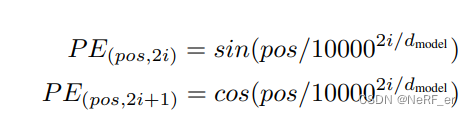
mvsplat 的笔记
因此,代码里有两个 for loop, 一个 循环 .torch 文件, 一个 循环 torch 文件里面的数据集。每一个 pixel 生成一个坐标, 对应一个 Gaussian. Pixel 发生光线,, 通过卷积 变成2个通道,其中一个作为 density, 另一个作为 视差。.并不一定是从 像素 中点 发生光心, 因此,每一个 pixel 还有一个。的4个通道预测的,先得到四元数。图像, 而
Pose 处理:
MVsplat 需要尽可能对于 Pose 进行 Normalize 得到的 效果比较好,就是 Translation 在 【-1,1】之间。
变量理解:
context_image
: 表示投影的 refrence image
Epipolar Transformer
vs Swin Transformer
: 不同于 Pixel Splat 使用的是 Epipolar Transformer. MVspalt 使用的是 Swin Transformer, 但是作者在 Code 里面 也使用了 Epipolar Transformer 并对此进行了 消融实验:
网络架构,得到 CNN_feature 和 Transformer_feature :
- 假设 context_imgae shape :
(1,2,3,256,256)
- 进入一个 Backbone Multiview 的Encoder; 这个是 一个 CNN 的 Encoder 去提取 Image 的 feature, 进行了 4 倍的 downsampling , 对应的 cnn_features shape
(1,2,128,64,64)
## CNN 提取特征
features_list = self.extract_feature(self.normalize_images(images)) # list of features
- 对这个 Feature 添加位置信息,使用Transformer 里面的 PE, 但是不会改变 Tensor 的大小。shape
(1,2,128,64,64)
- 将上面的 Tensor 送入到 Transformer 当中, 输出的 Tensor 维度不变, transformer 计算的 shape 依然是
(1,2,128,64,64)
经过Transformer 网络之后,预测 3D Gaussian feature 和 深度:
depths, densities, raw_gaussians = self.depth_predictor(
in_feats, ## transformer feature (1,2,128,64,64)
context["intrinsics"],
context["extrinsics"],
context["near"],
context["far"],
gaussians_per_pixel=True,
deterministic=deterministic,
extra_info=extra_info,
cnn_features=cnn_features, ## CNN feature (1,2,128,64,64)
)
变量 refine_out:(1,32,256,256)
image:(2,3,256,256)
pro_fea_in_fullers:(2,128,256,256)
1. 在这个函数中首先进行 数据预处理:
feat_comb_lists, intr_curr, pose_curr_lists, disp_candi_curr = (
prepare_feat_proj_data_lists(features,intrinsics,extrinsics,near,far,num_samples=self.num_depth_candidates)
)
主要的 功能如下:
* 对于 depth 进行等间距的 128 个采样点.
* feat_comb_lists 第0个元素是 [0,1] 排列的 transformer feature feature_01;
第1个元素是 [1,0] 排列的 transformer feature feature_10 ;
* 对于 re10k format 的内参 unnormalize
* pose_curr_lists 分别是 0->1 的位姿变换和 1->0 的位姿变换
2. 构建两个 Feature 的 Cost Volume:
feat10: 第一个元素是feature map 1; 第2个元素是feature map 2
pose_curr: 第一个元素是camera 1 -> camera 0 的 Transform ; 第2个元素是camera 0 -> camera 1 的 Transform
2.1 作用: 将feature map 1 根据深度 lift 成一个 3D Volume, 然后根据 Pose 将 3D 点投影到 image 0 的 2D 平面上 interpolate feature.
for feat10, pose_curr in zip(feat_comb_lists[1:], pose_curr_lists):
# 1. project feature1 to camera0 and project feture0 to camera 1
# feat10: [0] is feature map 1; [1] is feature map 0
feat01_warped = warp_with_pose_depth_candidates(
feat10,
intr_curr,
pose_curr,
1.0 / disp_candi_curr.repeat([1, 1, *feat10.shape[-2:]]),
warp_padding_mode="zeros",
) # [B, C, D, H, W] [2, 128, 128, 64, 64] 表示 128,64,64 个3D点 投影到2D平面上query 的feature. 每个feature 的 维度是 128维度
2.2 根据 不同的 depth 投影得到的 featuure 和原始的 feature 计算 点积 (相似度),然后对于 feature channel 那一个维度 求取 sum
raw_correlation_in = (feat01.unsqueeze(2) * feat01_warped).sum(1) / (c**0.5) # [vB, D, H, W]
2.3 使用2D U-Net 进行 CostVolume 的 Refine, 再使用Softmax 函数 估计 出每一个 采样 Depth的 权重。 (准确的Depth 权重应该最大)
pdf = F.softmax(
self.depth_head_lowres(raw_correlation), dim=1
) # [2xB, D, H, W]
2.4 SoftMax 得到的权重和 depth_candi 点积,可以得到 depth 的预测,然后上采样到原始分辨率
coarse_disps = (disp_candi_curr * pdf).sum(dim=1, keepdim=True) # (vb, 1, h, w)
fullres_disps = F.interpolate(
coarse_disps,
scale_factor=self.upscale_factor,
mode="bilinear",
align_corners=True,
)
coarse_disps :(2,1,64,64) 是feature map 的图像 的 Dpeth 预测
fullres_disps :(2,1,256,256) 是原始 Resolution 的图像 的 Dpeth 预测
2.5 对于预测的 Depth 使用 2DU-Net 进行Refine, 得到feature volume。
refine_out = self.refine_unet(torch.cat(
(extra_info["images"], proj_feature, fullres_disps, pdf_max), dim=1
))
最后的 refine depth 是 fullres_disps + delta_disps
fine_disps = (fullres_disps + delta_disps).clamp(
1.0 / rearrange(far, "b v -> (v b) () () ()"),
1.0 / rearrange(near, "b v -> (v b) () () ()"),
)
refine_out :(2,32,256,256) 是输入U-Net 得到的feature, 是32通道
2.6 利用 Transformer feature, CNN feature, Depth 的预测 和 原始的color 图像, 得到 Gaussian 的 feature map
这个 self. to_gaussians 是一个 两层的 CNN。 输入c=163, 输出 c=84
# gaussians head
raw_gaussians_in = [refine_out, extra_info["images"], proj_feat_in_fullres]
raw_gaussians_in = torch.cat(raw_gaussians_in, dim=1)
raw_gaussians = self.to_gaussians(raw_gaussians_in)
输出:raw_gaussians (2,84,256,256), 原始分辨率的 Gaussian feature map
下面是各种 Gaussian 属性的 预测 :
1. Opcaity 的 预测
对前面得到的 Costvolume 进行卷积。
输入是 refine_out:(1,32,256,256)
, 通过卷积 变成2个通道,其中一个作为 density, 另一个作为 视差。 文章的解释: matching volume 里面 对应关系越强,那么 density 越大
delta_disps_density = self.to_disparity(refine_out)
delta_disps, raw_densities = delta_disps_density.split(gaussians_per_pixel, dim=1)
# combine coarse and fine info and match shape
densities = repeat(
F.sigmoid(raw_densities),
"(v b) dpt h w -> b v (h w) srf dpt",
b=b,
v=v,
srf=1,
)
之后将 density 转成opacity, 转换通过一个构造函数进行的:
y
=
{
0
<
x
<
1
:
0.5
⋅
(
1
−
(
1
−
x
)
t
+
x
1
t
)
}
y = \left\{0<x<1: 0.5 \cdot\left(1-(1-x)^t+x^{\frac{1}{t}}\right)\right\}
y={0<x<1:0.5⋅(1−(1−x)t+xt1)}
2. Center 的 预测
每一个 pixel 生成一个坐标, 对应一个 Gaussian. Pixel 发生光线,根据 depth 反投影得到 Gaussian 的 Center.. 并不一定是从 像素 中点 发生光心, 因此,每一个 pixel 还有一个 2D 的offset 偏移量· offset_xy
,也是泛化得到的,从 raw_gaussians (2,84,256,256)
的前2个channel 生成。
offset_xy = gaussians[..., :2].sigmoid()
pixel_size = 1 / torch.tensor((w, h), dtype=torch.float32, device=device)
xy_ray = xy_ray + (offset_xy - 0.5) * pixel_size
means = origins + directions * depths[..., None]
3. Scale 的 预测
Scale 由 前3 个channel 确定,还需要和 depth 以及相机内参数有关系。 需要注意一下2点:
- Regarding multiplying by depths, further objects will be smaller when projected.
- Regarding multiplying by multiplier. This operation constrains the Gaussian scale concerning the pixel width in the image space, which
aims to ensure that the Gaussian scale with scale 1 is roughly the
same as 1 pixel in the image space.
scales = scale_min + (scale_max - scale_min) * scales.sigmoid()
h, w = image_shape
pixel_size = 1 / torch.tensor((w, h), dtype=torch.float32, device=device)
multiplier = self.get_scale_multiplier(intrinsics, pixel_size)
scales = scales * depths[..., None] * multiplier[..., None]
4. Covariance 的 预测
Rotations 是由 raw_gaussians
的4个通道预测的,先得到四元数。 之后再和 Scale 构成 协方差矩阵, 注意: 这里的 协方差矩阵是 camera 系下面的,还需要外参转到 world 坐标系:
rotations = rotations / (rotations.norm(dim=-1, keepdim=True) + eps)
covariances = build_covariance(scales, rotations)
c2w_rotations = extrinsics[..., :3, :3]
covariances = c2w_rotations @ covariances @ c2w_rotations.transpose(-1, -2)
4. SH 的 预测
剩下的 75个 channel 对应着 SH 系数
opacity 的生成 在 传入下面的函数之前已经生成了,是将 density 转换成 Gaussian 的 Opacity:
# 得到SH系数
sh = rearrange(sh, "... (xyz d_sh) -> ... xyz d_sh", xyz=3)
sh = sh.broadcast_to((*opacities.shape, 3, self.d_sh)) * self.sh_mask
根据上面的属性,得到 泛化的 Gaussian
return Gaussians(
means=means,
covariances=covariances,
harmonics=rotate_sh(sh, c2w_rotations[..., None, :, :]),
opacities=opacities,
# NOTE: These aren't yet rotated into world space, but they're only used for
# exporting Gaussians to ply files. This needs to be fixed...
scales=scales,
rotations=rotations.broadcast_to((*scales.shape[:-1], 4)),
)
生成当前场景的 3DGS 之后,在 Target View 上进行 Render
Pytorch Lighting 的基础知识:
Train 的主函数: training_step
函数:
Test 的主函数: test_step
函数:
Test 的 dataloader 的主函数:
val_dataloader
函数
test_dataloader
函数
数据Dataset 类 全部在 dataset_re10k.py 这个文件
def test_dataloader(self, dataset_cfg=None):
##主要用来 读取的数据文件都在 .torch
dataset = get_dataset(
self.dataset_cfg if dataset_cfg is None else dataset_cfg,
"test",
self.step_tracker,
)
dataset = self.dataset_shim(dataset, "test")
return DataLoader(
dataset,
self.data_loader_cfg.test.batch_size,
num_workers=self.data_loader_cfg.test.num_workers,
generator=self.get_generator(self.data_loader_cfg.test),
worker_init_fn=worker_init_fn,
persistent_workers=self.get_persistent(self.data_loader_cfg.test),
shuffle=False,
)
MVSplat 是加载 chunk 进行实验的:
每一个 chunk 是由 一个 xx.torch 文件加载过来的:
chunk = torch.load(chunk_path)
每一个 chunk 里面有 5个 dtu数据集, 每一个数据集里面存放着 45 张图像, 而每一个 数据集的以字典的形式进行存放。 如下所示,里面存放在 图像的 camera, image 和 数据集的名称 “key”. y 从 “camera” 读取随机一个场景的 内外参数:example 是 chunk 里面的某一个数据集:
extrinsics, intrinsics = self.convert_poses(example["cameras"])
之后 读取图像。
因此,代码里有两个 for loop, 一个 循环 .torch 文件, 一个 循环 torch 文件里面的数据集。
注意 3DGS 的 ply 文件是无法使用meshlab 打开查看的,必须有专门对应的 Viewer 才可以。
https://playcanvas.com/supersplat/editor
MVsplat 是如何在 KITTI-360 上运行的
-
将场景处理使用
convert_kitti360.py
处理成.torch
的文件, 每个场景生成一个torch
文件,注意 因为 最好对于 Pose 作为 归一化,才可能更好的 和 Re10K 的数据 尺度对齐; 但是如果生成的3DGS 和 Metric3D 估计的点云 重合,那就不应该归一化,使用KITTI-360提供的Pose。 -
虽然 Normalize Pose 在单场景上overfit 的更好,但是经过实验发现,在泛化的 时候,因为每个场景的 Normalize 的 Scale 并不一样,会导致在新的 Pose 上面出现 Render 的明显 shift. 因此在KITTI-360的泛化训练的时候,不要 对于 Pose 进行Normalize 并改变
near=0.01
和far=100.0
-
Mvsplat 使用 MVS 估计的 Depth 并不准确,为了得到更好的结果,建议的
near=0.01
和far=1.5
指定。 (Pose 必须 归一化之后)
运行代码:
python src/scripts/convert_kitti360.py --input_dir=/data/smiao/mvsplat_kitti/Train_10scene --output_dir=/data/smiao/mvsplat_kitti/
此外,需要修改 Stage , 生成的 文件路径保存在 line:135: Trainkitti
或者Testkitti
, 根据你生成的数据是用来训练还是用来 测试的。
- 将
Test
和Train
还有Val
三个数据集构建好. 构建的数据集TrainKitti
格式如下:├── TrainKitti ├── train ├── 00000.torch ├── 00001.torch ...... ├── test ├── val
一般来说,我们在训练的时候,只会用到 Train
还有 Val
两个数据集。
3.进入代码, Smiao_mvsplat. 修改 kitti360.yaml
里面的 roots
变量, 改成 [/data/smiao/mvsplat_kitti/Trainkitti/]
. 运行如下的命令:
python -m src.main +experiment=kitti360 data_loader.train.batch_size=1 output_dir=outputs/train_10scenes
训练好模型之后,需要在Pretrain 好的模型上进行 推理,一个场景一个场景进行推理。
假设我们有5个场景,全部生成为 .torch
的文件,放置在 test
的目录之下。 为了不和 Train
还有Val
的 dataloader
冲突, 我新添加了一个 dataset_Testkitti.py
程序文件,专门写如何在场景上进行不同 Droprate 的推理
执行前,需要先修改 data_set/__init__.py
的 cfg
文件
# DatasetCfg = DatasetRE10kCfg
# DatasetCfg = KITTI360Cfg ## Kitti360_Train
DatasetCfg = KITTI360Cfg_Test ## Kitti360_Test
实际执行的场景:
chunk_id = 1 ## 选择你需要 Inference Test 文件夹中的第几个场景。
chunk_path = self.chunks[chunk_id]
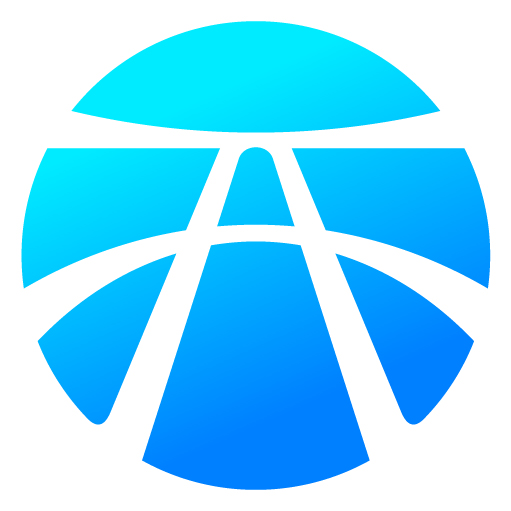
开放原子开发者工作坊旨在鼓励更多人参与开源活动,与志同道合的开发者们相互交流开发经验、分享开发心得、获取前沿技术趋势。工作坊有多种形式的开发者活动,如meetup、训练营等,主打技术交流,干货满满,真诚地邀请各位开发者共同参与!
更多推荐
所有评论(0)