LibTorch对tensor的索引/切片/掩码操作:对比PyTorch
目录一、通过索引获取值二、通过索引设置值在PyTorch C++ API(libtorch)中对张量进行索引的方式与Python API的方式很相似。诸如None / ... / integer / boolean / slice / tensor的索引类型在C++ API里同样有效,这样就可以很方便的实现Python代码与C++代码的转换。主要的不同是将Python API里对张量的“[ ]”操
目录
在PyTorch C++ API(libtorch)中对张量进行索引的方式与Python API的方式很相似。诸如None / ... / integer / boolean / slice / tensor的索引类型在C++ API里同样有效,这样就可以很方便的实现Python代码与C++代码的转换。主要的不同是将Python API里对张量的“[ ]”操作符转换成了以下形式:
torch::Tensor::index ( ) // 获取值
torch::Tensor::index_put_ ( ) // 设置值
有关官方文档请看这里。但是官方文档只简单地给了转换方式,没有具体例子有时还不太好理解。下面通过举例说明libtorch与pytorch中的向量索引/切片的方式,左边为Python方式,右边为C++方式:
一、通过索引获取值
1、tensor[Ellipsis, ...] --> tensor.index({Ellipsis, "..."})
import torch
a = torch.linspace(1,27,27).reshape(3, 3, 3)
print(a)
c = a[..., 2]
print(c)
#===================运行结果===============#
tensor([[[ 1., 2., 3.],
[ 4., 5., 6.],
[ 7., 8., 9.]],
[[10., 11., 12.],
[13., 14., 15.],
[16., 17., 18.]],
[[19., 20., 21.],
[22., 23., 24.],
[25., 26., 27.]]])
tensor([[ 3., 6., 9.],
[12., 15., 18.],
[21., 24., 27.]])
#include "iostream"
#include "torch/script.h"
int main()
{
torch::Tensor a = torch::linspace(1, 27, 27).reshape({3, 3, 3});
std::cout << a << std::endl;
at::Tensor b = a.index({"...", 2});
std::cout << b << std::endl;
return 0;
}
/****************输出结果******************/
(1,.,.) =
1 2 3
4 5 6
7 8 9
(2,.,.) =
10 11 12
13 14 15
16 17 18
(3,.,.) =
19 20 21
22 23 24
25 26 27
[ CPUFloatType{3,3,3} ]
3 6 9
12 15 18
21 24 27
[ CPUFloatType{3,3} ]
2、tensor[1, 2] --> tensor.index({1, 2})
import torch
a = torch.linspace(1,27,27).reshape(3, 3, 3)
print(a)
c = a[1, 2]
print(c)
#===================运行结果=================#
tensor([[[ 1., 2., 3.],
[ 4., 5., 6.],
[ 7., 8., 9.]],
[[10., 11., 12.],
[13., 14., 15.],
[16., 17., 18.]],
[[19., 20., 21.],
[22., 23., 24.],
[25., 26., 27.]]])
tensor([16., 17., 18.])
#include "iostream"
#include "torch/script.h"
int main()
{
torch::Tensor a = torch::linspace(1, 27, 27).reshape({3, 3, 3});
std::cout << a << std::endl;
at::Tensor b = a.index({1, 2});
std::cout << b << std::endl;
return 0;
}
/*****************运行结果***************/
(1,.,.) =
1 2 3
4 5 6
7 8 9
(2,.,.) =
10 11 12
13 14 15
16 17 18
(3,.,.) =
19 20 21
22 23 24
25 26 27
[ CPUFloatType{3,3,3} ]
16
17
18
[ CPUFloatType{3} ]
3、tensor[1::2] --> tensor.index({Slice(1, None, 2)})
import torch
a = torch.linspace(1, 6, 6)
print(a)
c = a[1::2]
print(c)
#==================运行结果==================#
tensor([1., 2., 3., 4., 5., 6.])
tensor([2., 4., 6.])
#include "iostream"
#include "torch/script.h"
using namespace torch::indexing;
int main()
{
torch::Tensor a = torch::linspace(1, 6, 6);
std::cout << a << std::endl;
at::Tensor b = a.index({Slice(1, None, 2)});
std::cout << b << std::endl;
return 0;
}
/*******************运行结果*********************/
1
2
3
4
5
6
[ CPUFloatType{6} ]
2
4
6
[ CPUFloatType{3} ]
3.33、tensor[..., 1:] --> tensor.index({"...", Slice(1)})
import torch
a = torch.linspace(1,27,27).reshape(3, 3, 3)
b = a[..., 1:]
print(b)
#===============运行结果===================#
tensor([[[ 2., 3.],
[ 5., 6.],
[ 8., 9.]],
[[11., 12.],
[14., 15.],
[17., 18.]],
[[20., 21.],
[23., 24.],
[26., 27.]]])
#include "iostream"
#include "torch/script.h"
using namespace torch::indexing;
int main()
{
torch::Tensor a = torch::linspace(1, 27, 27).reshape({3, 3, 3});
torch::Tensor b = a.index({"...", Slice(1)});
std::cout << b << std::endl;
return 0;
}
/******************运行结果**********************/
(1,.,.) =
2 3
5 6
8 9
(2,.,.) =
11 12
14 15
17 18
(3,.,.) =
20 21
23 24
26 27
[ CPUFloatType{3,3,2} ]
3.66、tensor[..., :2] --> tensor.index({"...", Slice({None, 2})})
#include "iostream"
#include "torch/script.h"
using namespace torch::indexing;
int main()
{
float a[2][3][3] = {{{1,2,2},{3,4,4},{5,6,6}},{{1,2,2},{3,4,4},{5,6,6}}};
at::Tensor b = at::from_blob(a, {2, 3, 3}, at::kFloat);
at::Tensor c = b.index({"...", Slice({None, 2})}); // 留下最后一维的前两列,相当于Python中的b[..., :2]
auto d = c.sizes();
std::cout << b << std::endl;
std::cout << c << std::endl;
std::cout << d << std::endl;
return 0;
}
/********************运行结果********************/
(1,.,.) =
1 2 2
3 4 4
5 6 6
(2,.,.) =
1 2 2
3 4 4
5 6 6
[ CPUFloatType{2,3,3} ]
(1,.,.) =
1 2
3 4
5 6
(2,.,.) =
1 2
3 4
5 6
[ CPUFloatType{2,3,2} ]
[2, 3, 2]
4、tensor[torch.tensor([1, 2])] --> tensor.index({torch::tensor({1, 2})})
import torch
a = torch.linspace(1,4,4)
b = torch.tensor([0, 1, 3, 2])
c = a[b]
print(a)
print(c)
#===============运行结果===============#
tensor([1., 2., 3., 4.])
tensor([1., 2., 4., 3.])
#include "iostream"
#include "torch/script.h"
using namespace torch::indexing;
int main()
{
torch::Tensor a = torch::linspace(1, 4, 4);
torch::Tensor b = torch::tensor({0, 1, 3, 2});
torch::Tensor c = a.index({b});
std::cout << a << std::endl;
std::cout << b << std::endl;
return 0;
}
/*******************运行结果********************/
1
2
3
4
[ CPUFloatType{4} ]
0
1
3
2
[ CPULongType{4} ]
二、通过索引设置值
1、tensor[1, 2] = 1 --> tensor.index_put_({1, 2}, 1)
import torch
a = torch.linspace(1,4,4).reshape(2, 2)
print(a)
a[1, 1] = 100
print(a)
#==================运行结果=====================#
tensor([[1., 2.],
[3., 4.]])
tensor([[ 1., 2.],
[ 3., 100.]])
#include "iostream"
#include "torch/script.h"
using namespace torch::indexing;
int main()
{
torch::Tensor a = torch::linspace(1, 4, 4).reshape({2, 2});
std::cout << a << std::endl;
a.index_put_({1, 1}, 100);
std::cout << a << std::endl;
return 0;
}
/***************运行结果****************/
1 2
3 4
[ CPUFloatType{2,2} ]
1 2
3 100
[ CPUFloatType{2,2} ]
2、tensor[Ellipsis, ...] = 1 --> tensor.index_put_({Ellipsis, "..."}, 1)
import torch
a = torch.linspace(1,4,4).reshape(2, 2)
print(a)
a[..., 1] = 100
print(a)
#====================运行结果=====================#
tensor([[1., 2.],
[3., 4.]])
tensor([[ 1., 100.],
[ 3., 100.]])
#include "iostream"
#include "torch/script.h"
using namespace torch::indexing;
int main()
{
torch::Tensor a = torch::linspace(1, 4, 4).reshape({2, 2});
std::cout << a << std::endl;
a.index_put_({"...", 1}, 100);
std::cout << a << std::endl;
return 0;
}
/***************运行结果****************/
1 2
3 4
[ CPUFloatType{2,2} ]
1 100
3 100
[ CPUFloatType{2,2} ]
3、tensor[torch.tensor([1, 2])] = 1 --> tensor.index_put_({torch::tensor({1, 2})}, 1)
import torch
a = torch.linspace(1,4,4)
b = torch.tensor([0, 2])
print(a)
a[b] = 100
print(a)
#===============运行结果==================#
tensor([1., 2., 3., 4.])
tensor([100., 2., 100., 4.])
#include "iostream"
#include "torch/script.h"
using namespace torch::indexing;
int main()
{
torch::Tensor a = torch::linspace(1, 4, 4);
torch::Tensor b = torch::tensor({0, 2});
std::cout << a << std::endl;
a.index_put_({b}, 100);
std::cout << a << std::endl;
return 0;
}
/*****************运行结果*****************/
1
2
3
4
[ CPUFloatType{4} ]
100
2
100
4
[ CPUFloatType{4} ]
三、掩码操作
按理说这部分也算索引的一部分,为什么要拿出来单独说呐?因为他比较特殊。首先看下例子吧。
在PyTorch中,我们使用Python的切片方式可以方便地通过掩码向量或矩阵来获取想要的tensor区域,比如以下操作:
import torch
a = torch.randint(0, 9,(4,4)) # 创建shape为4*4,值为[0,9]的随机整数的tensor
b = torch.tensor([0, 1, 0, 1]).bool() # 创建bool值向量,最终的结果是对应行向量的取舍
c = torch.tensor([0, 1, 0, 1]) # 注意这里不是bool值,最终的结果只是按行索引
a1 = a[b] # 掩码操作
a2 = a[c] # 索引操作
print('a:', a)
print('b:', b)
print('c:', c)
print('a1:', a1)
print('a2:', a2)
#=================运行结果===================#
a: tensor([[8, 0, 4, 8],
[5, 7, 7, 3],
[0, 5, 8, 1],
[3, 3, 2, 1]])
b: tensor([False, True, False, True])
c: tensor([0, 1, 0, 1])
a1: tensor([[5, 7, 7, 3],
[3, 3, 2, 1]])
a2: tensor([[8, 0, 4, 8],
[5, 7, 7, 3],
[8, 0, 4, 8],
[5, 7, 7, 3]])
在PyThon的C++API即LibTorch中,也有类似的操作,举例如下:
#include "iostream"
#include "ATen/Parallel.h"
#include "torch/script.h"
#include <c10/core/ScalarType.h>
using namespace torch::indexing;
int main()
{
torch::Tensor a = torch::randint(0, 9, {4,4}); // 初始化
torch::Tensor b = at::tensor({0,1,0,1}).toType(at::kBool); // bool型掩码向量,注意与非bool型的结果区别
torch::Tensor c = at::tensor({0,1,0,1}).toType(at::kLong); // 非bool型按行索引(这里的索引用tensor必须是long, byte or bool类型的)
torch::Tensor a1 = a.index({b}); // 掩码操作
torch::Tensor a2 = a.index({c}); // 按行索引
std::cout << a << std::endl;
std::cout << b << std::endl;
std::cout << c << std::endl;
std::cout << a1 << std::endl;
std::cout << a2 << std::endl;
return 0;
}
//==================运行结果====================//
7 5 8 0
1 0 1 2
0 6 6 3
2 1 5 3
[ CPUFloatType{4,4} ]
0
1
0
1
[ CPUBoolType{4} ]
0
1
0
1
[ CPULongType{4} ]
1 0 1 2
2 1 5 3
[ CPUFloatType{2,4} ]
7 5 8 0
1 0 1 2
7 5 8 0
1 0 1 2
[ CPUFloatType{4,4} ]
所以一定要注意0和1在bool型与非bool型情况下对索引结果的影响!
另外,LibTorch还有一个masked_select()方法,该方法是按照对应位置上的掩码值(bool值)来抽取值,先看定义:
inline Tensor Tensor::masked_select(const Tensor & mask)
其中mask必须是与要操作的tensor同shape或拥有相同数量元素的tensor,不满足条件就会自动复制补全,直至与目标tensor具有相同数量的元素。
举例如下:
#include "iostream"
#include "torch/script.h"
#include <c10/core/ScalarType.h>
using namespace torch::indexing;
int main()
{
torch::Tensor a = torch::randint(0, 9, {4,4}); // 初始化
torch::Tensor b = a > 5; // 获取满足条件的bool矩阵
torch::Tensor c = a.masked_select({b}); // 按照bool矩阵来取舍对应的值
std::cout << a << std::endl;
std::cout << b << std::endl;
std::cout << c << std::endl;
return 0;
}
//====================运行结果=======================//
5 2 8 8
1 0 3 0
2 2 4 4
8 4 7 5
[ CPUFloatType{4,4} ]
0 0 1 1
0 0 0 0
0 0 0 0
1 0 1 0
[ CPUBoolType{4,4} ]
8
8
8
7
[ CPUFloatType{4} ]
注:这里当时测试用的具体libtorch版本记不清了,不同版本间的操作可能会有差异
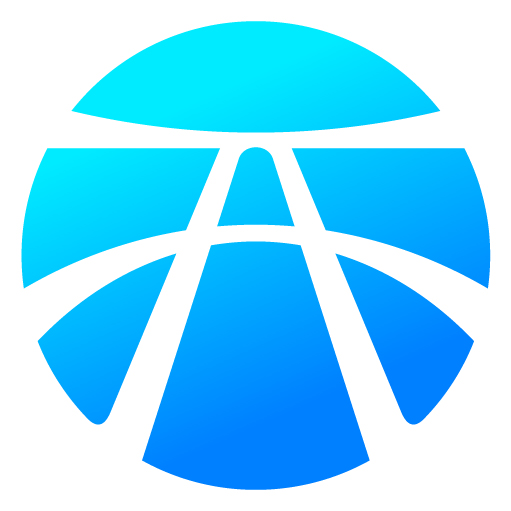
开放原子开发者工作坊旨在鼓励更多人参与开源活动,与志同道合的开发者们相互交流开发经验、分享开发心得、获取前沿技术趋势。工作坊有多种形式的开发者活动,如meetup、训练营等,主打技术交流,干货满满,真诚地邀请各位开发者共同参与!
更多推荐
所有评论(0)