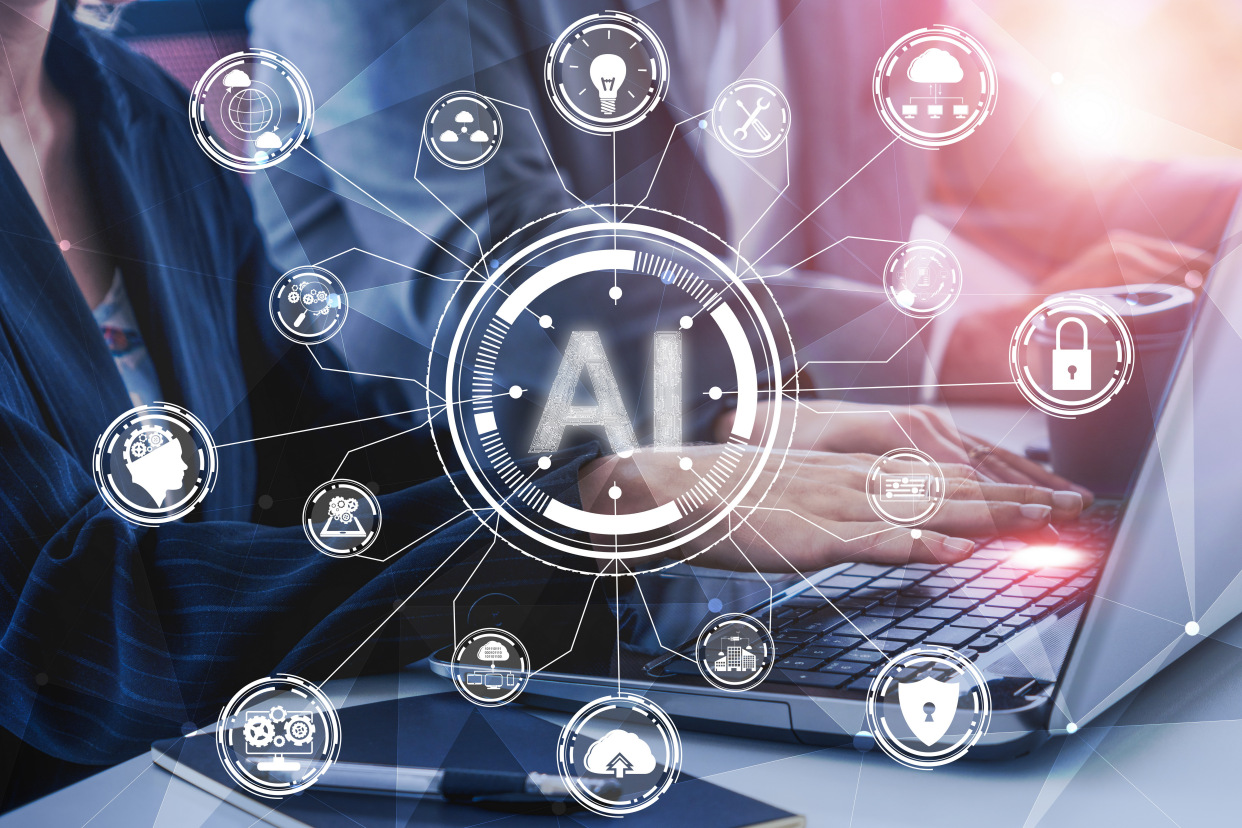
KNN算法实际应用案例:鸢尾花种类预测
1. scikit-learn数据集API介绍中获取数据的操作是在一个大类下的。1)sklearn小数据集使用获取小数据集,数据包含在datasets里,从本地获取,也就是活sklearn中有一小部分已经下好的数据集;例:使用获取鸢尾花数据集。返回值是鸢尾花数据集。# 1. 数据集获取# 1.1 获取小数据集用datasets.load_*()print(iris) # 会显示一堆的内容,但是阅读
KNN算法实际应用案例:鸢尾花种类预测
0. 案例:鸢尾花种类预测
Iris数据集是常用的分类实验数据集,由Fisher,1936年收集整理。Iris也称鸢尾花卉数据集,是一类多重变量分析的数据集。
关于数据集的具体介绍:
- 特征值-4个:花瓣、花萼的长度、宽度
- 目标值-3个:setosa、vericolor、virginica
该虹膜数据集包含150行数据,包括来自美国的三个相关鸢尾花种类50个样本:山鸢尾,虹膜锦葵,变色鸢尾。
注意:
在机器学习的相关细分领域,数据的收集工作一般是由行业专家来完成的。因为只有这些专家才知道哪些特征重要,哪些特征不重要。
1. 数据集获取和属性介绍
1.1 scikit-learn中的数据集介绍
1. scikit-learn数据集API介绍
scikit-learn
中获取数据的操作是在一个大类sklearn.datasets
下的。
1)sklearn小数据集
- 使用
sklearn.datasets.load_*()
获取小数据集,数据包含在datasets
里,从本地获取,也就是活sklearn
中有一小部分已经下好的数据集; - 例:使用
sklearn.datasets.load_iris()
获取鸢尾花数据集。sklearn.datasets.load_iris()
返回值是鸢尾花数据集。
from sklearn.datasets import load_iris
# 1. 数据集获取
# 1.1 获取小数据集用datasets.load_*()
iris = load_iris()
print(iris) # 会显示一堆的内容,但是阅读起来不方便
2)sklearn大数据集
- 使用
datasets.fetch_*(data_home=None)
获取大规模数据集,需要从网上下载,函数的第一个参数data_home
,表示数据集下载的目录,默认是~/scikit_learn_data/
; - 例:使用
sklearn.datasets.fetch_20newsgroups(data_home=None, subset='train')
获取20类新闻数据。参数subject
可传’train’、‘test’、或’all’,是可选的,表示要加载的数据集类型(测试集、训练集、全集)。
from sklearn.datasets import fetch_20newsgroups
# 1.2 获取大数据集用datasets.fetch_*(data_home=None)
news = fetch_20newsgroups()
print(news)
1.2 sklearn数据集返回值介绍
load
和fetch
返回的数据类型是datasets.base.Bunch
(字典格式):
data
:特征数据数组,是二维numpy.ndarray
数组;target
:标签数据,是一位numpy.ndarray
数组;DESCR
:数据描述信息;feature_names
:特证名;target_names
:标签名。
from sklearn.datasets import load_iris
# 1. 数据集获取
iris = load_iris()
# 2. 数据集属性描述
print('数据集的特征值:\n', iris.data)
print('数据集的目标值:\n', iris['target'])
print('数据集的特征值名字:\n', iris.feature_names)
print('数据集的目标值名字:\n', iris.target_names)
print('数据集的描述:\n', iris.DESCR)
输出:
数据集的特征值:
[[5.1 3.5 1.4 0.2]
[4.9 3. 1.4 0.2]
[4.7 3.2 1.3 0.2]
[4.6 3.1 1.5 0.2]
[5. 3.6 1.4 0.2]
[5.4 3.9 1.7 0.4]
[4.6 3.4 1.4 0.3]
[5. 3.4 1.5 0.2]
[4.4 2.9 1.4 0.2]
[4.9 3.1 1.5 0.1]
[5.4 3.7 1.5 0.2]
[4.8 3.4 1.6 0.2]
[4.8 3. 1.4 0.1]
[4.3 3. 1.1 0.1]
[5.8 4. 1.2 0.2]
[5.7 4.4 1.5 0.4]
[5.4 3.9 1.3 0.4]
[5.1 3.5 1.4 0.3]
[5.7 3.8 1.7 0.3]
[5.1 3.8 1.5 0.3]
[5.4 3.4 1.7 0.2]
[5.1 3.7 1.5 0.4]
[4.6 3.6 1. 0.2]
[5.1 3.3 1.7 0.5]
[4.8 3.4 1.9 0.2]
[5. 3. 1.6 0.2]
[5. 3.4 1.6 0.4]
[5.2 3.5 1.5 0.2]
[5.2 3.4 1.4 0.2]
[4.7 3.2 1.6 0.2]
[4.8 3.1 1.6 0.2]
[5.4 3.4 1.5 0.4]
[5.2 4.1 1.5 0.1]
[5.5 4.2 1.4 0.2]
[4.9 3.1 1.5 0.2]
[5. 3.2 1.2 0.2]
[5.5 3.5 1.3 0.2]
[4.9 3.6 1.4 0.1]
[4.4 3. 1.3 0.2]
[5.1 3.4 1.5 0.2]
[5. 3.5 1.3 0.3]
[4.5 2.3 1.3 0.3]
[4.4 3.2 1.3 0.2]
[5. 3.5 1.6 0.6]
[5.1 3.8 1.9 0.4]
[4.8 3. 1.4 0.3]
[5.1 3.8 1.6 0.2]
[4.6 3.2 1.4 0.2]
[5.3 3.7 1.5 0.2]
[5. 3.3 1.4 0.2]
[7. 3.2 4.7 1.4]
[6.4 3.2 4.5 1.5]
[6.9 3.1 4.9 1.5]
[5.5 2.3 4. 1.3]
[6.5 2.8 4.6 1.5]
[5.7 2.8 4.5 1.3]
[6.3 3.3 4.7 1.6]
[4.9 2.4 3.3 1. ]
[6.6 2.9 4.6 1.3]
[5.2 2.7 3.9 1.4]
[5. 2. 3.5 1. ]
[5.9 3. 4.2 1.5]
[6. 2.2 4. 1. ]
[6.1 2.9 4.7 1.4]
[5.6 2.9 3.6 1.3]
[6.7 3.1 4.4 1.4]
[5.6 3. 4.5 1.5]
[5.8 2.7 4.1 1. ]
[6.2 2.2 4.5 1.5]
[5.6 2.5 3.9 1.1]
[5.9 3.2 4.8 1.8]
[6.1 2.8 4. 1.3]
[6.3 2.5 4.9 1.5]
[6.1 2.8 4.7 1.2]
[6.4 2.9 4.3 1.3]
[6.6 3. 4.4 1.4]
[6.8 2.8 4.8 1.4]
[6.7 3. 5. 1.7]
[6. 2.9 4.5 1.5]
[5.7 2.6 3.5 1. ]
[5.5 2.4 3.8 1.1]
[5.5 2.4 3.7 1. ]
[5.8 2.7 3.9 1.2]
[6. 2.7 5.1 1.6]
[5.4 3. 4.5 1.5]
[6. 3.4 4.5 1.6]
[6.7 3.1 4.7 1.5]
[6.3 2.3 4.4 1.3]
[5.6 3. 4.1 1.3]
[5.5 2.5 4. 1.3]
[5.5 2.6 4.4 1.2]
[6.1 3. 4.6 1.4]
[5.8 2.6 4. 1.2]
[5. 2.3 3.3 1. ]
[5.6 2.7 4.2 1.3]
[5.7 3. 4.2 1.2]
[5.7 2.9 4.2 1.3]
[6.2 2.9 4.3 1.3]
[5.1 2.5 3. 1.1]
[5.7 2.8 4.1 1.3]
[6.3 3.3 6. 2.5]
[5.8 2.7 5.1 1.9]
[7.1 3. 5.9 2.1]
[6.3 2.9 5.6 1.8]
[6.5 3. 5.8 2.2]
[7.6 3. 6.6 2.1]
[4.9 2.5 4.5 1.7]
[7.3 2.9 6.3 1.8]
[6.7 2.5 5.8 1.8]
[7.2 3.6 6.1 2.5]
[6.5 3.2 5.1 2. ]
[6.4 2.7 5.3 1.9]
[6.8 3. 5.5 2.1]
[5.7 2.5 5. 2. ]
[5.8 2.8 5.1 2.4]
[6.4 3.2 5.3 2.3]
[6.5 3. 5.5 1.8]
[7.7 3.8 6.7 2.2]
[7.7 2.6 6.9 2.3]
[6. 2.2 5. 1.5]
[6.9 3.2 5.7 2.3]
[5.6 2.8 4.9 2. ]
[7.7 2.8 6.7 2. ]
[6.3 2.7 4.9 1.8]
[6.7 3.3 5.7 2.1]
[7.2 3.2 6. 1.8]
[6.2 2.8 4.8 1.8]
[6.1 3. 4.9 1.8]
[6.4 2.8 5.6 2.1]
[7.2 3. 5.8 1.6]
[7.4 2.8 6.1 1.9]
[7.9 3.8 6.4 2. ]
[6.4 2.8 5.6 2.2]
[6.3 2.8 5.1 1.5]
[6.1 2.6 5.6 1.4]
[7.7 3. 6.1 2.3]
[6.3 3.4 5.6 2.4]
[6.4 3.1 5.5 1.8]
[6. 3. 4.8 1.8]
[6.9 3.1 5.4 2.1]
[6.7 3.1 5.6 2.4]
[6.9 3.1 5.1 2.3]
[5.8 2.7 5.1 1.9]
[6.8 3.2 5.9 2.3]
[6.7 3.3 5.7 2.5]
[6.7 3. 5.2 2.3]
[6.3 2.5 5. 1.9]
[6.5 3. 5.2 2. ]
[6.2 3.4 5.4 2.3]
[5.9 3. 5.1 1.8]]
数据集的目标值:
[0 0 0 0 0 0 0 0 0 0 0 0 0 0 0 0 0 0 0 0 0 0 0 0 0 0 0 0 0 0 0 0 0 0 0 0 0
0 0 0 0 0 0 0 0 0 0 0 0 0 1 1 1 1 1 1 1 1 1 1 1 1 1 1 1 1 1 1 1 1 1 1 1 1
1 1 1 1 1 1 1 1 1 1 1 1 1 1 1 1 1 1 1 1 1 1 1 1 1 1 2 2 2 2 2 2 2 2 2 2 2
2 2 2 2 2 2 2 2 2 2 2 2 2 2 2 2 2 2 2 2 2 2 2 2 2 2 2 2 2 2 2 2 2 2 2 2 2
2 2]
数据集的特征值名字:
['sepal length (cm)', 'sepal width (cm)', 'petal length (cm)', 'petal width (cm)']
数据集的目标值名字:
['setosa' 'versicolor' 'virginica']
数据集的描述:
.. _iris_dataset:
Iris plants dataset
--------------------
**Data Set Characteristics:**
:Number of Instances: 150 (50 in each of three classes)
:Number of Attributes: 4 numeric, predictive attributes and the class
:Attribute Information:
- sepal length in cm
- sepal width in cm
- petal length in cm
- petal width in cm
- class:
- Iris-Setosa
- Iris-Versicolour
- Iris-Virginica
:Summary Statistics:
============== ==== ==== ======= ===== ====================
Min Max Mean SD Class Correlation
============== ==== ==== ======= ===== ====================
sepal length: 4.3 7.9 5.84 0.83 0.7826
sepal width: 2.0 4.4 3.05 0.43 -0.4194
petal length: 1.0 6.9 3.76 1.76 0.9490 (high!)
petal width: 0.1 2.5 1.20 0.76 0.9565 (high!)
============== ==== ==== ======= ===== ====================
:Missing Attribute Values: None
:Class Distribution: 33.3% for each of 3 classes.
:Creator: R.A. Fisher
:Donor: Michael Marshall (MARSHALL%PLU@io.arc.nasa.gov)
:Date: July, 1988
The famous Iris database, first used by Sir R.A. Fisher. The dataset is taken
from Fisher's paper. Note that it's the same as in R, but not as in the UCI
Machine Learning Repository, which has two wrong data points.
This is perhaps the best known database to be found in the
pattern recognition literature. Fisher's paper is a classic in the field and
is referenced frequently to this day. (See Duda & Hart, for example.) The
data set contains 3 classes of 50 instances each, where each class refers to a
type of iris plant. One class is linearly separable from the other 2; the
latter are NOT linearly separable from each other.
.. topic:: References
- Fisher, R.A. "The use of multiple measurements in taxonomic problems"
Annual Eugenics, 7, Part II, 179-188 (1936); also in "Contributions to
Mathematical Statistics" (John Wiley, NY, 1950).
- Duda, R.O., & Hart, P.E. (1973) Pattern Classification and Scene Analysis.
(Q327.D83) John Wiley & Sons. ISBN 0-471-22361-1. See page 218.
- Dasarathy, B.V. (1980) "Nosing Around the Neighborhood: A New System
Structure and Classification Rule for Recognition in Partially Exposed
Environments". IEEE Transactions on Pattern Analysis and Machine
Intelligence, Vol. PAMI-2, No. 1, 67-71.
- Gates, G.W. (1972) "The Reduced Nearest Neighbor Rule". IEEE Transactions
on Information Theory, May 1972, 431-433.
- See also: 1988 MLC Proceedings, 54-64. Cheeseman et al"s AUTOCLASS II
conceptual clustering system finds 3 classes in the data.
- Many, many more ...
2. 数据可视化介绍(查看数据分布)
通过创建一些图,来查看不同类别是如何通过特征来区分的。在理想情况下,标签类将由一个或多个特征对完美分割,在现实世界中,这种情况很少发生。
seaborn介绍:
seaborn
基于Matplotlib
核心库进行了更高级的API封装,可以让你轻松地画出更漂亮的图形。
seaborn的常用API介绍:
seaborn.lmplot()
是一个非常有用的方法,它会在绘制二维散点图时,自动完成回归拟合。sns.lmplot()
里的x,y分别表示横纵坐标的列名;data
:数据集,是DataFrame
类型;hue
:代表按照species
即花的类别分类显示;fit_reg
:表示是否进行线性拟合。
import seaborn as sns
import matplotlib.pyplot as plt
import pandas as pd
from sklearn.datasets import load_iris
# 1. 数据集获取
iris = load_iris()
# 2. 数据集属性描述
# ...
# 3. 数据可视化
# 把数据转换成dataframe的格式
iris_d = pd.DataFrame(iris.data, columns=['Sepal_length', 'Sepal_width', 'Petal_length', 'Petal_width'])
iris_d['Species'] = iris.target # 增加一列目标值
def plot_iris(iris_d, col1, col2):
sns.lmplot(x=col1, y=col2, data=iris_d, hue='Species', fit_reg=False)
plt.xlabel(col1)
plt.ylabel(col2)
plt.title('鸢尾花种类分布图')
plt.show()
plot_iris(iris_d, 'Petal_width', 'Sepal_length') # 这两个特征也可以换
3. 数据集的划分
1. 机器学习一般的数据集会划分为两个部分:
- 训练数据:用于训练,构建模型;
- 测试数据:在模型检验时使用,用于评估模型是否有效。
2. 划分比例:
- 训练集:70%,80%,75%;
- 测试集:30%,20%,25%。
3. 数据集划分api:sklearn.model_selection.train_test_split(arrays, *options)
1)参数:
x
:数据集的特征值;y
:数据集的标签值;test_size
:测试集占全集的百分比,一般穿float
类型数据;random_state
:随机数种子,不同的种子会造成不同的随机取样结果;相同的种子采样结果相同。
2)返回值:
x_train
,x_test
,y_train
,y_test
:一共四个返回值,一定要按顺序接收。分别是训练集特征值,测试集特征值,训练集目标值,测试集目标值。
from sklearn.datasets import load_iris, fetch_20newsgroups
from sklearn.model_selection import train_test_split
from pylab import mpl
# 设置显示中文字体
mpl.rcParams["font.sans-serif"] = ["SimHei"]
# 1. 数据集获取
iris = load_iris()
# 2. 数据集属性描述
# ...
# 3. 数据可视化
# ...
# 4. 数据集的划分
x_train, x_test, y_train, y_test = train_test_split(iris.data, iris.target, test_size=0.2, random_state=22)
print('训练集的特征值:\n', x_train)
print('训练集的目标值:\n', y_train)
print('测试集的特征值:\n', x_test)
print('测试集的目标值:\n', y_test)
# 可以发现,随机数种子一样,测试集的目标值一样,即测试结果一样
x_train1, x_test1, y_train1, y_test1 = train_test_split(iris.data, iris.target, test_size=0.2, random_state=2)
x_train2, x_test2, y_train2, y_test2 = train_test_split(iris.data, iris.target, test_size=0.2, random_state=2)
print('测试集的目标值:\n', y_test1)
print('测试集的目标值:\n', y_test2)
输出:
训练集的特征值:
[[4.8 3.1 1.6 0.2]
[5.4 3.4 1.5 0.4]
[5.5 2.5 4. 1.3]
[5.5 2.6 4.4 1.2]
[5.7 2.8 4.5 1.3]
[5. 3.4 1.6 0.4]
[5.1 3.4 1.5 0.2]
[4.9 3.6 1.4 0.1]
[6.9 3.1 5.4 2.1]
[6.7 2.5 5.8 1.8]
[7. 3.2 4.7 1.4]
[6.3 3.3 4.7 1.6]
[5.4 3.9 1.3 0.4]
[4.4 3.2 1.3 0.2]
[6.7 3. 5. 1.7]
[5.6 3. 4.1 1.3]
[5.7 2.5 5. 2. ]
[6.5 3. 5.8 2.2]
[5. 3.6 1.4 0.2]
[6.1 2.8 4. 1.3]
[6. 3.4 4.5 1.6]
[6.7 3. 5.2 2.3]
[5.7 4.4 1.5 0.4]
[5.4 3.4 1.7 0.2]
[5. 3.5 1.3 0.3]
[4.8 3. 1.4 0.1]
[5.5 4.2 1.4 0.2]
[4.6 3.6 1. 0.2]
[7.2 3.2 6. 1.8]
[5.1 2.5 3. 1.1]
[6.4 3.2 4.5 1.5]
[7.3 2.9 6.3 1.8]
[4.5 2.3 1.3 0.3]
[5. 3. 1.6 0.2]
[5.7 3.8 1.7 0.3]
[5. 3.3 1.4 0.2]
[6.2 2.2 4.5 1.5]
[5.1 3.5 1.4 0.2]
[6.4 2.9 4.3 1.3]
[4.9 2.4 3.3 1. ]
[6.3 2.5 4.9 1.5]
[6.1 2.8 4.7 1.2]
[5.9 3.2 4.8 1.8]
[5.4 3.9 1.7 0.4]
[6. 2.2 4. 1. ]
[6.4 2.8 5.6 2.1]
[4.8 3.4 1.9 0.2]
[6.4 3.1 5.5 1.8]
[5.9 3. 4.2 1.5]
[6.5 3. 5.5 1.8]
[6. 2.9 4.5 1.5]
[5.5 2.4 3.8 1.1]
[6.2 2.9 4.3 1.3]
[5.2 4.1 1.5 0.1]
[5.2 3.4 1.4 0.2]
[7.7 2.6 6.9 2.3]
[5.7 2.6 3.5 1. ]
[4.6 3.4 1.4 0.3]
[5.8 2.7 4.1 1. ]
[5.8 2.7 3.9 1.2]
[6.2 3.4 5.4 2.3]
[5.9 3. 5.1 1.8]
[4.6 3.1 1.5 0.2]
[5.8 2.8 5.1 2.4]
[5.1 3.5 1.4 0.3]
[6.8 3.2 5.9 2.3]
[4.9 3.1 1.5 0.1]
[5.5 2.3 4. 1.3]
[5.1 3.7 1.5 0.4]
[5.8 2.7 5.1 1.9]
[6.7 3.1 4.4 1.4]
[6.8 3. 5.5 2.1]
[5.2 2.7 3.9 1.4]
[6.7 3.1 5.6 2.4]
[5.3 3.7 1.5 0.2]
[5. 2. 3.5 1. ]
[6.6 2.9 4.6 1.3]
[6. 2.7 5.1 1.6]
[6.3 2.3 4.4 1.3]
[7.7 3. 6.1 2.3]
[4.9 3. 1.4 0.2]
[4.6 3.2 1.4 0.2]
[6.3 2.7 4.9 1.8]
[6.6 3. 4.4 1.4]
[6.9 3.1 4.9 1.5]
[4.3 3. 1.1 0.1]
[5.6 2.7 4.2 1.3]
[4.8 3.4 1.6 0.2]
[7.6 3. 6.6 2.1]
[7.7 2.8 6.7 2. ]
[4.9 2.5 4.5 1.7]
[6.5 3.2 5.1 2. ]
[5.1 3.3 1.7 0.5]
[6.3 2.9 5.6 1.8]
[6.1 2.6 5.6 1.4]
[5. 3.4 1.5 0.2]
[6.1 3. 4.6 1.4]
[5.6 3. 4.5 1.5]
[5.1 3.8 1.5 0.3]
[5.6 2.8 4.9 2. ]
[4.4 3. 1.3 0.2]
[5.5 2.4 3.7 1. ]
[4.7 3.2 1.6 0.2]
[6.7 3.3 5.7 2.5]
[5.2 3.5 1.5 0.2]
[6.4 2.7 5.3 1.9]
[6.3 2.8 5.1 1.5]
[4.4 2.9 1.4 0.2]
[6.1 3. 4.9 1.8]
[4.9 3.1 1.5 0.2]
[5. 2.3 3.3 1. ]
[4.8 3. 1.4 0.3]
[5.8 4. 1.2 0.2]
[6.3 3.4 5.6 2.4]
[5.4 3. 4.5 1.5]
[7.1 3. 5.9 2.1]
[6.3 3.3 6. 2.5]
[5.1 3.8 1.9 0.4]
[6.4 2.8 5.6 2.2]
[7.7 3.8 6.7 2.2]]
训练集的目标值:
[0 0 1 1 1 0 0 0 2 2 1 1 0 0 1 1 2 2 0 1 1 2 0 0 0 0 0 0 2 1 1 2 0 0 0 0 1
0 1 1 1 1 1 0 1 2 0 2 1 2 1 1 1 0 0 2 1 0 1 1 2 2 0 2 0 2 0 1 0 2 1 2 1 2
0 1 1 1 1 2 0 0 2 1 1 0 1 0 2 2 2 2 0 2 2 0 1 1 0 2 0 1 0 2 0 2 2 0 2 0 1
0 0 2 1 2 2 0 2 2]
测试集的特征值:
[[5.4 3.7 1.5 0.2]
[6.4 3.2 5.3 2.3]
[6.5 2.8 4.6 1.5]
[6.3 2.5 5. 1.9]
[6.1 2.9 4.7 1.4]
[6.8 2.8 4.8 1.4]
[6.7 3.1 4.7 1.5]
[6. 3. 4.8 1.8]
[5.6 2.9 3.6 1.3]
[5. 3.2 1.2 0.2]
[6.9 3.2 5.7 2.3]
[5.7 3. 4.2 1.2]
[7.4 2.8 6.1 1.9]
[7.2 3.6 6.1 2.5]
[5. 3.5 1.6 0.6]
[7.9 3.8 6.4 2. ]
[5.6 2.5 3.9 1.1]
[5.7 2.8 4.1 1.3]
[6. 2.2 5. 1.5]
[5.7 2.9 4.2 1.3]
[5.1 3.8 1.6 0.2]
[6.9 3.1 5.1 2.3]
[5.5 3.5 1.3 0.2]
[5.8 2.6 4. 1.2]
[5.8 2.7 5.1 1.9]
[4.7 3.2 1.3 0.2]
[7.2 3. 5.8 1.6]
[6.5 3. 5.2 2. ]
[6.7 3.3 5.7 2.1]
[6.2 2.8 4.8 1.8]]
测试集的目标值:
[0 2 1 2 1 1 1 2 1 0 2 1 2 2 0 2 1 1 2 1 0 2 0 1 2 0 2 2 2 2]
测试集的目标值:
[0 0 2 0 0 2 0 2 2 0 0 0 0 0 1 1 0 1 2 1 1 1 2 1 1 0 0 2 0 2]
测试集的目标值:
[0 0 2 0 0 2 0 2 2 0 0 0 0 0 1 1 0 1 2 1 1 1 2 1 1 0 0 2 0 2]
4. 特征工程
4.1 归一化
1. 定义:
通过对原始数据进行变换把数据映射到一个区间(默认为[0, 1])之间。
2. 公式:
X ′ = x − m i n m a x − m i n X'=\dfrac{x-min}{max-min} X′=max−minx−min X ′ ′ = X ′ ∗ ( m x − m i ) + m i X''=X'*(mx-mi)+mi X′′=X′∗(mx−mi)+mi
该公式作用于每一列, m a x max max为一列的最大值, m i n min min为一列的最小值, X ′ ′ X'' X′′为最终结果, m x mx mx, m i mi mi分别为指定区间值默认 m x mx mx为1, m i mi mi为0。
3. API:
sklearn.preprocessing.MinMaxScaler(feature_range=(0,1)...)
feature_range
:作用是指定区间,即 m x mx mx和 m i mi mi;
MinMaxScaler.fit_transform(X)
- X:要处理的数据;
- 返回值:转换后的
array
。
4. 数据计算:
我们将使用以下数据进行演示:
data = pd.DataFrame(np.random.randint(200, 4000, size=(5, 4)))
print(data)
print(data.shape)
'''
0 1 2 3
0 1042 2383 3304 1735
1 1671 583 3095 1299
2 585 202 1782 310
3 1598 2106 3108 1896
4 1844 3765 2852 516
(5, 4)
'''
先实例化MinMaxScaler
,再通过fit_transform()
处理数据:
import numpy as np
import pandas as pd
from sklearn.preprocessing import MinMaxScaler
data = pd.DataFrame(np.random.randint(200, 4000, size=(5, 4)))
print(data)
print(data.shape)
transfer = MinMaxScaler(feature_range=(2, 3)) # 实例化转换器
ret = transfer.fit_transform(data[[0, 1, 2]]) # 只处理前三列,目标值不用处理
print("最小值最大值归一化处理结果:\n", ret)
print(ret.shape)
输出:
0 1 2 3
0 1042 2383 3304 1735
1 1671 583 3095 1299
2 585 202 1782 310
3 1598 2106 3108 1896
4 1844 3765 2852 516
(5, 4)
最小值最大值归一化处理结果:
[[2.3629865 2.61212461 3. ]
[2.86258936 2.10693236 2.86268068]
[2. 2. 2. ]
[2.80460683 2.53438114 2.87122208]
[3. 3. 2.70302234]]
(5, 3)
5. 问题:如果数据中有异常值怎么办?
最小值和最大值非常容易受到异常点的影响,所以这归一化方法的鲁棒性(稳定性)较差,只适用于传统精确小数据场景。
可以通过标准化解决。
4.2 标准化
1. 定义:
通过对原始数据进行变换把数据变换到均值为0,标准差为1的范围内。
2. 公式:
X ′ = x − m e a n σ X'=\dfrac{x-mean}{\sigma} X′=σx−mean
作用于每一列, m e a n mean mean为平均值, σ \sigma σ为标准差。
- 对于归一化:如果出现异常点,影响了最大值和最小值,那么结果显然会发生改变;
- 对于标准化:如果出现异常点,由于具有一定数据量,少量的异常点对于平均值的影响并不大,从而方差改变较小。
3. API:
sklearn.preprocessing.StandardScaler()
- 处理之后,每一列的所有数据都聚集在均值0附近,标准差为1;
StandardScaler.fit_transform(X)
X
:要处理的数据;- 返回值:转换后的形状相同的
array
。
4. 数据计算:
准备数据:
import pandas as pd
import numpy as np
data = pd.DataFrame(np.random.randint(200, 4000, size=(5, 4)))
print(data)
'''
0 1 2 3
0 3682 1293 1565 2305
1 900 2344 2176 3036
2 884 2296 2413 292
3 1987 486 1279 1088
4 2206 2416 1155 990
'''
先实例化StandardScaler
,再用fit_transform()
处理数据:
import pandas as pd
import numpy as np
from sklearn.preprocessing import StandardScaler
data = pd.DataFrame(np.random.randint(200, 4000, size=(5, 4)))
print(data)
# 1. 实例化一个转换器嘞
transfer = StandardScaler()
# 2. 调用fit_transform
ret = transfer.fit_transform(data[[0, 1, 2]])
print('标准化的结果:\n', ret)
print('每一列特征的平均值:\n', transfer.mean_)
print('每一列特征的方差:\n', transfer.var_)
输出:
0 1 2 3
0 3682 1293 1565 2305
1 900 2344 2176 3036
2 884 2296 2413 292
3 1987 486 1279 1088
4 2206 2416 1155 990
标准化的结果:
[[ 1.6993148 -0.62243456 -0.30818698]
[-1.00180151 0.75768933 0.92577267]
[-1.01733633 0.69465798 1.40441168]
[ 0.05359512 -1.6821491 -0.88578511]
[ 0.26622793 0.85223635 -1.13621227]]
每一列特征的平均值:
[1931.8 1767. 1717.6]
每一列特征的方差:
[1060785.76 579921.6 245177.44]
5. 鸢尾花种类预测
1. 再识K-近邻算法API:sklearn.neighbors.KNeighborsClassifier(n_neighbors=5, algorithm=‘auto’)
n_neighbors
:int
类型数据,可选,默认为5,表示查询的邻居数;algorithm
:{‘auto’, ‘ball_tree’, ‘kd_tree’, ‘brute’}auto
:默认参数为auto
,表示自动选择算法;brute
:蛮力搜索,也就是线性扫描,当训练集很大时,计算非常耗时;kd_tree
:构造kd树存储数据,以便对其进行快速检索。在维数小于20时效率高;ball_tree
:是为了客服kd树高维失效而发明的,其构造过程是以质心C和半径r分割样本空间,每个节点是一个超球体。
2. 用K近邻算法实现鸢尾花的种类预测:
from sklearn.datasets import load_iris
from sklearn.model_selection import train_test_split
from sklearn.preprocessing import StandardScaler
from sklearn.neighbors import KNeighborsClassifier
# 1. 获取数据
iris = load_iris()
# 2. 数据基本处理
x_train, x_test, y_train, y_test = train_test_split(iris.data, iris.target, test_size=0.2, random_state=22)
# 3. 特征工程 - 特征预处理
transfer = StandardScaler() # 标准化
x_train = transfer.fit_transform(x_train)
x_test = transfer.fit_transform(x_test)
# 4. 机器学习-KNN
# 4.1 实例化一个估计器
estimator = KNeighborsClassifier(n_neighbors=5)
# 4.2 模型训练
estimator.fit(x_train, y_train)
# 5. 模型评估
# 5.1 预测值结果输出
y_pre = estimator.predict(x_test) # 预测值
print('预测值是:\n', y_pre)
print('预测值和真实值的对比:\n', y_pre==y_test)
# 5.2 准确率计算
score = estimator.score(x_test, y_test)
print('准确率为:\n', score)
输出:
预测值是:
[0 2 1 1 1 1 1 1 1 0 2 1 2 2 0 2 1 1 1 1 0 2 0 1 1 0 1 1 2 1]
预测值和真实值的对比:
[ True True True False True True True False True True True True
True True True True True True False True True True True True
False True False False True False]
准确率为:
0.7666666666666667
6. 交叉验证和网格搜索
6.1 交叉验证
1. 什么是交叉验证:
将拿到的训练集数据,再细分为训练集和验证集。将数据分为S份,其中一份作为验证集,然后经过S组的测试,每次更换不同的验证集。会得到了S组泛化误差,取平均值为最终。又称S折交叉验证。
2. 交叉验证过程:
1)随机将训练数据等分成k份,S1,S2,…,Sk;
2)对于每一个模型Mi,算法执行k次,每次选择一个Sj作为验证集,而其他作为训练集来训练模型Mi,把训练得到的模型在Sj上进行测试,这样一来,每次都会得到一个误差E,最后对k次得到的误差求平均,就可以得到Mi的泛化误差;
3)算法选择具有最小泛化误差的模型作为最终模型,并且在整个训练集上再次训练该模型,从而得到最终的模型。
- 以4折交叉验证为例:
将训练集数据等分成4份,用不同的验证集做4次训练,得到4组准确率,求这4组准确率的平均数。用1减去这个平均数可以得到一个泛化误差。
3. 交叉验证的目的:
为了让被评估的模型更加准确可信。
注意,只是准确可信,不能优化模型。
6.2 网格搜索
1. 什么是网格搜索:
通常情况下,有很多参数是需要手动指定的(如K-近邻算法中的K值),这种参数叫超参数。但是手动指定过程繁杂,所以需要对模型预设几种参数组合。每组超参数都采用交叉验证来进行评估。最后选出最优参数组合,建立模型。
2. 交叉验证、网格搜索(模型选择与调优)API:
sklearn.model_selection.GridSearchCV(estimator, param_grid=None, cv=None)
1)参数:
estimator
:估计器对象;param_grid
:估计器参数,字典类型数据;cv
:指定几折交叉验证。
2)结果分析:
best_score_
:在交叉验证中得到的最好结果;best_estimator_
:最好的参数模型(有时打印时不显示参数,可用best_params_
代替);cv_results_
:每次交叉验证后的验证集准确率结果和训练集准确率结果。
6.3 鸢尾花实例
from sklearn.datasets import load_iris
from sklearn.model_selection import train_test_split, GridSearchCV
from sklearn.preprocessing import StandardScaler
from sklearn.neighbors import KNeighborsClassifier
# 1. 获取数据
iris = load_iris()
# 2. 数据基本处理
x_train, x_test, y_train, y_test = train_test_split(iris.data, iris.target, test_size=0.2, random_state=22)
# 3. 特征工程 - 特征预处理
transfer = StandardScaler() # 标准化
x_train = transfer.fit_transform(x_train)
x_test = transfer.fit_transform(x_test)
# 4. 机器学习-KNN
# 4.1 实例化一个估计器
estimator = KNeighborsClassifier(n_neighbors=5)
# 4.2 模型调优 -- 交叉验证,网格搜索
param_grid = {'n_neighbors': [1, 3, 5, 7]}
estimator = GridSearchCV(estimator, param_grid=param_grid, cv=5)
# 4.3 模型训练
estimator.fit(x_train, y_train)
# 5. 模型评估
# 5.1 预测值结果输出
y_pre = estimator.predict(x_test) # 预测值
print('预测值是:\n', y_pre)
print('预测值和真实值的对比:\n', y_pre==y_test)
# 5.2 准确率计算
score = estimator.score(x_test, y_test)
print('准确率为:\n', score)
# 5.3 查看交叉验证,网格搜索的一些属性
print('交叉验证中,得到的最好结果:\n', estimator.best_score_)
print('交叉验证中,得到的最好模型的参数:\n', estimator.best_params_)
print('交叉验证中,得到的模型结果是:\n', estimator.cv_results_)
输出:
预测值是:
[0 2 1 1 1 1 1 1 1 0 2 1 2 2 0 2 1 1 1 1 0 2 0 1 1 0 1 1 2 1]
预测值和真实值的对比:
[ True True True False True True True False True True True True
True True True True True True False True True True True True
False True False False True False]
准确率为:
0.7666666666666667
交叉验证中,得到的最好结果:
0.9583333333333333
交叉验证中,得到的最好模型的参数:
{'n_neighbors': 5}
交叉验证中,得到的模型结果是:
{'mean_fit_time': array([0.00078802, 0.00040035, 0.00019875, 0.00040088]), 'std_fit_time': array([0.00039485, 0.00049033, 0.00039749, 0.00049097]), 'mean_score_time': array([0.00207067, 0.00200114, 0.00280037, 0.00239539]), 'std_score_time': array([1.03795303e-04, 2.66602721e-06, 4.03164015e-04, 4.85739388e-04]), 'param_n_neighbors': masked_array(data=[1, 3, 5, 7],
mask=[False, False, False, False],
fill_value='?',
dtype=object), 'params': [{'n_neighbors': 1}, {'n_neighbors': 3}, {'n_neighbors': 5}, {'n_neighbors': 7}], 'split0_test_score': array([0.95833333, 0.95833333, 1. , 1. ]), 'split1_test_score': array([0.95833333, 0.91666667, 0.91666667, 0.91666667]), 'split2_test_score': array([0.95833333, 0.95833333, 1. , 1. ]), 'split3_test_score': array([0.875 , 0.875 , 0.91666667, 0.91666667]), 'split4_test_score': array([0.95833333, 0.95833333, 0.95833333, 0.95833333]), 'mean_test_score': array([0.94166667, 0.93333333, 0.95833333, 0.95833333]), 'std_test_score': array([0.03333333, 0.03333333, 0.0372678 , 0.0372678 ]), 'rank_test_score': array([3, 4, 1, 1])}
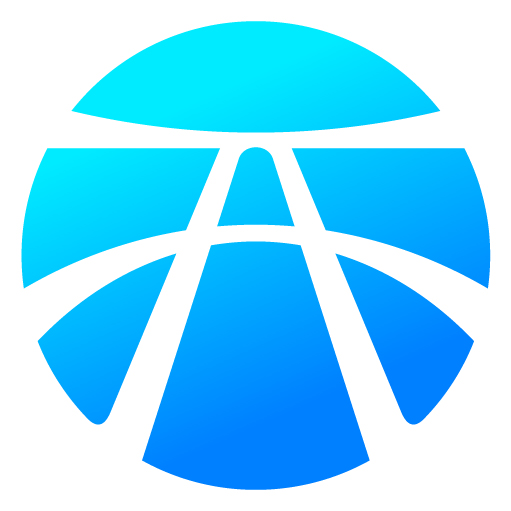
开放原子开发者工作坊旨在鼓励更多人参与开源活动,与志同道合的开发者们相互交流开发经验、分享开发心得、获取前沿技术趋势。工作坊有多种形式的开发者活动,如meetup、训练营等,主打技术交流,干货满满,真诚地邀请各位开发者共同参与!
更多推荐
所有评论(0)