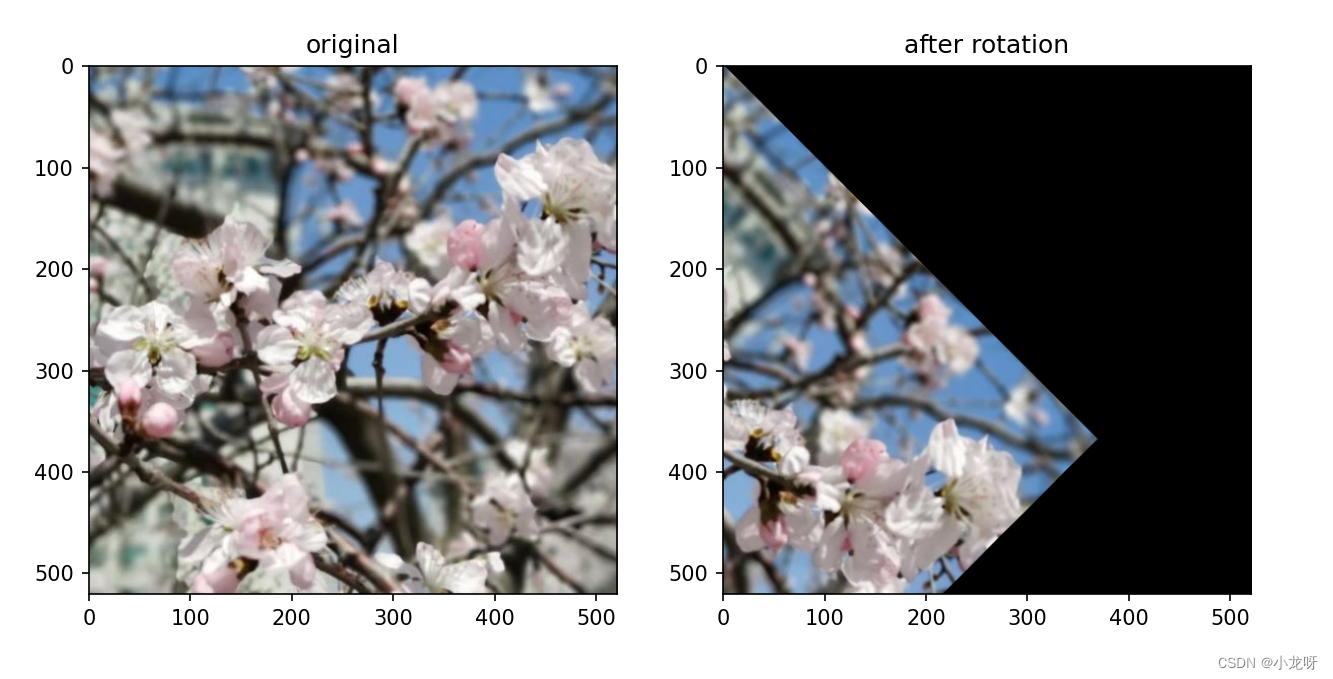
图像的几何变换—平移、旋转、镜像、缩放、剪切(原理+调用函数+像素操作)
图像的几何变换—平移、旋转、镜像、缩放、剪切(原理+调用函数+像素操作)
目录
一、平移
1.调用函数(平移矩阵)
图像平移后的坐标:
[
x
′
y
′
1
]
=
[
1
0
Δ
x
0
1
Δ
y
0
0
1
]
⋅
[
x
y
1
]
\begin{bmatrix} x' \\ y' \\ 1 \end{bmatrix} =\begin{bmatrix} 1&0&\Delta x \\ 0&1&\Delta y \\ 0&0&1 \end{bmatrix} \cdot \begin{bmatrix} x \\ y \\ 1 \end{bmatrix}
⎣
⎡x′y′1⎦
⎤=⎣
⎡100010ΔxΔy1⎦
⎤⋅⎣
⎡xy1⎦
⎤
{
x
′
=
x
+
Δ
x
y
′
=
y
+
Δ
y
\begin{cases} x' =x + \Delta x \\ y' =y + \Delta y \end{cases}
{x′=x+Δxy′=y+Δy
warpAffine函数用法参考这里:warpAffine函数
程序实现及运行结果:
import cv2
import matplotlib.pyplot as plt
import numpy as np
# 读取图像
img1 = cv2.imread("flower.jpeg")
# 图像平移
h, w = img1.shape[:2]
M = np.float32([[1, 0, 100], [0, 1, 50]]) # 平移矩阵,y方向向下平移50,x方向向右平移100
dst = cv2.warpAffine(img1, M, (w, h))
# 图像显示
fig, axes = plt.subplots(1, 2, figsize=(10, 8), dpi=100)
axes[0].imshow(img1[:, :, ::-1])
axes[0].set_title("original")
axes[1].imshow(dst[:, :, ::-1])
axes[1].set_title("after translation")
plt.show()
2.像素操作(遍历赋值)
算法思想很简单,首先将所有像素点沿x方向向右平移100,一行一行的进行处理,将(0,100)处的彩色值赋值给(0,0)处,(0,101)处的彩色值赋值给(0,1)处,然后把第0行所有列(521列)处理完。之后再处理下一行。同理沿着y方向向下平移50也是差不多这个思想。
至于这个从520到0也是有讲究的,因为我们为了避免移动过程发生覆盖而数据丢失,所以需要倒序处理。打个比方,第0行,假如我们从0列到520列,那么100列之后的值就会被覆盖,这样我们就不能正确将原来100列之后的初始值进行移动(赋值),而我们从第520列处理就可以完美的避免这个问题。
程序实现及运行结果:
import cv2
import matplotlib.pyplot as plt
img1 = cv2.imread('flower.jpeg')
img2 = img1.copy()
# x方向向右平移100
for j in range(521):
for i in range(520,-1,-1):
if i<100:
img2[j, i] = 0
else:
img2[j, i] = img2[j, i-100]
# y方向向下平移50
for u in range(521):
for v in range(520,-1,-1):
if v<50:
img2[v, u] = 0
else:
img2[v, u] = img2[v-50, u]
# 图像显示
fig, axes = plt.subplots(1, 2, figsize=(10, 8), dpi=100)
axes[0].imshow(img1[:, :, ::-1])
axes[0].set_title("original")
axes[1].imshow(img2[:, :, ::-1])
axes[1].set_title("after translation")
plt.show()
二、旋转
1.调用函数(旋转矩阵)
极坐标:
{
x
=
r
⋅
c
o
s
α
y
=
r
⋅
s
i
n
α
\begin{cases} x =r \cdot cos\alpha \\ y =r \cdot sin\alpha \end{cases}
{x=r⋅cosαy=r⋅sinα
{
x
′
=
r
⋅
c
o
s
(
α
+
θ
)
=
r
⋅
c
o
s
α
⋅
c
o
s
θ
−
r
⋅
s
i
n
α
⋅
s
i
n
θ
=
x
⋅
c
o
s
θ
−
y
⋅
s
i
n
θ
y
′
=
r
⋅
s
i
n
(
α
+
θ
)
=
r
⋅
s
i
n
α
⋅
c
o
s
θ
+
r
⋅
c
o
s
α
⋅
s
i
n
θ
=
x
⋅
s
i
n
θ
+
y
⋅
c
o
s
θ
\begin{cases} x' =r \cdot cos(\alpha+\theta)\\ \hspace{0.4cm} =r \cdot cos\alpha \cdot cos\theta - r \cdot sin\alpha \cdot sin\theta\\ \hspace{0.4cm} =x \cdot cos\theta - y \cdot sin\theta\\ \\ y' =r \cdot sin(\alpha+\theta)\\ \hspace{0.4cm} =r \cdot sin\alpha \cdot cos\theta + r \cdot cos\alpha \cdot sin\theta\\ \hspace{0.4cm} =x \cdot sin\theta + y \cdot cos\theta\\ \end{cases}
⎩
⎨
⎧x′=r⋅cos(α+θ)=r⋅cosα⋅cosθ−r⋅sinα⋅sinθ=x⋅cosθ−y⋅sinθy′=r⋅sin(α+θ)=r⋅sinα⋅cosθ+r⋅cosα⋅sinθ=x⋅sinθ+y⋅cosθ
直角坐标:
[
x
′
y
′
1
]
=
[
c
o
s
θ
−
s
i
n
θ
0
s
i
n
θ
c
o
s
θ
0
0
0
1
]
⋅
[
x
y
1
]
\begin{bmatrix} x' \\ y' \\ 1 \end{bmatrix} =\begin{bmatrix} cos\theta&-sin\theta&0 \\ sin\theta&cos\theta&0 \\ 0&0&1 \end{bmatrix} \cdot \begin{bmatrix} x \\ y \\ 1 \end{bmatrix}
⎣
⎡x′y′1⎦
⎤=⎣
⎡cosθsinθ0−sinθcosθ0001⎦
⎤⋅⎣
⎡xy1⎦
⎤
{
x
′
=
x
⋅
c
o
s
θ
−
y
⋅
s
i
n
θ
y
′
=
x
⋅
s
i
n
θ
+
y
⋅
c
o
s
θ
\begin{cases} x' =x \cdot cos\theta - y \cdot sin\theta\\ y' =x \cdot sin\theta + y \cdot cos\theta \end{cases}
{x′=x⋅cosθ−y⋅sinθy′=x⋅sinθ+y⋅cosθ
程序实现及运行结果:
import cv2
import matplotlib.pyplot as plt
import numpy as np
import math
# 读取图像
img1 = cv2.imread("blossom.jpeg")
# 图像旋转
h, w = img1.shape[:2]
M = np.float32([[math.cos(math.pi/4), -math.sin(math.pi/4), 0], [math.sin(math.pi/4), math.cos(math.pi/4), 0]]) # 顺时针旋转45°
dst = cv2.warpAffine(img1, M, (w, h))
# 图像显示
fig, axes = plt.subplots(1, 2, figsize=(10, 8), dpi=100)
axes[0].imshow(img1[:, :, ::-1])
axes[0].set_title("original")
axes[1].imshow(dst[:, :, ::-1])
axes[1].set_title("after rotation")
plt.show()
2.像素操作(反向映射)
此程序运用了一种反向映射的思想,这样可以确保旋转后的位置坐标都有彩色值,我们根据旋转矩阵的逆,可以找到旋转后的图像坐标所对应原图像的彩色值,从而对其进行赋值即可。
其中我们需要注意两个问题,一是我们旋转后的坐标位置所对应原图像的坐标可能是越界的(找不到坐标与其对应);二是我们求的坐标向量可能是负数,需要舍去。
程序实现及运行结果:
import cv2
import matplotlib.pyplot as plt
import numpy as np
import math
# 读取图像
img1 = cv2.imread("blossom.jpeg")
img2 = img1.copy()
# 图像旋转(反向映射)
RM1 = np.float32([[math.cos(math.pi/4), math.sin(math.pi/4), 0], [-math.sin(math.pi/4), math.cos(math.pi/4), 0], [0, 0, 1]]) # 计算出的旋转矩阵
RM2 = np.linalg.inv(RM1) # 求解旋转矩阵的逆
for i in range(521):
for j in range(521):
D = np.dot(RM2, [[i], [j], [1]]) # 旋转后的图像坐标位置 相对应的 原图像坐标位置
if int(D[0])>=521 or int(D[1])>=521: # 旋转后的图像坐标 相对应的 原图像坐标位置 越界
img2[i, j] = 0
elif int(D[0]) < 0 or int(D[1]) < 0: # 旋转后的图像坐标 相对应的 原图像坐标位置 负值
img2[i, j] = 0
else:
img2[i, j] = img1[int(D[0]), int(D[1])]
# 图像显示
fig, axes = plt.subplots(1, 2, figsize=(10, 8), dpi=100)
axes[0].imshow(img1[:, :, ::-1])
axes[0].set_title("original")
axes[1].imshow(img2[:, :, ::-1])
axes[1].set_title("after rotation")
plt.show()
三、镜像
水平镜像:
{
x
′
=
w
i
d
t
h
−
1
−
x
y
′
=
y
\begin{cases} x' =width-1-x\\ y' =y \end{cases}
{x′=width−1−xy′=y
[
x
′
y
′
1
]
=
[
−
1
0
w
i
d
t
h
−
1
0
1
0
0
0
1
]
⋅
[
x
y
1
]
\begin{bmatrix} x' \\ y' \\ 1 \end{bmatrix} =\begin{bmatrix} -1&0&width-1 \\ 0&1&0 \\ 0&0&1 \end{bmatrix} \cdot \begin{bmatrix} x \\ y \\ 1 \end{bmatrix}
⎣
⎡x′y′1⎦
⎤=⎣
⎡−100010width−101⎦
⎤⋅⎣
⎡xy1⎦
⎤
垂直镜像:
{
x
′
=
x
y
′
=
h
e
i
g
h
t
−
1
−
y
\begin{cases} x' =x\\ y' =height-1-y \end{cases}
{x′=xy′=height−1−y
[
x
′
y
′
1
]
=
[
1
0
0
0
−
1
h
e
i
g
h
t
−
1
0
0
1
]
⋅
[
x
y
1
]
\begin{bmatrix} x' \\ y' \\ 1 \end{bmatrix} =\begin{bmatrix} 1&0&0 \\ 0&-1&height-1 \\ 0&0&1 \end{bmatrix} \cdot \begin{bmatrix} x \\ y \\ 1 \end{bmatrix}
⎣
⎡x′y′1⎦
⎤=⎣
⎡1000−100height−11⎦
⎤⋅⎣
⎡xy1⎦
⎤
1.调用函数(镜像矩阵)
import cv2
import matplotlib.pyplot as plt
import numpy as np
# 读取图像
img1 = cv2.imread("technology building.jpeg")
# 图像水平镜像
h, w = img1.shape[:2]
M = np.float32([[-1, 0, 520], [0, 1, 0]])
dst1 = cv2.warpAffine(img1, M, (w, h))
# 图像垂直镜像
h, w = img1.shape[:2]
M = np.float32([[1, 0, 0], [0, -1, 520]])
dst2 = cv2.warpAffine(img1, M, (w, h))
# 图像显示
fig, axes = plt.subplots(1, 3, figsize=(80, 10), dpi=100)
axes[0].imshow(img1[:, :, ::-1])
axes[0].set_title("original")
axes[1].imshow(dst1[:, :, ::-1])
axes[1].set_title("after horizontal-mirror")
axes[2].imshow(dst2[:, :, ::-1])
axes[2].set_title("after vertical-mirror")
plt.show()
2.像素操作(反向映射)
import cv2
import matplotlib.pyplot as plt
import numpy as np
# 读取图像
img1 = cv2.imread("technology building.jpeg")
img2 = img1.copy()
img3 = img1.copy()
# 图像水平镜像
RM1 = np.float32([[1, 0, 0], [0, -1, 520], [0, 0, 1]]) # 计算出的旋转矩阵
RM2 = np.linalg.inv(RM1) # 求解旋转矩阵的逆
for i in range(521):
for j in range(521):
D = np.dot(RM2, [[i], [j], [1]]) # 旋转后的图像坐标位置 相对应的 原图像坐标位置
if int(D[0])>=521 or int(D[1])>=521: # 旋转后的图像坐标 相对应的 原图像坐标位置 越界
img2[i, j] = 0
elif int(D[0]) < 0 or int(D[1]) < 0: # 旋转后的图像坐标 相对应的 原图像坐标位置 负值
img2[i, j] = 0
else:
img2[i, j] = img1[int(D[0]), int(D[1])]
# 图像垂直镜像
RM3 = np.float32([[-1, 0, 520], [0, 1, 0], [0, 0, 1]]) # 计算出的旋转矩阵
RM4 = np.linalg.inv(RM3) # 求解旋转矩阵的逆
for i in range(521):
for j in range(521):
D = np.dot(RM4, [[i], [j], [1]]) # 旋转后的图像坐标位置 相对应的 原图像坐标位置
if int(D[0])>=521 or int(D[1])>=521: # 旋转后的图像坐标 相对应的 原图像坐标位置 越界
img3[i, j] = 0
elif int(D[0]) < 0 or int(D[1]) < 0: # 旋转后的图像坐标 相对应的 原图像坐标位置 负值
img3[i, j] = 0
else:
img3[i, j] = img1[int(D[0]), int(D[1])]
# 图像显示
fig, axes = plt.subplots(1, 3, figsize=(80, 10), dpi=100)
axes[0].imshow(img1[:, :, ::-1])
axes[0].set_title("original")
axes[1].imshow(img2[:, :, ::-1])
axes[1].set_title("after horizontal-mirror")
axes[2].imshow(img3[:, :, ::-1])
axes[2].set_title("after vertical-mirror")
plt.show()
四、缩放
1.缩小(八邻域均值)
算法思想很简单,就是我们遍历原图像的每一个像素,原图像(1080×1437)的每一个像素与自己所在的八邻域(9个像素值)取平均值,然后将像素放进模板(360×479)中形成新的图像。相当于我们用一个3×3的卷积核对原图像进行卷积操作。
一共需要注意两个问题,一是我们先对边界元素扩充一行一列,这样原图像边界也可以很好的进行卷积操作而不越界;二是我们在进行求和会超出255而越界(每个通道像素值0-255,比如我们用230+230,不会得到460,而是会变成460-255-1=204),如果不处理,就会让我们的缩小图像变成黑色,所以我这里是先将所有值缩小10倍相加再放大10呗,当然你也可以选择使用其它方式。
程序实现及运行结果:
import cv2
# 读取图像
img1 = cv2.imread("city.jpeg")
img3 = cv2.imread("img3.png") # 制作一个模板
# 图像缩小
img2 = cv2.copyMakeBorder(img1, 1, 1, 1, 1, cv2.BORDER_REFLECT) # 上下左右各扩充1,复制最近像素
# 索引列表(位置坐标)
list1 = list(range(1, 1080, 3))
list2 = list(range(360))
list3 = list(range(1, 1435, 3))
list4 = list(range(479))
# 一个像素点自身与八邻域取均值
for i,u in zip(list1, list2):
for j,v in zip(list3, list4):
# 防止越界
img3[u, v] = ((img2[i,j]/10 + img2[i-1,j-1]/10 + img2[i-1,j]/10 + img2[i,j+1]/10 + img2[i,j-1]/10 + img2[i,j+1]/10 + img2[i+1,j-1]/10 + img2[i+1,j]/10 + img2[i+1,j+1]/10) / 9)*10
# 保存图像
cv2.imwrite('shrink.jpeg',img3)
original:
shrink:
2.放大(双线性插值)
单线性插值:
y
−
y
0
x
−
x
0
=
y
1
−
y
0
x
1
−
x
0
\boxed {\frac {y-y_0} {x-x_0}=\frac {y_1-y_0} {x_1-x_0}}
x−x0y−y0=x1−x0y1−y0
y
=
x
1
−
x
x
1
−
x
0
⋅
y
0
+
x
−
x
0
x
1
−
x
0
⋅
y
1
=
d
1
d
⋅
y
0
+
d
0
d
⋅
y
1
\boxed {y = \frac {x_1-x} {x_1-x_0} \cdot y_0+\frac {x-x_0} {x_1-x_0} \cdot y_1}={ \frac {d_1} {d} \cdot y_0+\frac {d_0} {d} \cdot y_1}
y=x1−x0x1−x⋅y0+x1−x0x−x0⋅y1=dd1⋅y0+dd0⋅y1
这个公式表示的结果就是:x1和x0分别到x的距离作为权重,用于对y0和y1的加权。d0/d > d1/d,而y1到y < y0到y 说明y1比重更大,所以乘以d0/d。
双线性插值:
①x方向的单线性插值去分别计算R1、R2的像素值:
f
(
x
,
y
1
)
=
x
2
−
x
x
2
−
x
1
⋅
f
(
Q
11
)
+
x
−
x
0
x
1
−
x
0
⋅
f
(
Q
21
)
\boxed {f(x,y_1) = \frac {x_2-x} {x_2-x_1} \cdot f(Q_{11})+\frac {x-x_0} {x_1-x_0} \cdot f(Q_{21})}
f(x,y1)=x2−x1x2−x⋅f(Q11)+x1−x0x−x0⋅f(Q21)
f
(
x
,
y
2
)
=
x
2
−
x
x
2
−
x
1
⋅
f
(
Q
12
)
+
x
−
x
0
x
1
−
x
0
⋅
f
(
Q
22
)
\boxed {f(x,y_2)= \frac {x_2-x} {x_2-x_1} \cdot f(Q_{12})+\frac {x-x_0} {x_1-x_0} \cdot f(Q_{22})}
f(x,y2)=x2−x1x2−x⋅f(Q12)+x1−x0x−x0⋅f(Q22)
②y方向的单线性插值计算P点的像素值:
f
(
x
,
y
)
≈
y
2
−
y
y
2
−
y
1
⋅
f
(
x
,
y
1
)
+
y
−
y
1
y
2
−
y
1
⋅
f
(
x
,
y
2
)
\boxed {f(x,y) \approx \frac {y_2-y} {y_2-y_1} \cdot f(x,y_1)+\frac {y-y_1} {y_2-y_1} \cdot f(x,y_2)}
f(x,y)≈y2−y1y2−y⋅f(x,y1)+y2−y1y−y1⋅f(x,y2)
算法设计:
假设我们取原图像的四个像素(如图所示15、16行,20、21列)(黑圈和红圈),像素值分别为P1、P2、P3、P4。
以我们需要插值在(14.3,20.3)为例子,根据双线性插值原理计算:
Q12 = 0.3×P2 + 0.7×P1
Q34 = 0.3×P4 + 0.7×P3
PQ = 0.3×Q34 + 0.7×Q12
这样我们就可以得到绿色三角的像素值,根据每个插值的像素和原图像四个像素的距离得出它们各自的系数(权重),并能得到中间的(黄蓝紫黄)的像素值。
最后,我们再把这四个像进行相应的外扩(如第三块图所示),就可以把原来2×2的图像放大成一幅4×4的图像。(继承了原图像的4个像素值,利用双线性插值得到了新的12个像素值)
程序实现及运行结果:
import cv2
import matplotlib.pyplot as plt
# 定义一个类(双线性插值计算)
class bilinear_interpolation:
P1 = P2 = P3 = P4 = 0
# 计算图中绿色三角像素值
def green(self):
Q12_green = 0.3 * self.P2 + 0.7 * self.P1
Q34_green = 0.3 * self.P4 + 0.7 * self.P3
PQ_green = 0.3 * Q34_green + 0.7 * Q12_green
return PQ_green
# 计算图中蓝色三角像素值
def blue(self):
Q12_blue = 0.6 * self.P2 + 0.4 * self.P1
Q34_blue = 0.6 * self.P4 + 0.4 * self.P3
PQ_blue = 0.3 * Q34_blue + 0.7 * Q12_blue
return PQ_blue
# 计算图中紫色三角像素值
def purple(self):
Q12_purple = 0.3 * self.P2 + 0.7 * self.P1
Q34_purple = 0.3 * self.P4 + 0.7 * self.P3
PQ_purple = 0.6 * Q34_purple + 0.4 * Q12_purple
return PQ_purple
# 计算图中黄色三角像素值
def yellow(self):
Q12_yellow = 0.6 * self.P2 + 0.4 * self.P1
Q34_yellow = 0.6 * self.P4 + 0.4 * self.P3
PQ_yellow= 0.6 * Q34_yellow + 0.4 * Q12_yellow
return PQ_yellow
BI = bilinear_interpolation()
# 读取图像
img1 = cv2.imread("sunset.jpg")
img2 = cv2.imread("img4.png") # 制作一个模板
# 索引列表(位置坐标)
list1 = list(range(0, 322, 2))
list2 = list(range(0, 644, 4))
list3 = list(range(0, 500, 2))
list4 = list(range(0, 1000, 4))
# 放大图像(双线性插值)
for i,u in zip(list1, list2):
for j,v in zip(list3, list4):
img2[u, v], img2[u, v+3], img2[u+3, v], img2[u+3, v+3] = img1[i, j], img1[i, j+1], img1[i+1, j], img1[i+1, j+1] # 原图像未更改像素值
BI.P1 = img1[i, j]; BI.P2 = img1[i, j + 1]; BI.P3 = img1[i + 1, j]; BI.P4 = img1[i + 1, j + 1]
img2[u, v+1] = img2[u+1, v] = img2[u+1, v+1] =BI.green()
img2[u, v+2] = img2[u+1, v+3] = img2[u+1, v+2] =BI.blue()
img2[u+2, v] = img2[u+3, v+1] = img2[u+2, v+1] =BI.purple()
img2[u+3, v+2] = img2[u+2, v+3] = img2[u+2, v+2] =BI.yellow()
# 保存图像
cv2.imwrite('enlarged.jpeg',img2)
original:
enlarged:
五、剪切
索引切片
程序实现及运行结果:
import cv2
import matplotlib.pyplot as plt
# 读取图像
img1 = cv2.imread("podoid.jpeg")
print(img1.shape) # (1290, 1080, 3)
# 图像剪切
img2 = img1[420:800, 300:800]
print(img2.shape) # (380, 500, 3)
# 图像显示
fig, axes = plt.subplots(1, 2, figsize=(10, 8), dpi=100)
axes[0].imshow(img1[:, :, ::-1])
axes[0].set_title("original")
axes[1].imshow(img2[:, :, ::-1])
axes[1].set_title("shear")
plt.show()
本文代码已开源,欢迎大家进行二次开发:https://gitee.com/xiaolong_ROS/Graphics-Processing-and-Machine-Vision
如有错误或者不足之处,欢迎大家留言指正!
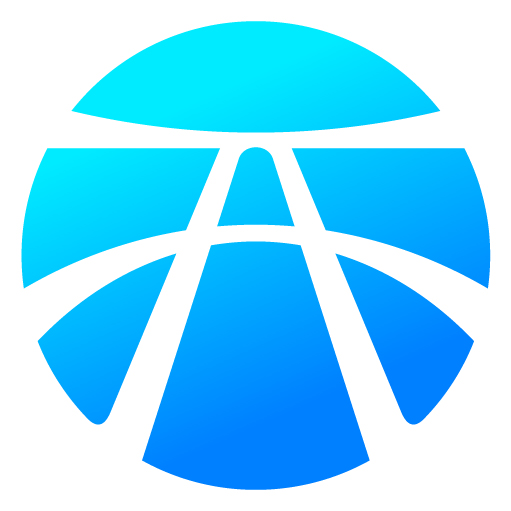
开放原子开发者工作坊旨在鼓励更多人参与开源活动,与志同道合的开发者们相互交流开发经验、分享开发心得、获取前沿技术趋势。工作坊有多种形式的开发者活动,如meetup、训练营等,主打技术交流,干货满满,真诚地邀请各位开发者共同参与!
更多推荐
所有评论(0)