loss函数之PoissonNLLLoss,GaussianNLLLoss
PoissonNLLLoss真实标签服从泊松分布的负对数似然损失泊松分布:P(Y=k)=λkk!e−λP(Y=k)=\frac{\lambda^{k}}{k !} e^{-\lambda}P(Y=k)=k!λke−λk是target$\lambda$ 是inputtarget∼Poisson(input)loss(input,target)=input−target∗log(input)+log
PoissonNLLLoss
真实标签服从泊松分布的负对数似然损失,神经网络的输出作为泊松分布的参数 λ \lambda λ。
泊松分布是一种离散分布,概率计算如下:
P ( Y = k ) = λ k k ! e − λ P(Y=k)=\frac{\lambda^{k}}{k !} e^{-\lambda} P(Y=k)=k!λke−λ
对于包含 N N N个样本的batch数据 D ( x , y ) D(x, y) D(x,y), y y y是样本对应的类别标签,服从泊松分布。 x x x与 y y y的维度相同。
(1)若 x x x是神经网络的输出,且未进行归一化和对数化处理。第 n n n个样本对应的损失 l n l_{n} ln 为:
由泊松分布的公式得到:
P
(
Y
=
y
n
)
=
x
n
y
n
y
n
!
e
−
x
n
P(Y=y_{n})=\frac{x_{n}^{y_{n}}}{y_{n}!} e^{-x_{n}}
P(Y=yn)=yn!xnyne−xn
l
n
=
−
l
o
g
P
(
Y
=
y
n
)
=
x
n
−
y
n
l
o
g
x
n
+
l
o
g
(
y
n
!
)
l_{n}=-logP(Y=y_{n}) =x_{n}-y_{n}logx_{n}+ log(y_{n}!)
ln=−logP(Y=yn)=xn−ynlogxn+log(yn!)
(2)若 x x x 是神经网络的输出,进行了归一化和对数化处理。第 n n n个样本对应的损失 l n l_{n} ln 为:
将(1) 公式中的
x
n
x_{n}
xn 替换为
e
x
p
(
x
n
)
exp(x_{n})
exp(xn),
l
o
g
x
n
logx_{n}
logxn替换为
x
n
x_{n}
xn
l
n
=
−
l
o
g
P
(
Y
=
y
n
)
=
e
x
p
(
x
n
)
−
y
n
x
n
+
l
o
g
(
y
n
!
)
l_{n}=-logP(Y=y_{n}) =exp(x_{n}) -y_{n}x_{n}+ log(y_{n}!)
ln=−logP(Y=yn)=exp(xn)−ynxn+log(yn!)
最后一项 l o g ( y n ! ) log(y_{n}!) log(yn!)可以省略或者用斯特林公式(Stirling’s formula)近似。
一个样本可能对应多个输出,每个输出都服从泊松分布,有自己的泊松分布参数。因此, l n l_{n} ln可能是一个值,也可能是一个向量。
class PoissonNLLLoss(_Loss):
__constants__ = ['log_input', 'full', 'eps', 'reduction']
def __init__(self, log_input=True, full=False, size_average=None,
eps=1e-8, reduce=None, reduction='mean'):
super(PoissonNLLLoss, self).__init__(size_average, reduce, reduction)
self.log_input = log_input
self.full = full
self.eps = eps
def forward(self, log_input, target):
return F.poisson_nll_loss(log_input, target, log_input=self.log_input, full=self.full,
eps=self.eps, reduction=self.reduction)
pytorch中通过torch.nn.PoissonNLLLoss
类实现,也可以直接调用F.poisson_nll_loss
函数,代码中的size_average
与reduce
已经弃用。reduction有三种取值mean
, sum
, none
,对应不同的返回
ℓ
(
x
,
y
)
\ell(x, y)
ℓ(x,y)。 默认为mean
,对应于一般情况下
l
o
s
s
loss
loss的计算
L = { l 1 , … , l N } L=\left\{l_{1}, \ldots, l_{N}\right\} L={l1,…,lN}
ℓ ( x , y ) = { L , if reduction = ’none’ mean ( L ) , if reduction = ’mean’ sum ( L ) , if reduction = ’sum’ \ell(x, y)=\left\{\begin{array}{ll}\operatorname L, & \text { if reduction }=\text { 'none' } \\ \operatorname{mean}(L), & \text { if reduction }=\text { 'mean' } \\ \operatorname{sum}(L), & \text { if reduction }=\text { 'sum' }\end{array} \right. ℓ(x,y)=⎩⎨⎧L,mean(L),sum(L), if reduction = ’none’ if reduction = ’mean’ if reduction = ’sum’
log_input
对应与输入是否进行对数化。
full
表示loss计算是否保留
l
o
g
(
y
n
!
)
log(y_{n}!)
log(yn!)。 如果保留使用
- 当 y n ≤ 1 y_{n}\leq 1 yn≤1, l o g ( y n ! ) log(y_{n}!) log(yn!) 近似为0。
- 当 y n > 1 y_{n}>1 yn>1,使用斯特林公式(Stirling’s formula), l o g ( y n ! ) log(y_{n}!) log(yn!) 近似为 y n ∗ l o g ( y n ) − y n + 0.5 ∗ l o g ( 2 π y n ) . y_{n}∗log(y_{n})−y_{n}+0.5∗log(2\pi y_{n}). yn∗log(yn)−yn+0.5∗log(2πyn).
eps
是为了防止
x
n
=
=
0
x_{n}==0
xn==0时,
l
o
g
x
n
logx_{n}
logxn计算出错
代码示例:
import torch
import torch.nn as nn
import math
def validate_loss(output, target, flag, full, eps=1e-08):
val = 0
for li_x, li_y in zip(output, target):
for i, xy in enumerate(zip(li_x, li_y)):
x, y = xy
if flag:
loss_val = math.exp(x) - y * x
if full:
if y <= 1:
loss_val = math.exp(x) - y * x + 0
else:
loss_val = math.exp(x) - y * x + \
y * math.log(y) - y + 0.5 * math.log(2 * math.pi * y)
else:
loss_val = x - y * math.log(x + eps)
if full:
if y <= 1:
loss_val = x - y * math.log(x + eps) + 0
else:
loss_val = x - y * math.log(x + eps) + \
y * math.log(y) - y + 0.5 * math.log(2 * math.pi * y)
val += loss_val
return val / output.nelement()
log_input = True
full = True
loss = nn.PoissonNLLLoss(log_input=log_input, full=full)
input_src = torch.Tensor([[0.8, 0.9, 0.3],
[0.8, 0.9, 0.3],
[0.8, 0.9, 0.3],
[0.8, 0.9, 0.3]])
target = torch.Tensor([[1, 3, 5], [1, 0, 6], [1, 4, 5], [1, 1, 7]])
print(input_src.size())
print(target.size())
output = loss(input_src, target)
print(output.item())
# 验证
validateloss = validate_loss(input_src, target, log_input, full)
print(validateloss.item())
# none
loss = nn.PoissonNLLLoss(log_input=log_input, full=full, reduction="none")
output = loss(input_src, target)
print(output)
结果输出:
torch.Size([4, 3])
torch.Size([4, 3])
3.0318071842193604
3.0318076610565186
tensor([[1.4255, 1.5237, 4.6207],
[1.4255, 2.4596, 6.1152],
[1.4255, 2.0169, 4.6207],
[1.4255, 1.5596, 7.7631]])
GaussianNLLLoss
真实标签服从高斯分布的负对数似然损失,神经网络的输出作为高斯分布的均值和方差。
对于包含 N N N个样本的batch数据 D ( x , v a r , y ) D(x, var, y) D(x,var,y), x x x神经网络的输出,作为高斯分布的均值, v a r var var神经网络的输出,作为高斯分布的方差, y y y是样本对应的标签,服从高斯分布。 x x x与 y y y的维度相同, v a r var var和 x x x的维度相同,或者最后一个维度不同且最后一个维度为1,可以进行broadcast。
服从高斯分布的标签对应的概率是积分的形式(不懂如何推导,望指教)。 这里仅仅给出结论,具体可参考论文Estimating the mean and variance of the target probability distribution
第 n n n个样本对应的损失 l n l_{n} ln 为:
l n = 0.5 ∗ ( l o g ( m a x ( v a r n , e p s ) ) + ( x n − y n ) m a x ( v a r n , e p s ) ) l_{n}=0.5*\left(log(max(var_{n},eps)) + \frac{(x_{n}-y_{n})}{max(var_{n},eps)}\right) ln=0.5∗(log(max(varn,eps))+max(varn,eps)(xn−yn))
e p s eps eps是为了防止 v a r n var_{n} varn为0
代码示例:
import torch
import torch.nn.functional as F
import torch.nn as nn
import math
torch.manual_seed(20)
loss = nn.GaussianNLLLoss(reduction='mean')
input = torch.randn(5, 2, requires_grad=True)
# 高斯分布的均值,神经网络输出
var = torch.ones(5, 2, requires_grad=True) # 序列中标签对应的方差不同
# 高斯分布的方差,神经网络的输出
target = torch.randn(5, 2)
output = loss(input, target, var)
print(output.item())
var = torch.ones(5, 1, requires_grad=True) # 序列中标签对应的方差相同
output = loss(input, target, var)
print(output.item())
var = torch.ones(5, requires_grad=True) # 序列中标签对应的方差相同
output = loss(input, target, var)
print(output.item())
loss = nn.GaussianNLLLoss(reduction='none')
var = torch.ones(5, requires_grad=True) # 序列中标签对应的方差相同
output = loss(input, target, var)
print(output)
结果输出:
2.7238247394561768
2.7238247394561768
2.7238247394561768
tensor([1.5049, 2.6215, 1.1505, 6.3066, 2.0357], grad_fn=<MulBackward0>)
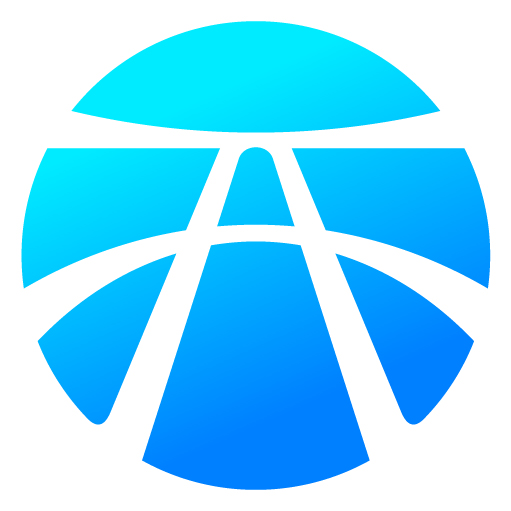
开放原子开发者工作坊旨在鼓励更多人参与开源活动,与志同道合的开发者们相互交流开发经验、分享开发心得、获取前沿技术趋势。工作坊有多种形式的开发者活动,如meetup、训练营等,主打技术交流,干货满满,真诚地邀请各位开发者共同参与!
更多推荐
所有评论(0)