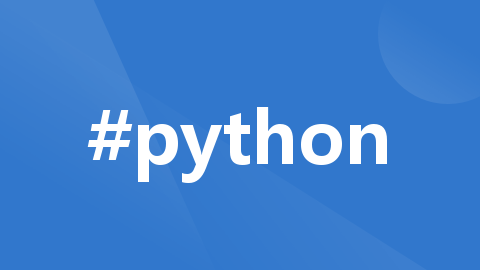
python之进程池、进程间通讯之用例、避坑(持续更新)
(a)需要引入以下模块(b)建议使用以下的方式,将会在执行完任务后,主动释放进程池但需要注意的是self.poolNum是创建进程数量。windows系统中最小数量是1,最大数量是61,除此之外会报错。pass(c)参数说明:工作进程数量。mp_context:进程上下文。如果None,则会以默认的进程上下文执行。:函数,启动进程前会执行这个函数。initargs:元组格式的参数,传参给的函数。(
·
目录
前言
☆ 广告时间
一、创建进程池的方式
(1)ProcessPoolExecutor
- (a)需要引入以下模块
from concurrent.futures import ProcessPoolExecutor
- (b)建议使用以下的方式,将会在执行完任务后,主动释放进程池
- 但需要注意的是self.poolNum是创建进程数量。windows系统中最小数量是1,最大数量是61,除此之外会报错。
with ProcessPoolExecutor(max_workers=self.poolNum) as executor:
pass
- (c)参数说明
max_workers
:工作进程数量。mp_context
:进程上下文。如果None,则会以默认的进程上下文执行。initializer
:函数,启动进程前会执行这个函数。initargs
:元组格式的参数,传参给initializer
的函数。
- (d)并发函数
submit
:异步提交任务。返回Future对象。Future.add_done_callback
:指进程进行异步非阻塞式执行,参数fn
只有一个参数Future
,当任务完成或者任务被中断时,就会回调这个fn
函数,并且成功时result
结果为None。
from concurrent.futures import ProcessPoolExecutor, Future import time import os # 错误示范函数 def test_failure(i): print('index:%d pid:%s\n' % (str(i), os.getpid())) pass # 正确示范函数 def test_success(i): time.sleep(0.2) print('index:%d pid:%s\n' % (i, os.getpid())) time.sleep(0.5) pass # 结果 def test_result(future: Future): print('----------result:%s pid:%s\n' % (str(future.done()), os.getpid())) pass def test_pool(): with ProcessPoolExecutor(max_workers=4) as executor: for i in range(0, 10): fu:Future = executor.submit(test_success, (i)) fu.add_done_callback(test_result) pass pass if __name__ == '__main__': test_pool()
Future.done
:指进程进行阻塞式执行。
from concurrent.futures import ProcessPoolExecutor import time import os # 错误示范函数 def test_failure(i): print('index:%d pid:%s\n' % (str(i), os.getpid())) pass # 正确示范函数 def test_success(i): time.sleep(1) print('index:%d pid:%s\n' % (i, os.getpid())) pass def test_pool(): with ProcessPoolExecutor(4) as executor: l = [] for i in range(0, 10): a = executor.submit(test_success, i) l.append(a) while len(l) > 0: for a1 in l: if a1.done(): l.remove(a1) time.sleep(0.05) executor.shutdown() pass pass if __name__ == '__main__': test_pool()
map
:可迭代对象异步并发任务。
from concurrent.futures import ProcessPoolExecutor import time import os # 错误示范函数 def test_failure(i): print('index:%d pid:%s\n' % (str(i), os.getpid())) pass # 正确示范函数 def test_success(i): time.sleep(1) print('index:%d pid:%s\n' % (i, os.getpid())) pass def test_pool(): with ProcessPoolExecutor(4) as executor: l = [1, 23, 4, 5, 6] executor.map(test_success, l) executor.shutdown() pass pass if __name__ == '__main__': test_pool()
- (e)关闭进程池
shutdown
:这函数中有两个参数wait
,cancel_futures
:wait
:true的话,则不会再收到任何任务执行反馈。cancel_futures
:true的话,将所有待处理的任务将会被取消,而已完成或者正在完成的任务则不会。
(2)multiprocessing
- (a)需要引入以下模块
from multiprocessing import Pool
- (b)建议使用以下方式,将会在执行完成任务后,主动释放进程池
with Pool(self.poolNum) as pool:
pass
- (c)参数说明
processes
:工作进程数量。initializer
:函数,启动进程前会执行这个函数。initargs
:元组格式的参数,传参给initializer
的函数。maxtasksperchild
:工作进程退出之前可以完成的任务数,完成后用一个新的工作进程来替代原进程,来让闲置的资源被释放。maxtasksperchild默认是None,意味着只要Pool存在工作进程就会一直存活。context
:进程的上下文。
- (d)并发函数
apply
:并发阻塞式执行任务。
from multiprocessing import Pool import os def test(i): print('index:%d pid:%s' % (i, os.getpid())) pass def test_pool(): with Pool(4) as pool: for i in range(0, 10): pool.apply(func=test, args=(i, )) pool.close() pool.join() pass pass if __name__ == '__main__': test_pool()
apply_async
:并发非阻塞式执行任务。但是如果调用了get
函数,则会变成阻塞式执行。
from multiprocessing import Pool import os # 错误示范函数 def test_failure(i): print('index:%d pid:%s\n' % (str(i), os.getpid())) pass # 正确示范函数 def test_success(i): print('index:%d pid:%s\n' % (str(i), os.getpid())) pass # 正确结束回调 def callback(data): print('callback:%s\n' % data) pass # 错误回调 def error_callback(error): print('error:%s\n' % error) pass def test_pool(): with Pool(2) as pool: for i in range(0, 5): pool.apply_async( func=test_success, args=(i, ), callback=callback, error_callback=error_callback) # 进程池用完了之后,close用于释放,join则是为了等待全部任务完成 pool.close() pool.join() pass pass if __name__ == '__main__': test_pool()
map
:将可迭代的变量,逐个元素进行并发阻塞式执行。
from multiprocessing import Pool import os # 错误示范函数 def test_failure(i): print('index:%d pid:%s\n' % (str(i), os.getpid())) pass # 正确示范函数 def test_success(i): print('index:%d pid:%s\n' % (i, os.getpid())) pass def test_pool(): with Pool(2) as pool: pool.map(func=test_success, iterable=range(0, 5)) # 进程池用完了之后,close用于释放,join则是为了等待全部任务完成 pool.close() pool.join() pass pass if __name__ == '__main__': test_pool()
map_async
:将可迭代的变量,逐个元素进行并发非阻塞式执行。但是如果调用了get
函数,则会变成阻塞式执行。
from multiprocessing import Pool import os # 错误示范函数 def test_failure(i): print('index:%d pid:%s\n' % (str(i), os.getpid())) pass # 正确示范函数 def test_success(i): print('index:%d pid:%s\n' % (i, os.getpid())) pass # 正确结束回调 def callback(data): print('callback:%s\n' % data) pass # 错误回调 def error_callback(error): print('error:%s\n' % error) pass def test_pool(): with Pool(2) as pool: pool.map_async( func=test_success, iterable=range(0, 5), callback=callback, error_callback=error_callback) # 进程池用完了之后,close用于释放,join则是为了等待全部任务完成 pool.close() pool.join() pass pass if __name__ == '__main__': test_pool()
starmap
:与map
函数相似,但是将func
参数修改为多个传参。
from multiprocessing import Pool import os # 错误示范函数 def test_failure(i): print('index:%d pid:%s\n' % (str(i), os.getpid())) pass # 正确示范函数 def test_success(i, j): print('index:%d j:%d pid:%s\n' % (i, j, os.getpid())) pass def test_pool(): with Pool(2) as pool: l = [(0, 1), (1, 2)] pool.starmap( func=test_success, iterable=l) # 进程池用完了之后,close用于释放,join则是为了等待全部任务完成 pool.close() pool.join() pass pass if __name__ == '__main__': test_pool()
starmap_async
:与map_async
函数相似,但是将func
参数修改为多个传参。
- (e)启动进程
join
:这个函数是开始并等待前面的任务执行完。
- (f)关闭进程池
close
:函数指,不再接收新的任务,并等到全部的任务完成才会退出进程池。terminate
:函数是指,将所有待处理的任务取消,并等待正在处理中的任务完成才会退出进程池。
这两个退出的函数均需要加join
函数才能正常退出进程池
二、进程间通讯
(1)管道
- (a)参数说明
Pipe([duplex])
:duplex=True,全双工,即双端都是收发消息;反之则parentConn只能收,childConn只能发。 - (b)用法示例
from multiprocessing import Process, Pipe
import os
# 错误示范函数
def test_failure(i):
print('index:%d pid:%s\n' % (str(i), os.getpid()))
pass
# 正确示范函数
def test_success(i, conn):
print('index:%d pid:%s\n' % (i, os.getpid()))
conn.send('idx:%d child:pid:%s'%(i, os.getpid()))
pass
def test_pool():
parentConn, childConn = Pipe()
for i in range(0, 10):
p = Process(target=test_success, args=(i, childConn, ))
p.start()
print('parent pid:%s recv:%s' % (os.getpid(), parentConn.recv()))
p.join()
parentConn.close()
childConn.close()
pass
if __name__ == '__main__':
test_pool()
(2)共享变量
- (a)需要引入以下模块
from multiprocessing import Manager
Manager模块的Queue
、Value
、Array
才是进程安全的共享变量。
- (b)
Queue
- (c)
Value
- (d)
Array
- (e)
Lock
Value
和Array
是支持进程共享的变量,但是为了避免脏读脏写,需要使用Lock
。
三、注意事项
(1)执行任务,时间并没有缩短太多
- (a)创建进程池或者多进程,本身需要系统或者程序分配空间,这就会产生比较大的时间和空间开销,会导致任务执行周期过长;
- (b)执行任务时,存在共享数据或者资源的情况下,实际上需要进行排队指定的资源,这是任务的短板。
(2)执行任务,出现资源竞争,或者脏读脏写
- (a)多进程执行的过程中共享了数据,或者抢占了资源,如多进程同时对一个文件进行读写,或者对一个数据进行读写,就会产生资源竞争或者脏读脏写。
解决方案:multiprocessing.Manager.Queue
:共享变量。- 将会产生竞争的子任务单独划分给指定进程处理。
(3)执行任务,使用global标识符,不能真正共享
- (a)因为对于每个进程来说,global会产生一个属于该进程的全局变量,因此,global并不能用于多进程间共享。
(4)执行任务,函数没有执行,也没有报错
- (a)可以作为传参的变量必须是可序列化的,而对象是没办法序列化的,如果没有执行也没有报错,很大概率是这个报错了。
- (b)可以监听异步函数中的
error_callback
异步回调,这样会比较容易定位到问题。
(5)进程池执行任务时,阻塞了父进程的运行
- (a)增加子线程,用这个线程来管理进程池中的子进程,这样就不会父进程(本身就是一个主线程)就不用忙于管理进程池的任务分配,而无暇顾及其他了。
from concurrent.futures import ProcessPoolExecutor, Future
import threading
import time
import os
# 错误示范函数
def test_failure(i):
print('index:%d pid:%s\n' % (str(i), os.getpid()))
pass
# 正确示范函数
def test_success(i):
time.sleep(0.2)
print('index:%d pid:%s\n' % (i, os.getpid()))
time.sleep(0.5)
pass
# 结果
def test_result(future: Future):
print('----------result:%s pid:%s\n' % (str(future.done()), os.getpid()))
pass
def test_pool():
with ProcessPoolExecutor(max_workers=4) as executor:
for i in range(0, 10):
fu:Future = executor.submit(test_success, (i))
fu.add_done_callback(test_result)
pass
pass
def main():
thread = threading.Thread(target=test_pool)
thread.start()
timeCount = 0
while True:
timeCount += 1
time.sleep(0.5)
if timeCount >= 20:
break
print('timeCount:%d pid:%s\n' % (timeCount, os.getpid()))
if __name__ == '__main__':
main()
(6)打包exe后,运行异常
- (a)程序中有多进程或者进程池,需要引入以下函数
from multiprocessing import freeze_support
并在程序中写入freeze_support()
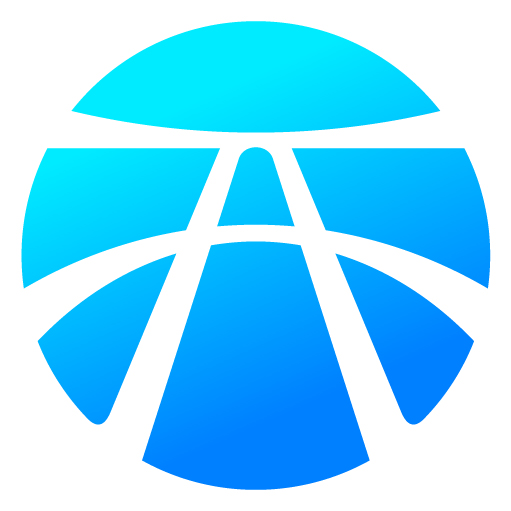
开放原子开发者工作坊旨在鼓励更多人参与开源活动,与志同道合的开发者们相互交流开发经验、分享开发心得、获取前沿技术趋势。工作坊有多种形式的开发者活动,如meetup、训练营等,主打技术交流,干货满满,真诚地邀请各位开发者共同参与!
更多推荐
所有评论(0)