Prometheus运维十二 Prometheus存储与高可用集群
海阔凭鱼跃,天高任鸟飞
海阔凭鱼跃,天高任鸟飞
文章目录
1.Prometheus存储
Prometheus包括本地磁盘时间序列数据库,可以选择与远程存储集成。
存储设计原理简介
时序数据的存储分俩个维度,如下图所示,纵轴表示所有存储的序列数据,横轴表示数据的时间分布:
promutheus 会定期拉取数据,从横轴的右侧垂直写入数据, 而我们在查询的时候,往往是查询图中任意矩形区域的数据,时序数据的存储和读取模式有着很大的差异,为了同时满足写入和查询两种不同需求,对存储层的时间有比较高的要求,如果在 k8s 环境,由于 pod 不断的新建和重启,时序数据会变成如下图的线性分布,更是提高了存储和查询难度。
1.1 本地存储
时间分片
Prometheus采用自定义的存储格式将样本数据保存在本地磁盘当中。
存储数据按照两个小时为一个时间窗口,将两小时内产生的数据存储在一个块(Block)中 也就是目录中,每一个块中包含该时间窗口内的所有样本数据(chunks),元数据文件(meta.json)以及索引文件(index)。
t0 t1 t2 now
┌───────────┐ ┌───────────┐ ┌───────────┐
│ │ │ │ │ │ ┌────────────┐
│ │ │ │ │ mutable │ <─── write ──── ┤ Prometheus │
│ │ │ │ │ │ └────────────┘
└───────────┘ └───────────┘ └───────────┘ ^
└──────────────┴───────┬──────┘ │
│ query
│ │
merge ──────────────────────────────────┘
当前时间窗口内正在收集的样本数据,Prometheus则会直接将数据保存在内存当中。
当Prometheus服务器重新启动时,可以通过重播日志(WAL)防止崩溃。
**预写日志文件wal以128MB的段存储在目录中。**这些文件包含尚未压缩的原始数据。因此,它们比常规的阻止文件大得多。Prometheus将至少保留三个预写日志文件。高流量的服务器可能会保留三个以上的WAL文件,以保留至少两个小时的原始数据。
在文件系统中这些块保存在单独的目录当中,Prometheus保存块数据的目录结构如下所示:
./data
├── 01BKGV7JBM69T2G1BGBGM6KB12 # 块
│ └── meta.json # 元数据
├── 01BKGTZQ1SYQJTR4PB43C8PD98
│ ├── chunks # 样本数据
│ │ └── 000001
│ ├── tombstones # 通过API方式对数据进行软删除,将删除记录存储在此处(API的删除方式,并不是立即将数据从chunks文件中移除)
│ ├── index # 索引文件
│ └── meta.json # 元数据
├── 01BKGTZQ1HHWHV8FBJXW1Y3W0K # 块
│ └── meta.json #元数据
├── 01BKGV7JC0RY8A6MACW02A2PJD
│ ├── chunks
│ │ └── 000001
│ ├── tombstones
│ ├── index
│ └── meta.json
├── chunks_head
│ └── 000001
└── wal # 写入日志
├── 000000002 #每n数据段最大为128M,存储默认存储两个小时的数据量。
└── checkpoint.00000001
└── 00000000
样本数据压缩
Prometheus 每秒会采集大量的数据,数据压缩势在必行,Prometheus 基于同一个序列的数据相似度比较高,在内存可以把每个数据点压缩到平均 1.37 byte 大小。
本地存储配置
--storage.tsdb.path:Prometheus写入数据库的位置。默认为data/。
--storage.tsdb.retention.time:何时删除旧数据。默认为15d。storage.tsdb.retention如果此标志设置为默认值以外的任何值,则覆盖。
--storage.tsdb.retention.size:[EXPERIMENTAL]要保留的最大存储块字节数。最旧的数据将首先被删除。默认为0或禁用。该标志是试验性的,将来的发行版中可能会更改。支持的单位:B,KB,MB,GB,TB,PB,EB。例如:“ 512MB”
--storage.tsdb.retention:不推荐使用storage.tsdb.retention.time。
--storage.tsdb.wal-compression:启用压缩预写日志(WAL)。根据您的数据,您可以预期WAL大小将减少一半,而额外的CPU负载却很少。该标志在2.11.0中引入,默认情况下在2.20.0中启用。请注意,一旦启用,将Prometheus降级到2.11.0以下的版本将需要删除WAL。
--storage.tsdb.max-block-duration:压缩块的最大时间戳范围,这是任何持久块的最小持续时间。
--storage.tsdb.no-lockfile:不要在数据目录中创建锁文件
一般情况下,Prometheus中存储的每一个样本大概占用1-2字节大小。如果需要对Prometheus Server的本地磁盘空间做容量规划时,可以通过以下公式计算:
needed_disk_space = retention_time_seconds * ingested_samples_per_second * bytes_per_sample
从上面公式中可以看出在保留时间(retention_time_seconds)和样本大小(bytes_per_sample)不变的情况下,如果想减少本地磁盘的容量需求,只能通过减少每秒获取样本数(ingested_samples_per_second)的方式。因此有两种手段,一是减少时间序列的数量,二是增加采集样本的时间间隔。考虑到Prometheus会对时间序列进行压缩效率,减少时间序列的数量效果更明显。
从失败中恢复
如果本地存储由于某种原因而损坏,解决该问题的最佳策略是关闭Prometheus,然后删除整个存储目录。也可以尝试删除单个块目录或WAL目录以解决问题。注意,这意味着每个块目录丢失大约两个小时的数据。再次,Prometheus的本地存储不旨在成为持久的长期存储;外部解决方案可提供更长的保留时间和数据持久性。
注意: Prometheus的本地存储不支持不兼容POSIX的文件系统,因为可能会发生不可恢复的损坏。不支持NFS文件系统(包括AWS的EFS)。NFS可能符合POSIX,但大多数实现均不符合。强烈建议使用本地文件系统以提高可靠性。
1.2 远程存储
Prometheus的本地存储仅限于单个节点的可伸缩性和持久性,减少其自身运维和管理的复杂度。
能够满足大部分用户监控规模的需求。但是本地存储也意味着Prometheus无法持久化数据,无法存储大量历史数据,同时也无法灵活扩展和迁移。
为了保持Prometheus的简单性,Prometheus并没有尝试在自身中解决以上问题,而是通过定义两个标准接口(remote_write/remote_read),让用户可以基于这两个接口对接将数据保存到任意第三方的存储服务中,这种方式在Promthues中称为Remote Storage。
概述
Prometheus通过两种方式与远程存储系统集成:
1.Prometheus可以将其提取的样本以标准格式写入远程URL。
2.Prometheus可以以标准化格式从远程URL读取(返回)样本数据。
Remote Write
用户可以在Prometheus配置文件中指定Remote Write(远程写)的URL地址,一旦设置了该配置项,Prometheus将采集到的样本数据通过HTTP的形式发送给适配器(Adaptor)。而用户则可以在适配器中对接外部任意的服务。外部服务可以是真正的存储系统,公有云的存储服务,也可以是消息队列等任意形式。
Remote Read
Promthues的Remote Read(远程读)也通过了一个适配器实现。在远程读的流程当中,当用户发起查询请求后,Promthues将向remote_read中配置的URL发起查询请求(matchers,ranges),Adaptor根据请求条件从第三方存储服务中获取响应的数据。同时将数据转换为Promthues的原始样本数据返回给Prometheus Server。
当获取到样本数据后,Promthues在本地使用PromQL对样本数据进行二次处理。
注意:启用远程读设置后,只在数据查询时有效,对于规则文件的处理,以及Metadata API的处理都只基于Prometheus本地存储完成。
配置文件
Prometheus配置文件中添加remote_write和remote_read配置,其中url用于指定远程读/写的HTTP服务地址。如果该URL启动了认证则可以通过basic_auth进行安全认证配置。对于https的支持需要设定tls_concig。proxy_url主要用于Prometheus无法直接访问适配器服务的情况下。
remote_write和remote_write具体配置如下所示:
remote_write:
url: <string>
[ remote_timeout: <duration> | default = 30s ]
write_relabel_configs:
[ - <relabel_config> ... ]
basic_auth:
[ username: <string> ]
[ password: <string> ]
[ bearer_token: <string> ]
[ bearer_token_file: /path/to/bearer/token/file ]
tls_config:
[ <tls_config> ]
[ proxy_url: <string> ]
remote_read:
url: <string>
required_matchers:
[ <labelname>: <labelvalue> ... ]
[ remote_timeout: <duration> | default = 30s ]
[ read_recent: <boolean> | default = false ]
basic_auth:
[ username: <string> ]
[ password: <string> ]
[ bearer_token: <string> ]
[ bearer_token_file: /path/to/bearer/token/file ]
[ <tls_config> ]
[ proxy_url: <string> ]
详细文档:https://prometheus.io/docs/prometheus/latest/configuration/configuration/#remote_write
自定义Remote Storage Adaptor
实现自定义Remote Storage需要用户分别创建用于支持remote_read和remote_write的HTTP服务。
当前Prometheus中Remote Storage相关的协议主要通过以下proto文件进行定义:
syntax = "proto3";
package prometheus;
option go_package = "prompb";
import "types.proto";
message WriteRequest {
repeated prometheus.TimeSeries timeseries = 1;
}
message ReadRequest {
repeated Query queries = 1;
}
message ReadResponse {
// In same order as the request's queries.
repeated QueryResult results = 1;
}
message Query {
int64 start_timestamp_ms = 1;
int64 end_timestamp_ms = 2;
repeated prometheus.LabelMatcher matchers = 3;
}
message QueryResult {
// Samples within a time series must be ordered by time.
repeated prometheus.TimeSeries timeseries = 1;
}
以下代码展示了一个简单的remote_write服务,创建用于接收remote_write的HTTP服务,将请求内容转换成WriteRequest后,用户就可以按照自己的需求进行后续的逻辑处理。
package main
import (
"fmt"
"io/ioutil"
"net/http"
"github.com/gogo/protobuf/proto"
"github.com/golang/snappy"
"github.com/prometheus/common/model"
"github.com/prometheus/prometheus/prompb"
)
func main() {
http.HandleFunc("/receive", func(w http.ResponseWriter, r *http.Request) {
compressed, err := ioutil.ReadAll(r.Body)
if err != nil {
http.Error(w, err.Error(), http.StatusInternalServerError)
return
}
reqBuf, err := snappy.Decode(nil, compressed)
if err != nil {
http.Error(w, err.Error(), http.StatusBadRequest)
return
}
var req prompb.WriteRequest
if err := proto.Unmarshal(reqBuf, &req); err != nil {
http.Error(w, err.Error(), http.StatusBadRequest)
return
}
for _, ts := range req.Timeseries {
m := make(model.Metric, len(ts.Labels))
for _, l := range ts.Labels {
m[model.LabelName(l.Name)] = model.LabelValue(l.Value)
}
fmt.Println(m)
for _, s := range ts.Samples {
fmt.Printf(" %f %d\n", s.Value, s.Timestamp)
}
}
})
http.ListenAndServe(":1234", nil)
}
使用Influxdb作为Remote Storage
相关存储集成文档:https://prometheus.io/docs/operating/integrations/#remote-endpoints-and-storage
使用Influxdb作为Prometheus的Remote Storage,从而确保当Prometheus发生宕机或者重启之后能够从Influxdb中恢复和获取历史数据。
这里使用docker-compose定义并启动Influxdb数据库服务,docker-compose.yml定义如下:
version: '2'
services:
influxdb:
image: influxdb:1.3.5
command: -config /etc/influxdb/influxdb.conf
ports:
- "8086:8086"
environment:
- INFLUXDB_DB=prometheus
- INFLUXDB_ADMIN_ENABLED=true
- INFLUXDB_ADMIN_USER=admin
- INFLUXDB_ADMIN_PASSWORD=admin
- INFLUXDB_USER=prom
- INFLUXDB_USER_PASSWORD=prom
启动influxdb服务
docker-compose up -d
获取并启动Prometheus提供的Remote Storage Adapter:
go get github.com/prometheus/prometheus/documentation/examples/remote_storage/remote_storage_adapter
获取remote_storage_adapter源码后,go会自动把相关的源码编译成可执行文件,并且保存在$GOPATH/bin/目录下。
启动remote_storage_adapter并且设置Influxdb相关的认证信息:
INFLUXDB_PW=prom $GOPATH/bin/remote_storage_adapter -influxdb-url=http://localhost:8086 -influxdb.username=prom -influxdb.database=prometheus -influxdb.retention-policy=autogen
修改prometheus.yml添加Remote Storage相关的配置内容:
remote_write:
- url: "http://localhost:9201/write"
remote_read:
- url: "http://localhost:9201/read"
重新启动Prometheus能够获取数据后,登录到influxdb容器,并验证数据写入。如下所示,当数据能够正常写入Influxdb后可以看到Prometheus相关的指标。
docker exec -it 795d0ead87a1 influx
Connected to http://localhost:8086 version 1.3.5
InfluxDB shell version: 1.3.5
> auth
username: prom
password:
> use prometheus
> SHOW MEASUREMENTS
name: measurements
name
----
go_gc_duration_seconds
go_gc_duration_seconds_count
go_gc_duration_seconds_sum
go_goroutines
go_info
go_memstats_alloc_bytes
go_memstats_alloc_bytes_total
go_memstats_buck_hash_sys_bytes
go_memstats_frees_total
go_memstats_gc_cpu_fraction
go_memstats_gc_sys_bytes
go_memstats_heap_alloc_bytes
go_memstats_heap_idle_bytes
当数据写入成功后,停止Prometheus服务。同时删除Prometheus的data目录,模拟Promthues数据丢失的情况后重启Prometheus。打开Prometheus UI如果配置正常,Prometheus可以正常查询到本地存储的已删除的历史数据记录。
2.联邦集群
官方解释:https://prometheus.io/docs/prometheus/latest/federation/
2.1分层联邦
分层联邦允许Prometheus扩展到数十个数据中心和数百万个节点的环境。在这种用例中,联邦拓扑类似于一棵树,更高级别的Prometheus服务器从大量的从属服务器收集汇总的时间序列数据。
2.2 跨服务联邦
在跨服务联合中,一个服务的普罗米修斯服务器被配置为从另一个服务的普罗米修斯服务器中刮取选定的数据,以使得能够针对单个服务器内的两个数据集进行警报和查询。
例如,运行多个服务的集群调度程序可能会暴露有关在集群上运行的服务实例的资源使用情况信息(如内存和CPU使用情况)。另一方面,在该群集上运行的服务将仅公开特定(指定)的应用程序的服务度量标准。通常,这两套指标是由单独的Prometheus来抓取的。使用联邦,包含服务级别度量的Prometheus服务器可以从集群Prometheus拉入有关其特定服务的集群资源使用度量,以便这两组度量可以在该服务器内使用。
对于大部分监控规模而言,我们只需要在每一个数据中心(例如:EC2可用区,Kubernetes集群)安装一个Prometheus Server实例,就可以在各个数据中心处理上千规模的集群。同时将Prometheus Server部署到不同的数据中心可以避免网络配置的复杂性。
如上图所示,在每个数据中心部署单独的Prometheus Server,用于采集当前数据中心监控数据。并由一个中心的Prometheus Server负责聚合多个数据中心的监控数据。这一特性在Promthues中称为联邦集群。
例如:
Prometheus的联邦集群使用它来作为Prometheus代理。因为我们是在监控rancher平台里面的docker容器里面的应用,那么拿到的就是容器的ip,而我们实际的Prometheus是部署在外部虚拟机上面的。这个时候外部的Prometheus就无法拿到rancher平台内部容器应用的metrics,所以部署一台prometheus到rancher组成联邦机,详细的官网有解释:federate,总体架构图如下
2.3 配置联邦
在任何Prometheus服务器上,/federate 端点都允许检索该服务器中所选时间序列集的当前值。
match[]必须至少指定一个 URL参数以选择要公开的系列。每个 match[]参数都需要指定一个 即时矢量选择器,例如 up或{job="api-server"}。如果match[]
提供了多个参数,则选择所有匹配系列的并集。
要将度量标准从一台服务器联合到另一台服务器,请将目标Prometheus服务器配置为从 /federate 源服务器的端点进行抓取,同时还启用honor_labelsscrape选项(不覆盖源服务器暴露的任何标签)并传递所需的match[] 参数。
例如,以下命令将从Prometheus服务器配置的scrape_configs中带有任何标签job="prometheus"或度量标准名称的系列联合抓取Prometheus中:job:source-prometheus-{1,2,3}:9090的数据。
scrape_configs:
- job_name: 'federate'
scrape_interval: 15s
honor_labels: true
metrics_path: '/federate'
params:
'match[]':
- '{job="prometheus"}'
- '{__name__=~"job:.*"}'
- '{job="xxx"}'
- '{job="xxx"}'
static_configs:
- targets:
- 'source-prometheus-1:9090'
- 'source-prometheus-2:9090'
- 'source-prometheus-3:9090'
为了有效的减少不必要的时间序列,通过params参数可以用于指定只获取某些时间序列的样本数据,例如
"http://xxx.xxx.xxx.xxx:9090/federate?match[]={job%3D"prometheus"}&match[]={__name__%3D~"job%3A.*"}&match[]={__name__%3D~"node.*"}"
通过URL中的match[]参数指定我们可以指定需要获取的时间序列。match[]参数必须是一个瞬时向量选择器,例如up或者{job=“api-server”}。配置多个match[]参数,用于获取多组时间序列的监控数据。
horbor_labels配置true可以确保当采集到的监控指标冲突时,能够自动忽略冲突的监控数据。如果为false时,prometheus会自动将冲突的标签替换为”exported_“的形式。
2.4 功能划分
联邦集群的特性可以帮助用户根据不同的监控规模对Promthues部署架构进行调整。例如如下所示,可以在各个数据中心中部署多个Prometheus Server实例。每一个Prometheus Server实例只负责采集当前数据中心中的一部分任务(Job),例如可以将不同的监控任务分离到不同的Prometheus实例当中,再有中心Prometheus实例进行聚合。
3. Prometheus高可用
远程存储解决了Prometheus的数据持久化和可扩展性的问题,联邦解决单台Prometheus的瓶颈压力,数据采集问题。将其汇总在一起可以组合成Prometheus的高可用集群。
如下介绍了几种集群方案:
3.1 基本HA: 服务可用性
由于Promthues的Pull机制的设计,为了确保Promthues服务的可用性,用户只需要部署多套Prometheus Server实例,并且采集相同的Exporter目标即可
基本的HA模式只能确保Promthues服务的可用性问题,但是不解决Prometheus Server之间的数据一致性问题以及持久化问题(数据丢失后无法恢复),也无法进行动态的扩展。因此这种部署方式适合监控规模不大,Promthues Server也不会频繁发生迁移的情况,并且只需要保存短周期监控数据的场景。
3.2 基本HA+远程存储
在基本HA模式的基础上通过添加Remote Storage存储支持,将监控数据保存在第三方存储服务上。
在解决了Promthues服务可用性的基础上,同时确保了数据的持久化,当Promthues Server发生宕机或者数据丢失的情况下,可以快速的恢复。 同时Promthues Server可能很好的进行迁移。因此,该方案适用于用户监控规模不大,但是希望能够将监控数据持久化,同时能够确保Promthues Server的可迁移性的场景。
3.3 基本HA+远程存储+联邦集群
当单台Promthues Server无法处理大量的采集任务时,用户可以考虑基于Prometheus联邦集群的方式将监控采集任务划分到不同的Promthues实例当中即在任务级别功能分区。
这种部署方式一般适用于两种场景:
场景一:单数据中心 + 大量的采集任务
这种场景下Promthues的性能瓶颈主要在于大量的采集任务,因此用户需要利用Prometheus联邦集群的特性,将不同类型的采集任务划分到不同的Promthues子服务中,从而实现功能分区。例如一个Promthues Server负责采集基础设施相关的监控指标,另外一个Prometheus Server负责采集应用监控指标。再有上层Prometheus Server实现对数据的汇聚。
场景二:多数据中心
这种模式也适合与多数据中心的情况,当Promthues Server无法直接与数据中心中的Exporter进行通讯时,在每一个数据中部署一个单独的Promthues Server负责当前数据中心的采集任务是一个不错的方式。这样可以避免用户进行大量的网络配置,只需要确保主Promthues Server实例能够与当前数据中心的Prometheus Server通讯即可。 中心Promthues Server负责实现对多数据中心数据的聚合。
3.4 按照实例进行功能分区
单个采集任务的Target数也变得非常巨大。这时简单通过联邦集群进行功能分区,Prometheus Server也无法有效处理时。这种情况只能考虑继续在实例级别进行功能划分。
如上图所示,将统一任务的不同实例的监控数据采集任务划分到不同的Prometheus实例。通过relabel设置,我们可以确保当前Prometheus Server只收集当前采集任务的一部分实例的监控指标。
global:
external_labels:
slave: 1 # This is the 2nd slave. This prevents clashes between slaves.
scrape_configs:
- job_name: some_job
relabel_configs:
- source_labels: [__address__]
modulus: 4
target_label: __tmp_hash
action: hashmod
- source_labels: [__tmp_hash]
regex: ^1$
action: keep
并且通过当前数据中心的一个中心Prometheus Server将监控数据进行聚合到任务级别。
- scrape_config:
- job_name: slaves
honor_labels: true
metrics_path: /federate
params:
match[]:
- '{__name__=~"^slave:.*"}' # Request all slave-level time series
static_configs:
- targets:
- slave0:9090
- slave1:9090
- slave3:9090
- slave4:9090
3.5 高可用方案选择
上面介绍了三中Prometheus集群的部署方案,需要根据业务需求来选择相应的集群方案。
选项\需求 | 服务可用性 | 数据持久化 | 水平扩展 |
---|---|---|---|
主备HA | V | X | X |
远程存储 | X | V | X |
联邦集群 | X | X | V |
4.Alertmanager高可用
为了提升Promthues的服务可用性,通常用户会部署两个或者两个以上的Promthus Server,它们具有完全相同的配置包括Job配置,以及告警配置等。当某一个Prometheus Server发生故障后可以确保Promthues持续可用。
同时基于Alertmanager的告警分组机制即使不同的Prometheus Sever分别发送相同的告警给Alertmanager(去重机制),Alertmanager也可以自动将这些告警合并为一个通知向receiver发送。
但虽然Alertmanager能够同时处理多个相同的Prometheus Server所产生的告警。但是由于单个Alertmanager的存在,当前的部署结构存在明显的单点故障风险,当Alertmanager单点失效后,告警的后续所有业务全部失效。
最直接的方式,就是尝试部署多套Alertmanager。但是由于Alertmanager之间不存在并不了解彼此的存在,因此则会出现告警通知被不同的Alertmanager重复发送多次的问题。
为了解决这一问题,如下所示。Alertmanager引入了Gossip机制。**Gossip机制为多个Alertmanager之间提供了信息传递的机制。**确保及时在多个Alertmanager分别接收到相同告警信息的情况下,也只有一个告警通知被发送给Receiver。
4.1 Gossip协议
Gossip是分布式系统中被广泛使用的协议,用于实现分布式节点之间的信息交换和状态同步。
Gossip协议同步状态类似于流言或者病毒的传播.
一般来说Gossip有两种实现方式分别为Push-based和Pull-based。
在Push-based当集群中某一节点A完成一个工作后,随机的从其它节点B并向其发送相应的消息,节点B接收到消息后在重复完成相同的工作,直到传播到集群中的所有节点。
Pull-based的实现中节点A会随机的向节点B发起询问是否有新的状态需要同步,如果有则返回。
Alertmanager是如何基于Gossip协议实现集群高可用的。
如下所示,当Alertmanager接收到来自Prometheus的告警消息后,会按照以下流程对告警进行处理:
1.在第一个阶段Silence中,Alertmanager会判断当前通知是否匹配到任何的静默规则,如果没有则进入下一个阶段,否则则中断流水线不发送通知。
2.在第二个阶段Wait中,Alertmanager会根据当前Alertmanager在集群中所在的顺序(index)等待index * 5s的时间。
3.当前Alertmanager等待阶段结束后,Dedup阶段则会判断当前Alertmanager数据库中该通知是否已经发送,如果已经发送则中断流水线,不发送告警,否则则进入下一阶段Send对外发送告警通知。
4.告警发送完成后该Alertmanager进入最后一个阶段Gossip,Gossip会通知其他Alertmanager实例当前告警已经发送。其他实例接收到Gossip消息后,则会在自己的数据库中保存该通知已发送的记录。
Gossip机制的关键在于两点:
1.Silence设置同步:Alertmanager启动阶段基于Pull-based从集群其它节点同步Silence状态,当有新的Silence产生时使用Push-based方式在集群中传播Gossip信息。
2.通知发送状态同步:告警通知发送完成后,基于Push-based同步告警发送状态。Wait阶段可以确保集群状态一致。
Alertmanager基于Gossip实现的集群机制虽然不能保证所有实例上的数据时刻保持一致,但是实现了CAP理论中的AP系统,即可用性和分区容错性。同时对于Prometheus Server而言保持了配置了简单性,Promthues Server之间不需要任何的状态同步。
搭建本地集群环境
为了能够让Alertmanager节点之间进行通讯,需要在Alertmanager启动时设置相应的参数。其中主要的参数包括:
--cluster.listen-address string: 当前实例集群服务监听地址
--cluster.peer value: 初始化时关联的其它实例的集群服务地址
例如:
定义Alertmanager实例a1,其中Alertmanager的服务运行在9093端口,集群服务地址运行在8001端口。
alertmanager --web.listen-address=":9093" --cluster.listen-address="127.0.0.1:8001" --config.file=/etc/prometheus/alertmanager.yml --storage.path=/data/alertmanager/
定义Alertmanager实例a2,其中主服务运行在9094端口,集群服务运行在8002端口。为了将a1,a2组成集群。 a2启动时需要定义–cluster.peer参数并且指向a1实例的集群服务地址:8001。
alertmanager --web.listen-address=":9094" --cluster.listen-address="127.0.0.1:8002" --cluster.peer=127.0.0.1:8001 --config.file=/etc/prometheus/alertmanager.yml --storage.path=/data/alertmanager2/
为了能够在本地模拟集群环境,这里使用了一个轻量级的多线程管理工具goreman。使用以下命令可以在本地安装goreman命令行工具。
go get github.com/mattn/goreman
创建Alertmanager集群
创建Alertmanager配置文件/etc/prometheus/alertmanager-ha.yml, 为了验证Alertmanager的集群行为,这里在本地启动一个webhook服务用于打印Alertmanager发送的告警通知信息。
route:
receiver: 'default-receiver'
receivers:
- name: default-receiver
webhook_configs:
- url: 'http://127.0.0.1:5001/'
本地webhook服务可以直接从Github获取。
# 获取alertmanager提供的webhook示例,如果该目录下定义了main函数,go get会自动将其编译成可执行文件
go get github.com/prometheus/alertmanager/examples/webhook
# 设置环境变量指向GOPATH的bin目录
export PATH=$GOPATH/bin:$PATH
# 启动服务
webhook
示例结构如下所示:
创建alertmanager.procfile文件,并且定义了三个Alertmanager节点(a1,a2,a3)以及用于接收告警通知的webhook服务:
a1: alertmanager --web.listen-address=":9093" --cluster.listen-address="127.0.0.1:8001" --config.file=/etc/prometheus/alertmanager-ha.yml --storage.path=/data/alertmanager/ --log.level=debug
a2: alertmanager --web.listen-address=":9094" --cluster.listen-address="127.0.0.1:8002" --cluster.peer=127.0.0.1:8001 --config.file=/etc/prometheus/alertmanager-ha.yml --storage.path=/data/alertmanager2/ --log.level=debug
a3: alertmanager --web.listen-address=":9095" --cluster.listen-address="127.0.0.1:8003" --cluster.peer=127.0.0.1:8001 --config.file=/etc/prometheus/alertmanager-ha.yml --storage.path=/data/alertmanager2/ --log.level=debug
webhook: webhook
在Procfile文件所在目录,执行goreman start命令,启动所有进程:
$ goreman -f alertmanager.procfile start
10:27:57 a1 | level=debug ts=2018-03-12T02:27:57.399166371Z caller=cluster.go:125 component=cluster msg="joined cluster" peers=0
10:27:57 a3 | level=info ts=2018-03-12T02:27:57.40004678Z caller=main.go:346 msg=Listening address=:9095
10:27:57 a1 | level=info ts=2018-03-12T02:27:57.400212246Z caller=main.go:271 msg="Loading configuration file" file=/etc/prometheus/alertmanager.yml
10:27:57 a1 | level=info ts=2018-03-12T02:27:57.405638714Z caller=main.go:346 msg=Listening address=:9093
启动完成后访问任意Alertmanager节点http://localhost:9093/#/status,可以查看当前Alertmanager集群的状态。
当集群中的Alertmanager节点不在一台主机时,通常需要使用–cluster.advertise-address参数指定当前节点所在网络地址。
注意:由于goreman不保证进程之间的启动顺序,如果集群状态未达到预期,可以使用goreman -f alertmanager.procfile run restart a2重启a2,a3服务。
当Alertmanager集群启动完成后,可以使用send-alerts.sh脚本对集群进行简单测试,这里利用curl分别向3个Alertmanager实例发送告警信息。
alerts1='[
{
"labels": {
"alertname": "DiskRunningFull",
"dev": "sda1",
"instance": "example1"
},
"annotations": {
"info": "The disk sda1 is running full",
"summary": "please check the instance example1"
}
},
{
"labels": {
"alertname": "DiskRunningFull",
"dev": "sdb2",
"instance": "example2"
},
"annotations": {
"info": "The disk sdb2 is running full",
"summary": "please check the instance example2"
}
},
{
"labels": {
"alertname": "DiskRunningFull",
"dev": "sda1",
"instance": "example3",
"severity": "critical"
}
},
{
"labels": {
"alertname": "DiskRunningFull",
"dev": "sda1",
"instance": "example3",
"severity": "warning"
}
}
]'
curl -XPOST -d"$alerts1" http://localhost:9093/api/v1/alerts
curl -XPOST -d"$alerts1" http://localhost:9094/api/v1/alerts
curl -XPOST -d"$alerts1" http://localhost:9095/api/v1/alerts
运行send-alerts.sh后,查看alertmanager日志,可以看到以下输出,3个Alertmanager实例分别接收到模拟的告警信息:
10:43:36 a1 | level=debug ts=2018-03-12T02:43:36.853370185Z caller=dispatch.go:188 component=dispatcher msg="Received alert" alert=DiskRunningFull[6543bc1][active]
10:43:36 a2 | level=debug ts=2018-03-12T02:43:36.871180749Z caller=dispatch.go:188 component=dispatcher msg="Received alert" alert=DiskRunningFull[8320f0a][active]
10:43:36 a3 | level=debug ts=2018-03-12T02:43:36.894923811Z caller=dispatch.go:188 component=dispatcher msg="Received alert" alert=DiskRunningFull[8320f0a][active]
查看webhook日志只接收到一个告警通知:
10:44:06 webhook | 2018/03/12 10:44:06 {
10:44:06 webhook | > "receiver": "default-receiver",
10:44:06 webhook | > "status": "firing",
10:44:06 webhook | > "alerts": [
10:44:06 webhook | > {
10:44:06 webhook | > "status": "firing",
10:44:06 webhook | > "labels": {
10:44:06 webhook | > "alertname": "DiskRunningFull",
多实例Prometheus与Alertmanager集群
由于Gossip机制的实现,在Promthues和Alertmanager实例之间不要使用任何的负载均衡,需要确保Promthues将告警发送到所有的Alertmanager实例中:
alerting:
alertmanagers:
- static_configs:
- targets:
- 127.0.0.1:9093
- 127.0.0.1:9094
- 127.0.0.1:9095
创建Promthues集群配置文件/etc/prometheus/prometheus-ha.yml,完整内容如下:
global:
scrape_interval: 15s
scrape_timeout: 10s
evaluation_interval: 15s
rule_files:
- /etc/prometheus/rules/*.rules
alerting:
alertmanagers:
- static_configs:
- targets:
- 127.0.0.1:9093
- 127.0.0.1:9094
- 127.0.0.1:9095
scrape_configs:
- job_name: prometheus
static_configs:
- targets:
- localhost:9090
- job_name: 'node'
static_configs:
- targets: ['localhost:9100']
同时定义告警规则文件/etc/prometheus/rules/hoststats-alert.rules,如下所示:
groups:
- name: hostStatsAlert
rules:
- alert: hostCpuUsageAlert
expr: sum(avg without (cpu)(irate(node_cpu{mode!='idle'}[5m]))) by (instance) * 100 > 50
for: 1m
labels:
severity: page
annotations:
summary: "Instance {{ $labels.instance }} CPU usgae high"
description: "{{ $labels.instance }} CPU usage above 50% (current value: {{ $value }})"
- alert: hostMemUsageAlert
expr: (node_memory_MemTotal - node_memory_MemAvailable)/node_memory_MemTotal * 100 > 85
for: 1m
labels:
severity: page
annotations:
summary: "Instance {{ $labels.instance }} MEM usgae high"
description: "{{ $labels.instance }} MEM usage above 85% (current value: {{ $value }})"
示例部署结构如下所示:
创建prometheus.procfile文件,创建两个Promthues节点,分别监听9090和9091端口:
p1: prometheus --config.file=/etc/prometheus/prometheus-ha.yml --storage.tsdb.path=/data/prometheus/ --web.listen-address="127.0.0.1:9090"
p2: prometheus --config.file=/etc/prometheus/prometheus-ha.yml --storage.tsdb.path=/data/prometheus2/ --web.listen-address="127.0.0.1:9091"
node_exporter: node_exporter -web.listen-address="0.0.0.0:9100"
使用goreman启动多节点Promthues:
goreman -f prometheus.procfile -p 8556 start
Promthues启动完成后,手动拉高系统CPU使用率:
cat /dev/zero>/dev/null
注意,对于多核主机,如果CPU达不到预期,运行多个命令。
当CPU利用率达到告警规则触发条件,两个Prometheus实例告警分别被触发。查看Alertmanager输出日志:
11:14:41 a3 | level=debug ts=2018-03-12T03:14:41.945493505Z caller=dispatch.go:188 component=dispatcher msg="Received alert" alert=hostCpuUsageAlert[7d698ac][active]
11:14:41 a1 | level=debug ts=2018-03-12T03:14:41.945534548Z caller=dispatch.go:188 component=dispatcher msg="Received alert" alert=hostCpuUsageAlert[7d698ac][active]
11:14:41 a2 | level=debug ts=2018-03-12T03:14:41.945687812Z caller=dispatch.go:188 component=dispatcher msg="Received alert" alert=hostCpuUsageAlert[7d698ac][active]
3个Alertmanager实例分别接收到来自不同Prometheus实例的告警信息。而Webhook服务只接收到来自Alertmanager集群的一条告警通知:
11:15:11 webhook | 2018/03/12 11:15:11 {
11:15:11 webhook | > "receiver": "default-receiver",
11:15:11 webhook | > "status": "firing",
11:15:11 webhook | > "alerts": [
11:15:11 webhook | > {
11:15:11 webhook | > "status": "firing",
11:15:11 webhook | > "labels": {
11:15:11 webhook | > "alertname": "hostCpuUsageAlert",
参考文献:
https://opensource.actionsky.com/20200825-prometheus/
https://yunlzheng.gitbook.io/prometheus-book/part-ii-prometheus-jin-jie/readmd/prometheus-local-storage
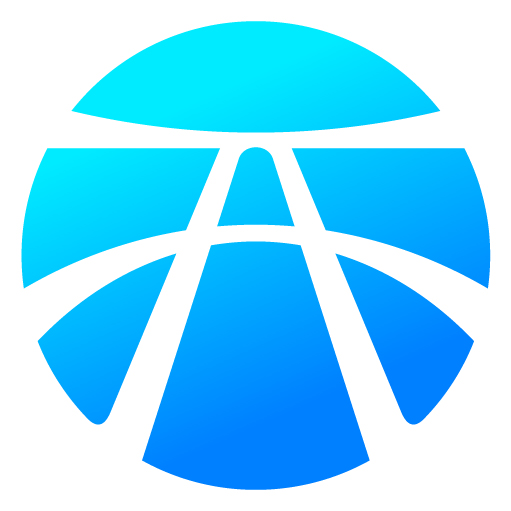
开放原子开发者工作坊旨在鼓励更多人参与开源活动,与志同道合的开发者们相互交流开发经验、分享开发心得、获取前沿技术趋势。工作坊有多种形式的开发者活动,如meetup、训练营等,主打技术交流,干货满满,真诚地邀请各位开发者共同参与!
更多推荐
所有评论(0)