clickhouse 基本数据类型
clickhouse有数值类型(整形,浮点数,定点数),字符串类型,日期时间类型,还有一些特别的数据类型。没有Boolean类型,但是可以用整形的0和1替代。使用UInt即可。0.支持的数据类型::) select * from system.data_type_families ;┌─name────────────────────┬─case_insensitive─┬─alias_to───
clickhouse有数值类型(整形,浮点数,定点数),字符串类型,日期时间类型,还有一些特别的数据类型。
没有Boolean类型,但是可以用整形的0和1替代。使用UInt即可。
0.支持的数据类型:
:) select * from system.data_type_families ;
┌─name────────────────────┬─case_insensitive─┬─alias_to────┐
│ IPv6 │ 0 │ │
│ IPv4 │ 0 │ │
│ IntervalYear │ 0 │ │
│ IntervalQuarter │ 0 │ │
│ IntervalMonth │ 0 │ │
│ IntervalDay │ 0 │ │
│ IntervalHour │ 0 │ │
│ IntervalSecond │ 0 │ │
│ AggregateFunction │ 0 │ │
│ Nothing │ 0 │ │
│ Tuple │ 0 │ │
│ Array │ 0 │ │
│ Nullable │ 0 │ │
│ Int32 │ 0 │ │
│ Date │ 1 │ │
│ Enum │ 0 │ │
│ Enum8 │ 0 │ │
│ IntervalMinute │ 0 │ │
│ FixedString │ 0 │ │
│ LowCardinality │ 0 │ │
│ String │ 0 │ │
│ DateTime │ 1 │ │
│ UUID │ 0 │ │
│ Decimal64 │ 1 │ │
│ Decimal32 │ 1 │ │
│ Float64 │ 0 │ │
│ Int16 │ 0 │ │
│ DateTime64 │ 1 │ │
│ Decimal128 │ 1 │ │
│ Int8 │ 0 │ │
│ SimpleAggregateFunction │ 0 │ │
│ Nested │ 0 │ │
│ Int64 │ 0 │ │
│ Decimal │ 1 │ │
│ IntervalWeek │ 0 │ │
│ UInt64 │ 0 │ │
│ Enum16 │ 0 │ │
│ UInt32 │ 0 │ │
│ UInt16 │ 0 │ │
│ Float32 │ 0 │ │
│ UInt8 │ 0 │ │
│ BINARY │ 1 │ FixedString │
│ LONGBLOB │ 1 │ String │
│ LONGTEXT │ 1 │ String │
│ TINYTEXT │ 1 │ String │
│ TEXT │ 1 │ String │
│ TINYINT │ 1 │ Int8 │
│ DEC │ 1 │ Decimal │
│ VARCHAR │ 1 │ String │
│ MEDIUMBLOB │ 1 │ String │
│ TIMESTAMP │ 1 │ DateTime │
│ BLOB │ 1 │ String │
│ FLOAT │ 1 │ Float32 │
│ INTEGER │ 1 │ Int32 │
│ DOUBLE │ 1 │ Float64 │
│ BIGINT │ 1 │ Int64 │
│ TINYBLOB │ 1 │ String │
│ CHAR │ 1 │ String │
│ MEDIUMTEXT │ 1 │ String │
│ INT │ 1 │ Int32 │
│ SMALLINT │ 1 │ Int16 │
└─────────────────────────┴──────────────────┴─────────────┘
61 rows in set. Elapsed: 0.002 sec.
hadoop101 :) select * from system.data_type_families where case_insensitive=1;
┌─name───────┬─case_insensitive─┬─alias_to────┐
│ Date │ 1 │ │
│ DateTime │ 1 │ │
│ Decimal64 │ 1 │ │
│ Decimal32 │ 1 │ │
│ DateTime64 │ 1 │ │
│ Decimal128 │ 1 │ │
│ Decimal │ 1 │ │
│ BINARY │ 1 │ FixedString │
│ LONGBLOB │ 1 │ String │
│ LONGTEXT │ 1 │ String │
│ TINYTEXT │ 1 │ String │
│ TEXT │ 1 │ String │
│ TINYINT │ 1 │ Int8 │
│ DEC │ 1 │ Decimal │
│ VARCHAR │ 1 │ String │
│ MEDIUMBLOB │ 1 │ String │
│ TIMESTAMP │ 1 │ DateTime │
│ BLOB │ 1 │ String │
│ FLOAT │ 1 │ Float32 │
│ INTEGER │ 1 │ Int32 │
│ DOUBLE │ 1 │ Float64 │
│ BIGINT │ 1 │ Int64 │
│ TINYBLOB │ 1 │ String │
│ CHAR │ 1 │ String │
│ MEDIUMTEXT │ 1 │ String │
│ INT │ 1 │ Int32 │
│ SMALLINT │ 1 │ Int16 │
└────────────┴──────────────────┴─────────────┘
27 rows in set. Elapsed: 0.015 sec.
可以看到支持61中数据类型,有20种数据类型是为了兼容其他数据库的类型,主要是兼容MySQL的数据类型。
case_insensitive 选项为1 表示大小写不敏感,字段类型不区分大小写
为0 表示大小写敏感,即字段类型需要严格区分大小写。
1.整形
Clickhouse是使用C和C++类型写的,数据类型和C语言的类型息息相关。
1.1 Int
整形区分为有符号和无符号类型的整数。
有符号类型:整型范围(-2^n-1~2^n-1 -1): 8位=1字节
MySQL | Hive | Clickhouse | 大小(字节) | 数据范围 |
tinyint | tinyint | Int8 | 1 | [-128 : 127] |
smallint | smallint | Int16 | 2 | [-32768 : 32767] |
int | int | Int32 | 3 | [-2147483648 : 2147483647] |
bigint | bigint | Int64 | 4 | [-9223372036854775808 : 9223372036854775807] |
无符号类型:
无符号类型:整形范围0~2^n-1
MySQL | Hive | Clickhouse | 大小(字节) | 数据范围 |
Tinyint unsigned |
| UInt8 | 1 | [0 : 255] |
smallint unsigned |
| UInt16 | 2 | [0 : 65535] |
Int unsigned |
| UInt32 | 3 | [0 : 4294967295] |
Bigint unsigned |
| UInt64 | 4 | [0 : 18446744073709551615] |
1.2 Float
MySQL | Clickhouse | 大小字节 | 有效精度(位数) |
float | Float32 | 4 | 7 |
double | Flout64 | 8 | 16 |
clickhouse 直接使用Float32代表单精度浮点数 使用Float64表示双精度浮点数。
使用浮点数需要注意它的精度是有限的,Float32从小数点后第8位,Float64从小数点后的第17位起会产生数据溢出。
hadoop101 :) select toFloat32('0.12345678901234567890') as a,toTypeName(a);
┌──────────a─┬─toTypeName(toFloat32('0.12345678901234567890'))─┐
│ 0.12345679 │ Float32 │
└────────────┴─────────────────────────────────────────────────┘
1 rows in set. Elapsed: 0.014 sec.
hadoop101 :) select toFloat64('0.12345678901234567890') as a,toTypeName(a);
┌───────────────────a─┬─toTypeName(toFloat64('0.12345678901234567890'))─┐
│ 0.12345678901234568 │ Float64 │
└─────────────────────┴─────────────────────────────────────────────────┘
1 rows in set. Elapsed: 0.012 sec.
hadoop101 :) select 0.123456789123456789+0.987654321987654321 as b;
SELECT 0.12345678912345678 + 0.9876543219876543 AS b
┌──────────────────b─┐
│ 1.1111111111111112 │
└────────────────────┘
1 rows in set. Elapsed: 0.002 sec.
hadoop101 :) select 0.123456789+0.987654321 as a;
SELECT 0.123456789 + 0.987654321 AS a
┌──────────a─┐
│ 1.11111111 │
└────────────┘
clickhouse的浮点数支持正无穷,负无穷和非数字的表达方式:
select 1.0/0,-1/0,0/0;
┌─divide(1., 0)─┬─divide(-1, 0)─┬─divide(0, 0)─┐
│ inf │ -inf │ nan │
└───────────────┴───────────────┴──────────────┘
inf表示英文单词infinity NaN 表示not-a-number
使用浮点数进行数据运算会数据数据计算不准确的情形:
结论:Float32 和Float64 在数据精度较高的计算中会存在数据精度丢失,使用需谨慎。
1.3 Decimal
若需要要求更高的精度的数值运算,则需要使用定点数。Clickhouse提供了Decimal32,Decimal64,Decimal128三种精度的定点数。简写为Decimal32(S),Decimal64(S),Decimal128(S),原生方式为Decimal(P,S):
其中P表示精度precision,决定总位数(整数部分+小数位部分),取值范围为1--38
S代表规模 scale,决定小数位,取值范围是0--P。
简写方式和原生方式的对应表:
Decimal类型:
名称 | 等效声明 | 数据范围 |
Decimal32(S) | Decimal(1-9,S) | - ( -1 * 10^(9 - S), 1 * 10^(9 - S) ) |
Decimal64(S) | Decimal(10-18,S) | - ( -1 * 10^(18 - S), 1 * 10^(18 - S) ) |
Decimal128(S) | Decimal(19-38,S) | - ( -1 * 10^(38 - S), 1 * 10^(38 - S) ) |
MySQL Clickhouse
Decimal(9,2) Decimal32(2)
Decimal(22,6) Decimal128(6)
Decimal32(4) 表示的数据类型等同于MySQL的decimal(9,4)
数据范围为 -99999.9999 to 99999.9999 最小精度为0.0001
由于现代计算机只支持32bit和64bit,Decimal128在软件层面是由软件模拟实现,速度要比Decimal32和Decimal64慢。
在适用不同精度的定点数进行四则运算的时候小数位会发生变化:
四则运算规则:
运算名称 | 规则 |
加法 | S=max(S1,S2) |
减法 | S=max(S1,S2) |
乘法 | S=S1+S2(S1>=S2) |
除法 | S=S1(S为分子,被除数,S1/S2) |
Decimal类型Overflow
1.类型越界
2.类型不同
SELECT toDecimal32(1, 8) < 100
DB::Exception: Can't compare.
SET decimal_check_overflow = 0
SELECT toDecimal32(1, 8) < 100
┌─less(toDecimal32(1, 8), 100)─┐
│ 1 │
└──────────────────────────────┘
decimal_check_overflow 参数默认开启 参数值为1.
SELECT toDecimal32(4.2, 8) AS x, 6 * x
DB::Exception: Decimal math overflow.
SET decimal_check_overflow = 0;
hadoop101 :) SELECT toDecimal32(4.2, 8) AS x, 6 * x
┌──────────x─┬─multiply(6, toDecimal32(4.2, 8))─┐
│ 4.20000000 │ -17.74967296 │
└────────────┴──────────────────────────────────┘
可以看到数值不对
hadoop101 :) SELECT toDecimal64(4.2, 8) AS x, 6 * x
SELECT
toDecimal64(4.2, 8) AS x,
6 * x
┌──────────x─┬─multiply(6, toDecimal64(4.2, 8))─┐
│ 4.20000000 │ 25.20000000 │
└────────────┴──────────────────────────────────┘
2.字符串类型:
2.1 String
String表示字符串类型,长度不限。在声明String类型的时候无须声明字符串的大小。String类型不限定字符集,理论上可以将任意编码的字符串存进来。但是为了规范和统一,推荐使用UTF-8字符集。
2.2FixedString
和MySQL的CHAR比较类似,对于有明确长度的字符串可以使用。
和char不同的是FixedString使用Null字节填充末尾字符,而char使用空格填充。
hadoop101 :) select toFixedString('wuhan',10) as wh ,length(wh) as length;
┌─wh────┬─length─┐
│ wuhan │ 10 │
└───────┴────────┘
hadoop101 :) select toFixedString('wuhan',10) as wh ,substring(wh,1,5) string,substring(wh,6,10) rest;
┌─wh────┬─string─┬─rest─┐
│ wuhan │ wuhan │ │
└───────┴────────┴──────┘
2.3 UUID
UUID和MySQL中的UUID类似,格式为8-4-4-4-12
若一个UUID类型的字段在写入数据时候没有被赋值则按照格式使用0填充。
hadoop101 :) create table uuid(pkey UUID,name varchar ) ENGINE = Memory;
insert into uuid select generateUUIDv4(),'China';
insert into uuid(name) select 'WUHAN';
-- 查询
hadoop101 :) select pkey,name from uuid;
┌─────────────────────────────────pkey─┬─name──┐
│ 8df34966-a69d-4d1c-9936-5229d515f5f2 │ China │
└──────────────────────────────────────┴───────┘
┌─────────────────────────────────pkey─┬─name──┐
│ 00000000-0000-0000-0000-000000000000 │ WUHAN │
└──────────────────────────────────────┴───────┘
2 rows in set. Elapsed: 0.002 sec.
3.时间类型:
Clickhouse支持Datetime,Datetime64 和Date类型
Datetime类型包含时分秒,精确到秒,支持用字符串形式插入。 [1970-01-01 00:00:00, 2105-12-31 23:59:59] 可以配合市区使用。
Datetime64 可以记录亚秒,在Datetime上增加了精度的设置。 语法:DateTime64(precision, [timezone])
Date 不包含具体的时间信息,只精确到天,支持字符串形式写入。 范围:1970-01-01 2105-12-31
最小输出为0000-00-00
hadoop101 :) create table dt(pk int,createtime datetime,createdate date,lastmodifytime datetime64(6)) engine=Memory;
查看表结构:
DESCRIBE TABLE dt
┌─name───────────┬─type──────────┬─default_type─┬─default_expression─┬─comment─┬─codec_expression─┬─ttl_expression─┐
│ pk │ Int32 │ │ │ │ │ │
│ createtime │ DateTime │ │ │ │ │ │
│ createdate │ Date │ │ │ │ │ │
│ lastmodifytime │ DateTime64(6) │ │ │ │ │ │
└────────────────┴───────────────┴──────────────┴────────────────────┴─────────┴──────────────────┴────────────────┘
insert into dt select 1,now(),'2020-06-09',now();
查看数据:
hadoop101 :) select * from dt;
┌─pk─┬──────────createtime─┬─createdate─┬─────────────lastmodifytime─┐
│ 1 │ 2020-06-09 05:18:48 │ 2020-06-09 │ 2020-06-09 05:18:48.000000 │
└────┴─────────────────────┴────────────┴────────────────────────────┘
hadoop101 :) insert into dt(pk,createtime,createdate,lastmodifytime)values(2,'2020-06-10 12:30:45','2020-06-10','2020-06-10 18:30:45:123456');
INSERT INTO dt (pk, createtime, createdate, lastmodifytime) VALUES
Exception on client:
Code: 6. DB::Exception: Cannot parse string '2020-06-10 18:30:45:123456' as DateTime64(6): syntax error at position 19 (parsed just '2020-06-10 18:30:45')
hadoop101 :) insert into dt(pk,createtime,createdate,lastmodifytime)values(2,'2020-06-10 12:30:45','2020-06-10','2020-06-10 18:30:45');
hadoop101 :) select * from dt;
SELECT *
FROM dt
┌─pk─┬──────────createtime─┬─createdate─┬─────────────lastmodifytime─┐
│ 1 │ 2020-06-09 05:18:48 │ 2020-06-09 │ 2020-06-09 05:18:48.000000 │
└────┴─────────────────────┴────────────┴────────────────────────────┘
┌─pk─┬──────────createtime─┬─createdate─┬─────────────lastmodifytime─┐
│ 2 │ 2020-06-10 12:30:45 │ 2020-06-10 │ 2020-06-10 18:30:45.000000 │
└────┴─────────────────────┴────────────┴────────────────────────────┘
2 rows in set. Elapsed: 0.002 sec.
其他支持的日期时间类型:
hadoop101 :) select * from system.data_type_families where name like 'Interval%';
┌─name────────────┬─case_insensitive─┬─alias_to─┐
│ IntervalYear │ 0 │ │
│ IntervalQuarter │ 0 │ │
│ IntervalMonth │ 0 │ │
│ IntervalDay │ 0 │ │
│ IntervalHour │ 0 │ │
│ IntervalSecond │ 0 │ │
│ IntervalMinute │ 0 │ │
│ IntervalWeek │ 0 │ │
└─────────────────┴──────────────────┴──────────┘
获取年份 季度 月份 日期 时 分 秒 周等信息。
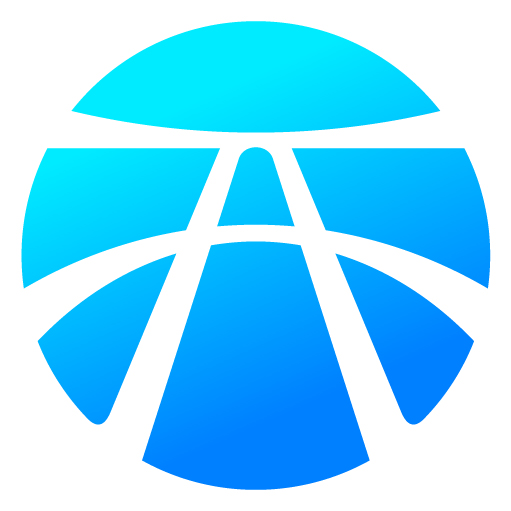
开放原子开发者工作坊旨在鼓励更多人参与开源活动,与志同道合的开发者们相互交流开发经验、分享开发心得、获取前沿技术趋势。工作坊有多种形式的开发者活动,如meetup、训练营等,主打技术交流,干货满满,真诚地邀请各位开发者共同参与!
更多推荐
所有评论(0)