TVM中的auto-scheduling机制(Ansor)学习笔记
背景TVM沿用了Halide中算法的计算与调度分离的思想。用户使用Tensor Expression(TE)这种DSL定义计算,然后编译器优化相应的schedule,最后生成目标平台的代码。因此,要根据给定的计算自动产生高性能的算子实现,其核心就是找到好的schedule。然而这个schedule不仅和计算相关,还与硬件平台相关。这个搜索空间很大,本质上是个np-complete的组合优化问题。因
背景
TVM继承了Halide中算法(Algorithm)与调度(Schedule)分离的思想。用户使用TE(Tensor expression)这种DSL定义计算(算法),然后编译器优化相应的schedule,最后根据schedule生成目标平台的代码。要根据给定的计算自动产生高性能的算子实现,其核心就是找到好的schedule。然而这个schedule和计算、输入相关,还与硬件平台相关。要找到最优的schedule,搜索空间非常大。本质上是个NP-hard的组合优化问题。为了解决这个问题,TVM引入了auto-tuning机制,它使得编译出的算子kernel在性能上有了很大的提升。最早一代的auto-tuning机制称为AutoTVM。相应论文为《Learning to Optimize Tensor Programs》。它需要用户写schedule的template,其中留出一些可供tuning的参数。但写schedule template仍需要一些领域知识和时间成本。因此,TVM中引入了auto-tuning 2.0,即Ansor(AutoScheduler, AutoTVM 2.0)。它对应的论文是《Ansor : Generating High-Performance Tensor Programs for Deep Learning》。与上一代AutoTVM相比,Ansor的主要优点之一是无需写schedule template,从而使整个schedule的生成可以更加地自动化。官网博客《Introducing TVM Auto-scheduler (a.k.a. Ansor)》的Table 1很好地展示了它的特点以及与前一代的区别。整个搜索过程可参见官方博客的Figure 1。论文配套PPT在《Ansor : Generating High-Performance Tensor Programs for Deep Learning》。本文主要是对该机制实现进行学习的一些琐碎的笔记。下面结合代码了解一下它的实现过程。
工作流程
官网上有一些Ansor使用的例子(可参见《Use AutoScheduler for Template-Free Scheduling》)。如针对Nvidia GPU平台的例子:
- 针对单个卷积:Auto-scheduling a Convolution Layer for GPU,对应的代码在
tune_conv2d_layer_cuda.py
。 - 针对整个网络:Auto-scheduling a Neural Network for NVIDIA GPU,对应的代码在
tune_network_cuda.py
。
定义计算
首先需要先定义计算。对于单个算子,可以先使用register_workload()
函数(定义在workload_registry.py
文件中)注册计算任务。如在tune_conv2d_layer_cuda.py
文件中的:
@auto_scheduler.register_workload
def conv2d_layer(N, H, W, CO, CI, KH, KW, stride, padding):
data = te.placeholder((N, CI, H, W), name="data")
kernel = te.placeholder((CO, CI, KH, KW), name="kernel")
bias = te.placeholder((1, CO, 1, 1), name="bias")
conv = topi.nn.conv2d_nchw(data, kernel, stride, padding, dilation=1, out_dtype="float32")
out = topi.nn.relu(conv + bias)
return [data, kernel, bias, out]
这个decorator的主要作用是将定义的函数存在WORKLOAD_FUNC_REGISTRY
这个映射中。然后创建相应的搜索任务:
N, H, W, CO, CI, KH, KW, strides, padding = 1, 7, 7, 512, 512, 3, 3, (1, 1), (1, 1)
task = auto_scheduler.SearchTask(
func=conv2d_layer, args=(N, H, W, CO, CI, KH, KW, strides, padding), target=target
)
其中SearchTask()
函数定义在search_task.py
文件中,它在C++
层对应的同名对象定义在search_task.*
文件中。该对象构建时会先根据workload构建ComputeDAG
对象。ComputeDAG
是一种专门为Ansor设计的IR。它是由TE描述的计算声明(可以是单个算子或一个子图)转换而来。ComputeDAG
的Python和C++
层对象定义在compute_dag.*
文件中。以matmul和conv2d为例,它们的ComputeDAG
为:
# matmul
A = PLACEHOLDER [512, 512]
B = PLACEHOLDER [512, 512]
C(i, j) += (A[i, k]*B[k, j])
# conv2d
data = PLACEHOLDER [1, 512, 7, 7]
pad_temp(i0, i1, i2, i3) = tir.if_then_else(((((i2 >= 1) && (i2 < 8)) && (i3 >= 1)) && (i3 < 8)), data[i0, i1, (i2 - 1), (i3 - 1)], 0f)
kernel = PLACEHOLDER [512, 512, 3, 3]
compute(nn, ff, yy, xx) += (pad_temp[nn, rc, (yy + ry), (xx + rx)]*kernel[ff, rc, ry, rx])
bias = PLACEHOLDER [1, 512, 1, 1]
T_add(ax0, ax1, ax2, ax3) = (compute[ax0, ax1, ax2, ax3] + bias[ax0, ax1, 0, 0])
compute(i0, i1, i2, i3) = max(T_add[i0, i1, i2, i3], 0f)
和AutoTVM中在预定参数中搜索不同,Ansor通过修改循环结构来建构搜索空间。而为了灵活地变更循环结构,它基于原来的IR实现了一个轻量的循环结构IR,专用于schedule的搜索。它包含状态(State)和动作(Action)。前者代表schedule搜索的状态,即schedule定义的循环结构;后者包含一或多个schedule原语。至于为啥不同已有的IR,而是扩展一套Sketch IR,社区里有讨论。大体是为了循环结构的快速增量修改、transform历史可追溯等。ComputeDAG
实现了一些分析过程,如总浮点运算、消费/生产者关系和stage是否该被tile等。
如果是要tuning的对象是整个网络,则需要调用extract_tasks()
函数进行任务的抽取。它会从一个Relay表示的网络中抽取tuning任务。
# Extract tasks from the network
print("Extract tasks...")
mod, params, input_shape, output_shape = get_network(network, batch_size, layout, dtype=dtype)
tasks, task_weights = auto_scheduler.extract_tasks(mod["main"], params, target)
for idx, task in enumerate(tasks):
print("========== Task %d (workload key: %s) ==========" % (idx, task.workload_key))
print(task.compute_dag)
比如对于ResNet50网络模型,其输出为(部分):
========== Task 0 (workload key: ["dac19035dd5fe9424ee8617421b9c817", 1, 28, 28, 128, 4, 4, 128, 128, 1, 28, 28, 128, 1, 28, 28, 128]) ==========
placeholder = PLACEHOLDER [1, 28, 28, 128]
data_pad(i0, i1, i2, i3) = tir.if_then_else(((((i1 >= 1) && (i1 < 29)) && (i2 >= 1)) && (i2 < 29)), placeholder[i0, (i1 - 1), (i2 - 1), i3], 0f)
input_tile(eps, nu, p, ci) = data_pad[floordiv(p, 196), ((floormod(floordiv(p, 14), 14)*2) + eps), ((floormod(p, 14)*2) + nu), ci]
B(i, j) = select(((floormod(i, 4) == 3) && (floormod(j, 4) == 3)), 1f, select(((floormod(i, 4) == 3) && (floormod(j, 4) == 2)), ..(OMITTED).. ormod(i, 4) == 0) && (floormod(j, 4) == 1)), 0f, select(((floormod(i, 4) == 0) && (floormod(j, 4) == 0)), 1f, 0f))))))))))))))))
data_pack(eps, nu, p, ci) += ((input_tile[r_a, r_b, p, ci]*B[r_a, eps])*B[r_b, nu])
placeholder = PLACEHOLDER [4, 4, 128, 128]
bgemm(eps, nu, p, co) += (data_pack[eps, nu, p, ci]*placeholder[eps, nu, co, ci])
A(i, j) = select(((floormod(i, 4) == 3) && (floormod(j, 2) == 1)), 1f, select(((floormod(i, 4) == 3) && (floormod(j, 2) == 0)), ..(OMITTED).. ct(((floormod(i, 4) == 0) && (floormod(j, 2) == 1)), 0f, select(((floormod(i, 4) == 0) && (floormod(j, 2) == 0)), 1f, 0f))))))))
inverse(vh, vw, p, co) += ((bgemm[r_a, r_b, p, co]*A[r_a, vh])*A[r_b, vw])
conv2d_winograd(n, h, w, co) = inverse[floormod(h, 2), floormod(w, 2), ((((n*14)*14) + (floordiv(h, 2)*14)) + floordiv(w, 2)), co]
placeholder = PLACEHOLDER [1, 28, 28, 128]
T_add(ax0, ax1, ax2, ax3) = (conv2d_winograd[ax0, ax1, ax2, ax3] + placeholder[ax0, ax1, ax2, ax3])
========== Task 1 (workload key: ["7006235cfc29b73be524cf390ed5a977", 1, 56, 56, 64, 1, 1, 64, 64, 1, 56, 56, 64]) ==========
placeholder = PLACEHOLDER [1, 56, 56, 64]
PaddedInput(i0, i1, i2, i3) = placeholder[i0, i1, i2, i3]
placeholder = PLACEHOLDER [1, 1, 64, 64]
Conv2dOutput(nn, yy, xx, ff) += (PaddedInput[nn, (yy + ry), (xx + rx), rc]*placeholder[ry, rx, rc, ff])
========== Task 2 (workload key: ["d7b65649a4dd54becea0a52aabbc5af5", 1, 1000, 1, 1000]) ==========
placeholder = PLACEHOLDER [1, 1000]
T_softmax_maxelem(i0) max= placeholder[i0, k]
T_softmax_exp(i0, i1) = tir.exp((placeholder[i0, i1] - T_softmax_maxelem[i0]))
T_softmax_expsum(i0) += T_softmax_exp[i0, k]
T_softmax_norm(i0, i1) = (T_softmax_exp[i0, i1]/T_softmax_expsum[i0])
========== Task 3 (workload key: ["f4380bb1dc62422a69ad4a1a9771f927", 1, 56, 56, 64, 1, 1, 64, 128, 1, 28, 28, 128]) ==========
placeholder = PLACEHOLDER [1, 56, 56, 64]
PaddedInput(i0, i1, i2, i3) = placeholder[i0, i1, i2, i3]
placeholder = PLACEHOLDER [1, 1, 64, 128]
Conv2dOutput(nn, yy, xx, ff) += (PaddedInput[nn, ((yy*2) + ry), ((xx*2) + rx), rc]*placeholder[ry, rx, rc, ff])
其中的extract_tasks()
函数定义在relay_integration.py
文件中。它执行call_all_topi_funcs()
函数,过程中TracingEnvironment
对象会收集TOPI(TVM Operator Inventory)调用。完成后任务的key和权重会记录在TracingEnvironment
对象的wkl_key_to_weight
成员中。对于这个任务和相应的权重,会创建相应的SearchTask
对象。
对于每个CallNode
,会创建ComputeDAG
对象,并将之注册。其中 AccessAnalyzer
会对该计算做静态分析,如对于某个算子,检查它是否是simple access(element-wise),是否需要multi-level tiling,是否是output,是否strictly inlineable等。这些信息主要用于后面的sketch生成。具体实现在AccessAnalyzer::AccessAnalyzer()
函数(在compute_dag.cc
文件中)中。
Tuning
下一步设置tuning的一些参数,然后调用tune()
函数开始tuning过程。相应代码:
def run_tuning():
print("Begin tuning...")
measure_ctx = auto_scheduler.LocalRPCMeasureContext(repeat=1, min_repeat_ms=300, timeout=10)
tuner = auto_scheduler.TaskScheduler(tasks, task_weights)
tune_option = auto_scheduler.TuningOptions(
num_measure_trials=200, # change this to 20000 to achieve the best performance
runner=measure_ctx.runner,
measure_callbacks=[auto_scheduler.RecordToFile(log_file)],
)
tuner.tune(tune_option)
这整个tuning的过程有几个关键组件。其中任务调度器(Task scheduler)负责任务的调度。前面提到,整个网络经过切分后变为多个子图,每个子图对应一个任务。调度器负责调度这些任务使那些最容易提升性能的任务得以优先得到tuning。对于每个任务(子图),sketch generation过程生成sketch。它可以理解为比较粗粒度的调度,其中会确定大体的循环结构。然后annotation过程是细化这个调度。它会随机产生更细粒度的优化 (涉及parallel, unrolling, tiling size等),从而形成完整的schedule。它主要为下一步的搜索提供初始种群。最后,通过进化算法(Evolutionary algorithm)进行搜索。为了减少耗时的性能测试,搜索过程同时会训练cost model用于对生成的程序进行性能评估。根据评估结果选取高分的候选进行性能测试。然后把真实硬件上运行测得的性能数据,喂给cost model训练提升其准确率,以此循环。
Task Scheduler
对于一个神经网络,经过任务抽取会将其切分为许多的子图(如ResNet50网络经过分图后有约30个子图)。如何将时间在这些子图上有效地分配使得性能提升尽可能大便是个问题。官方PPT p20的图形象地说明了它与以往方法的比较。
Ansor中采用的方法是估计每个任务对于目标函数(代表端到端的latency)的影响,然后利用导数选取能使目标函数下降最快的任务 。假设有
n
n
n个任务 ,令
t
=
[
t
1
,
t
2
,
.
.
.
,
t
n
]
t = [t_1, t_2, ..., t_n]
t=[t1,t2,...,tn]为分配向量(Allocation vector),其中
t
i
t_i
ti表示第
i
i
i个任务所花时间。另外令
g
i
(
t
)
g_i(t)
gi(t)为任务
i
i
i对应子图的延迟,它是分配向量
t
t
t的函数。目标函数可写为
f
=
∑
i
=
1
n
w
i
×
g
i
(
t
)
f = \sum_{i=1}^n w_i \times g_i(t)
f=∑i=1nwi×gi(t)。其中
w
i
w_i
wi指第
i
i
i个子图的权重(实现中是该子图的重复次数)。这样,每次选导数小的任务即可。但因为它是离散的,没法直接求导,所以导数的计算需要近似(以下公式来自论文附录部分):
∂
f
∂
t
i
=
∂
f
∂
g
i
∂
g
i
∂
t
i
≈
∂
f
∂
g
i
(
α
g
i
(
t
i
)
−
g
i
(
t
i
−
Δ
t
)
Δ
t
)
+
(
1
−
α
)
(
g
i
(
t
i
+
Δ
t
)
−
g
i
(
t
i
)
Δ
t
)
≈
∂
f
∂
g
i
(
α
g
i
(
t
i
)
−
g
i
(
t
i
−
Δ
t
)
Δ
t
)
+
(
1
−
α
)
(
g
i
(
t
i
+
1
)
−
g
i
(
t
i
)
)
\frac{\partial f}{\partial t_i} = \frac{\partial f}{\partial g_i} \frac{\partial g_i}{\partial t_i} \\ \approx \frac{\partial f}{\partial g_i} (\alpha \frac{g_i(t_i) - g_i(t_i - \Delta t)}{\Delta t}) + (1 - \alpha)( \frac{g_i(t_i + \Delta t) - g_i(t_i)}{\Delta t}) \\ \approx \frac{\partial f}{\partial g_i} (\alpha \frac{g_i(t_i) - g_i(t_i - \Delta t)}{\Delta t}) + (1 - \alpha)( g_i(t_i + 1) - g_i(t_i))
∂ti∂f=∂gi∂f∂ti∂gi≈∂gi∂f(αΔtgi(ti)−gi(ti−Δt))+(1−α)(Δtgi(ti+Δt)−gi(ti))≈∂gi∂f(αΔtgi(ti)−gi(ti−Δt))+(1−α)(gi(ti+1)−gi(ti))
其中 g i ( t i ) g_i(t_i) gi(ti)和 g i ( t i − Δ t ) g_i(t_i - \Delta t) gi(ti−Δt)可以从历史信息获得,但 g i ( t i + 1 ) g_i(t_i + 1) gi(ti+1)不行,因为它是未来的信息,因此需要预测。有两种方法:
- 乐观猜测(Optimistic guess):假设再经过 t i t_i ti时间后 g i g_i gi到0。也是说 g i ( t i + 1 ) = g i ( t i ) − g i ( t i ) / t i g_i(t_i + 1) = g_i(t_i) - g_i(t_i) / t_i gi(ti+1)=gi(ti)−gi(ti)/ti。
- 任务间的相似度:假设如果子图相似,则它们的latency也应该相似。因此可以用相似的子图的latency作为近似。
考虑这两个因素,可以得到以下近似(以下公式来自论文附录):
g
i
(
t
i
+
1
)
≈
min
(
g
i
(
t
i
)
−
g
i
(
t
i
)
t
i
,
β
C
i
max
k
∈
N
(
i
)
V
k
)
g_i(t_i + 1) \approx \min (g_i(t_i) - \frac{g_i(t_i)}{t_i} , \beta \frac{C_i}{\max_{k \in N(i)} V_k})
gi(ti+1)≈min(gi(ti)−tigi(ti),βmaxk∈N(i)VkCi)
其中
C
i
C_i
Ci是任务
i
i
i的浮点操作,
V
k
V_k
Vk是任务
k
k
k每秒浮点操作数。实现中
g
i
(
t
i
)
g_i(t_i)
gi(ti)使用的是任务
i
i
i当前最优latency,
t
i
t_i
ti是对于任务
i
i
i进行tuning的轮数(即调用_tune_task()
函数次数)。
该机制主要实现类为TaskScheduler
(在task_scheeduler.py
文件中)。实现中有两种策略:round-robin和gradient。Round-robin就简单的轮流,而Gradient就是上面提到基于导数方法 。TaskScheduler::tune()
函数主要流程如下:
tune() # In task_scheduler.py
serach_policies = make_search_policies(search_policy, ...)
cost_model = XGBModel(...)
search_policies = [SketchPolicy() for task in tasks]
# do a round robin first to warm up
for idx in range(len(self.tasks)):
_tune_task(idx)
# use the specific strategy to choose workload to tune
while self.ct < tune_option.num_measure_trials and len(self.dead_tasks) < len(self.tasks):
if strategy is round_robin:
...
elif strategy is gradient:
for each task:
# compute gradient from chain rule : (delta f / delta g_i)
...
# compute (g_i(t_i) - g(t_i - \Delta t)) / (\Delta t)
...
# compute (g_i(t_i + \Delta t) - g(t_i)) / (\Delta t)
...
# combine all grads
...
gradients.append(grad)
task_idx = np.argmin(gradient)
_tune_task(task_idx)
search_policies[task_idx].continue_search_one_round()
这里先进行 一轮warm up,即对于每个任务进行一轮tuning。接下来进入主循环,这时开始使用上面提到的基于gradient方式选取任务来进行tuning,每次被选中的任务会被tuning一轮。一轮中trial的次数为num_measures_per_round
,其值为默认值64和num_measure_trials/task size
的最小值(为了让所有的task都至少被tuning一次)。该循环结束的条件为达到指定的measure trials次数,或者所有的任务都“死亡”(“死亡”指其搜索空间被充分探索,或者一段时间没有改进)。
Program Sampler
这一步的目标主要是自动构建搜索空间并在其中均匀采样。采用的方法是两层(sketch + annotation)的层次化搜索空间。其中第一个阶段称为sketch generation,这一步产生的sketch数量较少,第二个阶段称为random annotation,其数量相对多一些。官方PPT p12开始有它的图示介绍。
Sketch生成针对一个子图 。上面提到的continue_search_one_round()
函数用于执行一轮搜索。主要流程如下:
continue_search_one_round() // In search_policy.py
SketchPolicy::ContinueSearchOneRound() // In sketch_policy.cc
// Search one round to get promising states
best_states = SearchOneRound()
// 1. Generate sketches
sketch_cache_ = GenerateSketches()
// 2. Sample the init population
init_population = SampleInitPopulation(sketch_cache_)
// 3. Perform evolutionary search
// Also insert already measured good states to the initial population
init_population.push_back(...)
// Sample some random states for eps-greedy
RandomSampleStates(init_population, ...)
return EvolutionarySearch(...)
// Pick 'num_measure_per_iter' states to measure, also pick som e random states
inputs = PickStatesWithEpsGreedy(best_states, random_states, num_measures)
// Measure candiate states
results = ProgramMeasurer::Measure(search_task, ..., inputs)
// Update the cost model
CostModel::Update()
update_func()
update() // In xgb_model.py
其中SketchPolicyNode::GenerateSketches()
函数(实现在sketch_policy.cc
文件中)用于产生sketch。它以ComputeDAG
中的初始状态为起点,对于该状态中的每一个Stage
,尝试应用推导规则。如果能应用,则应用后加入列表等待下一步的推导。这是一个枚举遍历的过程。实现中的State
(实现在loop_state.*
文件中)包含当前循环结构和于构建它的变换步骤(Transformation step)的列表。每个State
对应ComputeDAG
中一个特定的schedule。这个枚举过程示意图如下:
关于推导规则,可以参见SketchPolicy::SketchPolicy()
函数,它之中会初始化推导规则列表(SketchPolicyNode
中的sketch_rules
)。注意这个规则列表是平台相关的。推导规则在论文中的Table 1中有比较详细的描述。对应地,代码中相应部分在sketch_policy.cc
文件中:
/********** Sketch generation rules **********/
static RuleSkipStage rule_skip_stage;
static RuleAlwaysInline rule_always_inline;
static RuleMultiLevelTiling rule_multi_level_tiling;
static RuleMultiLevelTilingWithFusion rule_multi_level_tiling_with_fusion;
static RuleAddCacheRead rule_add_cache_read_stage;
static RuleAddCacheWrite rule_add_cache_write_stage;
static RuleAddRfactor rule_add_rfactor;
static RuleCrossThreadReduction rule_cross_thread_reduction;
static RuleSimplifyComputeWithConstTensor rule_simplify_compute_with_const_tensor;
static RuleSpecialComputeLocationGPU rule_special_compute_location_gpu;
对于每条规则,需要实现两个函数,一是判断是否要应用该规则的条件的函数MeetCondition()
;另一个是应用该规则的函数Apply()
。在是否要应用规则的判断中,使用了之前构建ComputeDAG
时AccessAnalyzer
中的信息。这些规则包含以下这一些:
- RuleSkipState:忽略,就是啥事都不干。
- RuleAlwaysInline:简单的element-wise操作,如element-wise add, relu等。
- RuleMultiLevelTiling:有算子内部重用机会的计算密集算子,如matmul, conv2d。
- RuleMultiLevelTilingWithFusion:符合上面条件且数据的使用者可以被融合,如conv2d + relu。
- RuleAddCacheRead:数据使用者是需要多层tiling的操作。
- RuleAddCacheWrite:操作需要多层tiling,数据使用者不能被融合。
- RuleAddRfactor:Reduce轴拆分。用于在space axis短而reduce axis长的情况下。
- RuleCrossThreadReduction:将上面拆分后的reduce轴通过多个线程并行化。
- RuleSimplifyComputeWithConstTensor:主要用于卷积的Winograd算法。
- RuleSpecialComputeLocationGPU:和上面类似,主要用于GPU上的Winograd算法。
对于multi-level tiling这种经典的变换模式有固定的模式。CPU上是"SSRSRS"的结构,GPU上是"SSSRRSRS"的结构。其中的"S"代表one tile level of space loops,"R"代表one tile level of reduction loops。它适用于计算密集型的算子(如matmul, conv2d, conv3d)。
为了应用进化算法,至少需要一个初始种群,该种群中个体都需要是完整的schedule。上一步中,虽然生成了循环结构,但是一些细节还没有确定,如tile的size,GPU thread bind,循环的unroll, vectorize, parallel等。因此,接下来需要随机加上这些信息,使之完整。这一步称为annotation。相关例子可参见官方PPT p15。
SketchPolicyNode::SampleInitPopulation()
函数(实现在sketch_policy.cc
文件中)实现了通过随机的方法来产生annotation的机制。它先从给定的sketch随机选一个,然后随机做一些修改,如填tile size,将outer loop并行化,内部循环向量化,和循环展开一些inner loop,从而生成schedule。当生成的schedule数量达到指定的数量时停止(默认为50)。代码中的init_rules
中存有生成annotation的规则。代码中对应声明如下:
/********** Init population rules **********/
static InitFillTileSize init_fill_tile_size;
static InitChangeComputeLocation init_change_compute_location;
static InitParallel init_parallel;
static InitUnroll init_unroll;
static InitVectorization init_vectorization;
static InitThreadBind init_thread_bind;
以InitFillTileSize
为例,它的Apply()
函数扫描变换历史,然后对其中所有的SplitStep
随机填tile size。
Performance Tuner
随机采样产生的样本数较少,无法保证得到好的性能。因此需要在采样出来的程序上采用结合cost model的进化算法进行tuning。产生了初始种群后,就可以通过进化算法进行搜索了。这部分主要实现在SketchPolicyNode::EvolutionarySearch()
函数(在sketch_policy.cc
文件中)中。进化算法中多轮重复利用mutation机制产生候选集合,输出一些由cost model判定score最高的程序。这些程序会被编译和运行在目标机器上,得到真实的运行速度数据。这个数据被喂给cost model进行训练。因此,cost model的准确率会随着数据的增多逐渐上升。
根据进化算法的一般套路,其中有两个比较重要的步骤:
-
Crossover
通过融合两个或多个parent的基因来产生新的后代。可参见官方PPT p17。这块目前在代码中貌似还没有实现。 -
Mutation
这一步主要应用以下规则:- Tile size:随机挑选一个tiled loop,将一个tile level中的tile size除以随机的因子,再将这个因子乘到另一个tile level上。
- Parallel/unroll/vectorize factor and granularity:随机选取一个标为parallel的loop,通过split它或者融合相邻的loop level改变并行粒度。随机挑选循环展开的最大步数。
- Computation location:随机选择一个非multi-level tiled的节点,随机将它的computation location改到另一个合法的attach point上。
这些规则对应的代码在sketch_policy_rules.h
中:
/*! \brief The rule that mutates tile size by randomly dividing a tile size by a factor
and multipling it to another tile size. */
DEFINE_MUTATE_POPULATION_RULE(MutateTileSize);
/*! \brief The rule that mutates the number of fused outer iterators annotated by parallel. */
DEFINE_MUTATE_POPULATION_RULE(MutateParallel);
/*! \brief The rule that randomly changes the computation location for some stages that do not
* need tiling and are not strictly inlineable(e.g. data padding). */
DEFINE_MUTATE_POPULATION_RULE(MutateComputeLocation);
/*! \brief The rule that mutates the value of a randomly selected auto unroll pragma step. */
DEFINE_MUTATE_POPULATION_RULE(MutateAutoUnroll);
整个进化算法搜索过程示意图如下:
整个迭代默认有5轮,每一轮中会根据上一轮的种群(第一轮时使用前面生成的初始种群)通过mutation的规则生成新的种群。对于上一轮的种群使用cost model对其性能进行预测,得到它们的得分。得分越大代表性能可能越好。因此,得分越大,该个体被选中用来mutation的几率也越大。另外,整个过程中维护一个数量有上限的heap,它存有目前为止预测性能最好的一些个体。经过几轮迭代后,该heap中即存有几轮进化后“优胜”的个体,其它的就被“劣汰”了。这些个体随后会在真实硬件上进行性能的测试。得到的数据用来训练cost model。于是,cost model会变得更加精确,然后又被用于后面的搜索。
如上描述,在候选的挑选过程中,进化算法会使用cost model预测性能。具体地,cost model用于预测每个innermost non-loop statement的score。示意图可参见官方PPT p18。它提取每个innermost non-loop statement的特征(浮点、整数操作数量、向量化、循环展开、并行化、GPU线程绑定相关特征、计算密度、buffer访问特征、内在分配特征和其它)。具体地,它将这些特征编码到固定长的特征向量。模型基于性能测试的数据进行训练。默认使用的模型是XGBModel,对应的代码在xgb_modelpy
中。由于XGBoost不支持增量训练,所以每次都会拿所有数据重新训练一把。它的update()
函数用于训练,predict()
函数用于预测。特征提取部分实现在feature.cc
文件中,主要是在GetPerStoreFeaturesFromStates()
函数中。
应用搜索结果
当tuning过程完成后,搜索的历史记录在log文件中。接下来就是应用搜索历史中最优的配置生成schedule。
# Apply the best schedule
sch, args = task.apply_best(log_file)
然后调用lower和build最终生成目标代码。这块没有什么特殊的,和以往是一样的:
print(tvm.lower(sch, args, simple_mode=True))
func = tvm.build(sch, args, target)
结语
与TVM中原auto-tuning机制AutoTVM相比,Ansor的优势主要在于几点:
- 更好的易用性:它将很多专家经验以规则的形式实现在代码中,使得开发者不用手写schedule template,从而实现了自动化,降低了使用门槛。
- 更优的schedule:通过sketch加annotation这样的层次化搜索设计扩大了搜索空间,从而可能搜索到性能更好的schedule。
- 更高效的搜索:通过进化算法(AutoTVM中使用的是模拟退火)和基于机器学习的cost model使搜索更高效。另外,它还通过更智能的任务调度(这部分AutoTVM中没有)使得性能收益更大的子图任务得到更充分的优化。通过这些技术,与AutoTVM相比,Ansor的搜索时间已成倍地减少。但由于要探索的空间仍然很大,进一步减少搜索时间仍是一个值得研究的方向。
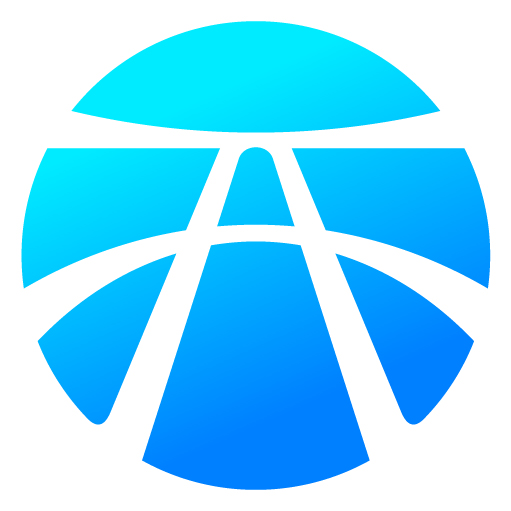
开放原子开发者工作坊旨在鼓励更多人参与开源活动,与志同道合的开发者们相互交流开发经验、分享开发心得、获取前沿技术趋势。工作坊有多种形式的开发者活动,如meetup、训练营等,主打技术交流,干货满满,真诚地邀请各位开发者共同参与!
更多推荐
所有评论(0)