCH_BLOCKING=1.”
或者 “RuntimeError: CUDA error: out of memory
CUDA kernel errors might be asynchronously reported at some other API call,so the stacktrace below might be incorrect.
For debugging consider passing CUDA_LAUNCH_BLOCKING=1.”
实验室服务器上有多台GPU设备,训练时候会指定cuda:0, cuda:1。报这个错误是因为input_size会自动将参数传入cuda:0,而你有可能将mode传入了cuda:5,输入Tensor和model在两张不同的显卡上!!这个时候你需要将物理上的cuda:5设置为你环境变量中的cuda:0便可以解决问题,代码如下:
from torchsummary import summary
import os, sys
os.environ["CUDA_VISIBLE_DEVICES"] = '5'
device = torch.device("cuda" if torch.cuda.is_available() else "cpu")
model = model.to(device=device)
summary(model, input_size=(3, 224, 224))
需要注意的事项
input_size并不需要传入batch_size参数,除了batch_size之外的channel,slice(dimension),height,width都需要传入。而你在train时则需要传入batch_size参数,下面两句话是等价的,虽然他俩Tensor.shape的维度不同。
input = torch.randn(1, 3, 224, 224)
summary(model, input_size=(3, 224, 224))
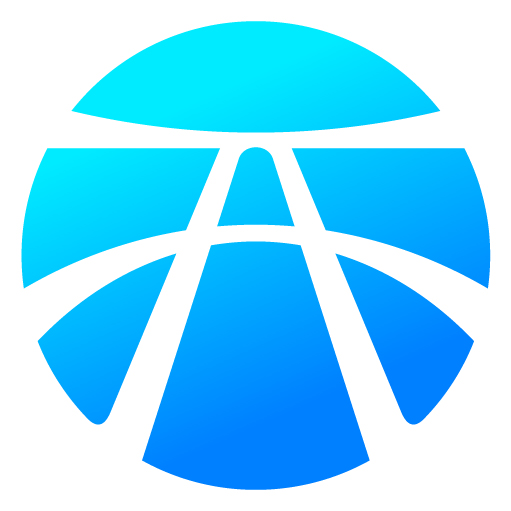
开放原子开发者工作坊旨在鼓励更多人参与开源活动,与志同道合的开发者们相互交流开发经验、分享开发心得、获取前沿技术趋势。工作坊有多种形式的开发者活动,如meetup、训练营等,主打技术交流,干货满满,真诚地邀请各位开发者共同参与!
更多推荐
所有评论(0)