机器学习之二分类模型评价指标
机器学习之二分类模型评价指标一、二分类模型衡量指标1.1 混淆矩阵:Confusion matrix1.2 精确度:Accuracy1.3 准确率:Precision1.4 召回率:Recall一、二分类模型衡量指标1.1 混淆矩阵:Confusion matrixP(Positive) :代表1N(Negative) :代表0T(True) :代表预测正确F(False) :代表预测错误1.2
·
机器学习之二分类模型评价指标
一、二分类模型衡量指标
1.1 混淆矩阵:Confusion matrix
1.1.1 原理
- P(Positive) :代表1
- N(Negative) :代表0
- T(True) :代表预测正确
- F(False) :代表预测错误
1.1.2 实现
from sklearn.metrics import confusion_matrix
confusion_matrix(y_true,y_pred,labels=None,sample_weight = None)
'''
y_true:真实的label,一维数组,列名
y_pred:预测值的label,一维数组,行名
labels:默认不指定,此时y_true,y_pred去并集,做升序,做label
sample_weight:样本权重
返回结果:返回混淆矩阵
'''
import numpy as np
from sklearn.metrics import confusion_matrix
y_true = np.array([0,1,1,1,0,0,1,0,0,1])
y_pred = np.array([0,0,1,1,1,0,1,1,0,1])
confusion_matrix = confusion_matrix(y_true,y_pred)
confusion_matrix
#结果
array([[3, 2],
[1, 4]], dtype=int64)
1.2 精确度:Accuracy
1.2.1 原理
- 预测正确的结果占总样本的比例。
P r e c i s i o n = T P + T N T P + F P + T N + F N Precision=\frac{TP+TN}{TP+FP+TN+FN} Precision=TP+FP+TN+FNTP+TN - 缺点:在样本不平衡时,不能很好的衡量结果。如正样本占90%,负样本占10%,样本是严重不平衡的。对于这种情况,我们只需要将全部样本预测为正样本即可得到90%的高准确率,但模型对负样本没有任何识别度。正因为如此,也就衍生了其他两种指标:准确率和召回率。
1.2.2 实现
from sklearn.metrics import accuracy_score
accuracy_score(y_true, y_pred, normalize=True, sample_weight=None)
'''
y_true:数据的真实label值
y_pred:数据的预测标签值
normalize:默认为True,返回正确预测的个数,若是为False,返回正确预测的比例
sample_weight:样本权重
返回结果:score为正确预测的个数或者比例,由normalize确定
'''
import numpy as np
import pandas as pd
from sklearn.metrics import accuracy_score
y_true = [1,1,0,1,0]
y_pred = [1,1,1,0,0]
score = accuracy_score(y_true,y_pred)
print(score)
#结果:0.6#正确预测的比例
score1 = accuracy_score(y_true,y_pred,normalize = True)
print(score1)
#结果:3#正确预测的个数
1.3 准确率:Precision
1.3.1 原理
- 又叫查准率,含义是所有被预测为正的样本中实际为正的样本的比例。
P r e c i s i o n = T P T P + F P Precision=\frac{TP}{TP+FP} Precision=TP+FPTP
1.3.2 实现
from sklearn.metrics import classification_report
classification_report(y_true, y_pred, labels=None,
target_names=None, sample_weight=None, digits=2, output_dict=False)
'''
y_true:真实的label,一维数组,列名
y_pred:预测值的label,一维数组,行名
labels:默认不指定,此时y_true,y_pred去并集,做升序,做label
sample_weight:样本权重
target_names:行标签,顺序和label的要一致
digits,整型,小数的位数
out_dict:输出格式,默认False,如果为True,输出字典。
'''
import numpy as np
from sklearn.metrics import classification_report
y_true = np.array([0,1,1,0,1,2,1])
y_pred = np.array([0,1,0,0,1,2,1])
target_names = ['class0','class1','class2']
print(classification_report(y_true,y_pred,target_names = target_names))
#结果如下
precision recall f1-score support
class0 0.67 1.00 0.80 2
class1 1.00 0.75 0.86 4
class2 1.00 1.00 1.00 1
micro avg 0.86 0.86 0.86 7
macro avg 0.89 0.92 0.89 7
weighted avg 0.90 0.86 0.86 7
1.4 召回率:Recall
1.4.1 原理
- 又叫查全率,含义是实际为正的样本中被预测为正样本的比例。
R e c a l l = T P T P + F N Recall=\frac{TP}{TP+FN} Recall=TP+FNTP - 召回率的应用场景:比如拿网贷违约率为例,相对好用户,我们更关心坏用户,不能错放过任何一个坏用户。因为如果我们过多的将坏用户当成好用户,这样后续可能发生的违约金额会远超过好用户偿还的借贷利息金额,造成严重偿失。召回率越高,代表实际坏用户被预测出来的概率越高,它的含义类似:宁可错杀一千,绝不放过一个。
1.4.2 实现
- 实现同1.3.2 。
1.5 F1-Score
1.5.1 原理
- 找寻Precision和Recall之间的平衡,是一个综合的评价指标。
F 1 − S c o r e = 2 1 P r e c i s i o n 1 R e c a l l = 2 ∗ P r e c i s i o n ∗ R e c a l l P r e c i s i o n + R e c a l l F1-Score=\frac{2}{\frac{1}{Precision}\frac{1}{Recall}}=\frac{2*Precision*Recall}{Precision+Recall} F1−Score=Precision1Recall12=Precision+Recall2∗Precision∗Recall
1.5.2 实现
- 实现同1.3.2 。
1.6 PR曲线
1.6.1 原理
- 当正负样本数差距不大时,ROC和PR曲线的趋势是差不多的,在正负样本分布不均匀的情况下,PRC比ROC能更有效地反应分类器的好坏。
- 横坐标是查全率Recall,纵坐标是查准率Precision。
- 当PR曲线越靠近右上方时,表明模型性能越好;
- 与ROC曲线类似,在对不同模型进行比较时,若一个模型的PR曲线被另一个模型的PR曲线完全包住则说明后者的性能优于前者.如上图中橘色线代表的模型要优于蓝色线代表的模型;
- 若模型的PR曲线发生了交叉,则无法直接判断哪个模型更好。在周志华老师的机器学习上中提到了可以用平衡点。它是查准率=查全率时的取值,如上图黑色线代表的模型的平衡点要大于橘色线模型代表的平衡点,表明前者优于后者,除此之外更为常用的是F1 score,也就是查准率和查全率的加权平均,F1 = (2查准率查全率)/(查准率+查全率)
- PR曲线绘制:设置一个从高到低的阈值,预测概率大于等于阈值的样本被预测为正类,小于阈值的样本被预测为负类。显然,设置n个阈值后,就可以得到n个混淆矩阵,从而得到n对Precision和Recall的值,便可绘制成PR曲线。
1.6.1 实现
from sklearn.metrics import precision_recall_curve
precision, recall, t =precision_recall_curve(y_test, y_score) #y_score是预测的概率值,y_test是真实值标签值
print(t) #t是阈值
plt.xlabel('Recall')
plt.ylabel('Precision')
plt.ylim([0.0, 1.0])
plt.xlim([0.0, 1.0])
plt.plot(recall, precision)
plt.title("Precision-Recall")
plt.show()
1.7 ROC曲线
1.7.1 原理
- TPR(True Positive Rate):真正例率,等于Recall。
T P R = T P T P + F N TPR=\frac{TP}{TP+FN} TPR=TP+FNTP - FPR(False Positive Rate):假正例率
F P R = F P F P + T N FPR=\frac{FP}{FP+TN} FPR=FP+TNFP - ROC:Receiver Operating Characteristic Curve,中文名字叫“受试者工作特征曲线”,ROC曲线以FPR为横坐标,TPR为纵坐标,样本数量越多,ROC曲线越平滑。
1.7.2 实现
from sklearn.metrics import roc_curve, auc
fpr,tpr,threshold = roc_curve(y_test, y_score) #y_score是预测概率,y_test是真实值类别
roc_auc = auc(fpr,tpr) ###计算auc的值
plt.figure()
lw = 2
plt.figure(figsize=(10,10))
plt.plot(fpr, tpr,
lw=lw, label='ROC curve (area = %0.2f)' % roc_auc) ###假正率为横坐标,真正率为纵坐标做曲线
plt.plot([0, 1], [0, 1], lw=lw, linestyle='--')
plt.xlim([0.0, 1.0])
plt.ylim([0.0, 1.05])
plt.xlabel('False Positive Rate')
plt.ylabel('True Positive Rate')
plt.title('ROC')
plt.legend(loc="best")
plt.show()
1.8 AUC值
1.8.1 原理
- AUC值是ROC曲线下方的面积,越接近于1,模型效果越好。
- 比较有意思的是,如果我们连接对角线,它的面积正好是0.5。对角线的实际含义是:随机判断正负样本,正负样本比率应该都是50%,表示随机效果。即最差的随机判断都有0.5,所以一般AUC的值是介于0.5到1之间的。
- UC的一般判断标准:
0.5 - 0.7:效果较低,但用于预测股票已经很不错了
0.7 - 0.85:效果一般
0.85 - 0.95:效果很好
0.95 - 1:效果非常好,但一般不太可能
1.8.2 实现
from sklearn.metrics import roc_curve, auc
fpr,tpr,threshold = roc_curve(y_test, y_score) #y_score是预测概率,y_test是真实值类别
roc_auc = auc(fpr,tpr) ###计算auc的值
print(roc_auc)
#结果
0.8133333333333334
1.9 KS值
1.9.1 原理
- KS值是风控领域常用语评估模型区分度的指标。
K S = m a x ( T P R − F P R ) KS=max(TPR-FPR) KS=max(TPR−FPR) - KS值用来评估模型的区分能力,KS值越大,模型的区分能力越强。
1.9.2 实现
- 实现1
from sklearn.metrics import roc_curve, auc
fpr,tpr,threshold = roc_curve(y_test, y_score) #y_score是预测概率,y_test是真实值类别
ks=max(tpr-fpr)
print(ks)
- 实现2
y_true,y_pred = y_train,pred_train # 先以train的结果为例
df = pd.DataFrame({"y":y_true,"pred":y_pred})
good_cumlis = []
bad_cumlis = []
good_total = np.sum(df['y']==0)
bad_total = np.sum(df['y']==1)
for i in cutlist:
tmpdf = df.loc[df['pred']<=i,:]
a = np.sum(tmpdf['y']==0)
b = np.sum(tmpdf['y']==1)
good_cumlis.append(a/good_total)
bad_cumlis.append(b/bad_total)
df2 = pd.DataFrame({"cutoff":cutlist,
"good_cum":good_cumlis,
"bad_cum":bad_cumlis,
"delta(good_cum,bad_cum)":[a-b for a,b in zip(good_cumlis,bad_cumlis)]})
plt.figure(figsize=(6,5))
deltalis = df2['delta(good_cum,bad_cum)'].tolist()
plt.plot(cutlist,df2['good_cum'], label = 'good_cum')
plt.plot(cutlist,df2['bad_cum'], label = 'bad_cum')
plt.plot(cutlist,deltalis,label = 'delta')
plt.legend(loc = 'best')
x1=cutlist[deltalis.index(max(deltalis))]
y1=max(deltalis)
plt.scatter(x1,y1,color='red')
plt.xlabel("cutoff")
plt.ylabel("delta(good_cum,bad_cum)")
plt.show()
print("Delta最大值坐标:\ncutoff=%.4f\ndelta(good_cum,bad_cum)=ks=%.4f"%(x1,y1))
#-----------------------OUTPUT-----------------------#
Delta最大值坐标:
cutoff=0.4174
delta(good_cum,bad_cum)=ks=0.5083
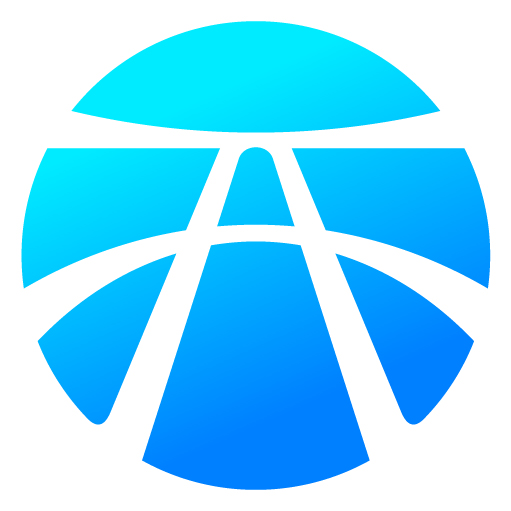
开放原子开发者工作坊旨在鼓励更多人参与开源活动,与志同道合的开发者们相互交流开发经验、分享开发心得、获取前沿技术趋势。工作坊有多种形式的开发者活动,如meetup、训练营等,主打技术交流,干货满满,真诚地邀请各位开发者共同参与!
更多推荐
所有评论(0)