pytroch中ctx和self的区别
welcome to my blog阅读某个pytorch模型源代码时碰见的ctx参数, 查阅了资料大概总结一下ctx貌似是context的缩写, 翻译成"上下文; 环境"ctx专门用在静态方法中self指的是实例对象; 而ctx用在静态方法中, 调用的时候不需要实例化对象, 直接通过类名就可以调用, 所以self在静态方法中没有意义ctx.save_for_backward(a, b...
·
welcome to my blog
阅读某个pytorch模型源代码时碰见的ctx参数, 查阅了资料大概总结一下
- ctx是context的缩写, 翻译成"上下文; 环境"
- ctx专门用在静态方法中
- self指的是实例对象; 而ctx用在静态方法中, 调用的时候不需要实例化对象, 直接通过类名就可以调用, 所以self在静态方法中没有意义
- 自定义的forward()方法和backward()方法的第一个参数必须是ctx; ctx可以保存forward()中的变量,以便在backward()中继续使用, 下一条是具体的示例
ctx.save_for_backward(a, b)
能够保存forward()静态方法中的张量, 从而可以在backward()静态方法中调用, 具体地, 下面地代码通过a, b = ctx.saved_tensors
重新得到a和bctx.needs_input_grad
是一个元组, 元素是True或者False, 表示forward()中对应的输入是否需要求导, 比如ctx.needs_input_grad[0]
指的是下面forwad()代码中indices是否需要求导
class SpecialSpmmFunction(torch.autograd.Function):
"""
Special function for only sparse region backpropataion layer.
"""
# 自定义前向传播过程
@staticmethod
def forward(ctx, indices, values, shape, b):
assert indices.requires_grad == False
a = torch.sparse_coo_tensor(indices, values, shape)
ctx.save_for_backward(a, b)
ctx.N = shape[0]
return torch.matmul(a, b)
# 自定义反向传播过程
@staticmethod
def backward(ctx, grad_output):
a, b = ctx.saved_tensors
grad_values = grad_b = None
if ctx.needs_input_grad[1]:
grad_a_dense = grad_output.matmul(b.t())
edge_idx = a._indices()[0, :] * ctx.N + a._indices()[1, :]
grad_values = grad_a_dense.view(-1)[edge_idx]
if ctx.needs_input_grad[3]:
grad_b = a.t().matmul(grad_output)
return None, grad_values, None, grad_b
ctx还能调用很多方法, pytorch1.3.1源码中竟然说"no doc", 没有相关的文档…
class _FunctionBase(object):
# no doc
@classmethod
def apply(cls, *args, **kwargs): # real signature unknown
pass
def register_hook(self, *args, **kwargs): # real signature unknown
pass
def _do_backward(self, *args, **kwargs): # real signature unknown
pass
def _do_forward(self, *args, **kwargs): # real signature unknown
pass
def _register_hook_dict(self, *args, **kwargs): # real signature unknown
pass
def __init__(self, *args, **kwargs): # real signature unknown
pass
@staticmethod # known case of __new__
def __new__(*args, **kwargs): # real signature unknown
""" Create and return a new object. See help(type) for accurate signature. """
pass
dirty_tensors = property(lambda self: object(), lambda self, v: None, lambda self: None) # default
metadata = property(lambda self: object(), lambda self, v: None, lambda self: None) # default
needs_input_grad = property(lambda self: object(), lambda self, v: None, lambda self: None) # default
next_functions = property(lambda self: object(), lambda self, v: None, lambda self: None) # default
non_differentiable = property(lambda self: object(), lambda self, v: None, lambda self: None) # default
requires_grad = property(lambda self: object(), lambda self, v: None, lambda self: None) # default
saved_tensors = property(lambda self: object(), lambda self, v: None, lambda self: None) # default
saved_variables = property(lambda self: object(), lambda self, v: None, lambda self: None) # default
to_save = property(lambda self: object(), lambda self, v: None, lambda self: None) # default
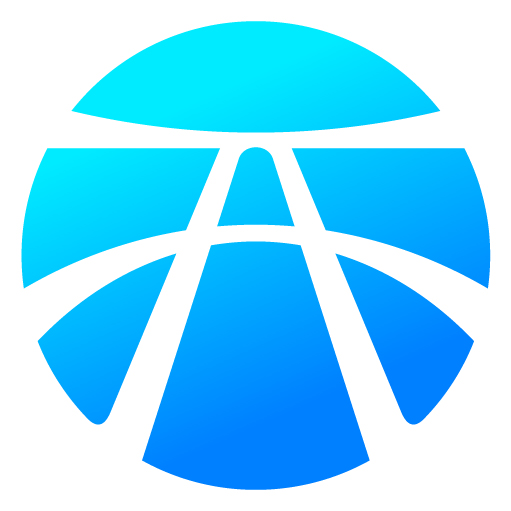
开放原子开发者工作坊旨在鼓励更多人参与开源活动,与志同道合的开发者们相互交流开发经验、分享开发心得、获取前沿技术趋势。工作坊有多种形式的开发者活动,如meetup、训练营等,主打技术交流,干货满满,真诚地邀请各位开发者共同参与!
更多推荐
所有评论(0)