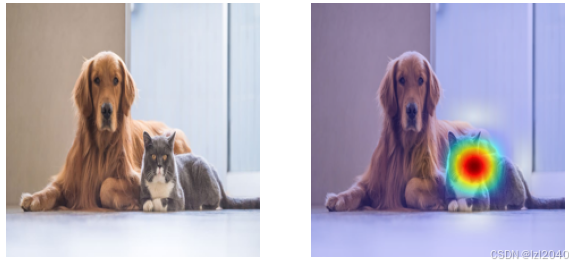
【深度学习总结】热力图-Grad-CAM使用
GradCAM使用
Grad-CAM使用
介绍
Grad-CAM,全称为Gradient-weighted Class Activation Mapping,是一种用于深度学习模型可视化的技术,特别是在卷积神经网络(CNN)中。它通过生成热力图来展示模型在做出决策时关注的区域,从而提供模型决策过程的可视化解释。
官方的代码pytorch-grad-cam中,里面也集成了其他热力图可视化方法。
源码解读
下面对pytorch-grad-cam的核心代码进行解读。
以语义分割的GradCAM为例,当我们使用它时,代码通常为:
from pytorch_grad_cam import GradCAM
class SemanticSegmentationTarget:
def __init__(self, category, mask):
self.category = category
self.mask = torch.from_numpy(mask)
if torch.cuda.is_available():
self.mask = self.mask.cuda()
def __call__(self, model_output):
return (model_output[self.category, :, : ] * self.mask).sum()
target_layers = [model.model.backbone.layer4]
targets = [SemanticSegmentationTarget(car_category, car_mask_float)]
with GradCAM(model=model,
target_layers=target_layers,
use_cuda=torch.cuda.is_available()) as cam:
grayscale_cam = cam(input_tensor=input_tensor,
targets=targets)[0, :]
其中model
是网络模型,target_layers
是需要可视化的层,input_tensor
是图片数据,targets
是想要最大化的目标,这里就是对属于“汽车”类别的所有像素的预测进行求和。
BaseCAM
在pytorch_grad_cam
库中,GradCAM
继承的类就是BaseCAM
,代码在:base_cam.py,GradCAM的代码在grad_cam.py,它只是实现了一个get_cam_weights
函数。
准备工作
在BaseCAM
的forward
函数中,它的参数为:
input_tensor
:输入数据targets
:想要最大化的目标,通常是一个nn.Module
类列表
它首先设置输入数据的梯度以及得到模型的梯度和激活值,如下:
self.outputs = outputs = self.activations_and_grads(input_tensor)
其中activations_and_grads
是专门抓取模型的梯度和激活值的,这个后面会讲,它返回的是模型的输出。
如果你没有提供targets
,它会按照分类模型的标准来构建:
if targets is None:
target_categories = np.argmax(outputs.cpu().data.numpy(), axis=-1)
targets = [ClassifierOutputTarget(category) for category in target_categories]
接下来就会根据target
来计算损失,然后进行梯度反向传播:
if self.uses_gradients:
self.model.zero_grad()
loss = sum([target(output) for target, output in zip(targets, outputs)])
loss.backward(retain_graph=True)
此时目标层的梯度和激活值已经保存在self.activations_and_grads
中。
热力图计算
然后就是计算每层的热力图,这是最重要的。先获取目标层的梯度和激活值,以及特征的大小:
activations_list = [a.cpu().data.numpy() for a in self.activations_and_grads.activations]
grads_list = [g.cpu().data.numpy() for g in self.activations_and_grads.gradients]
target_size = self.get_target_width_height(input_tensor)
然后遍历每个目标层,获取对应的梯度和激活值:
layer_activations = activations_list[i]
layer_grads = grads_list[i]
接着就是计算热力图,先获取对应的权重:
weights = self.get_cam_weights(input_tensor, target_layer, targets, activations, grads)
不同的激活图方法会有不同的实现,GradCAM
的做法就是对梯度进行平均:
# 2D image
if len(grads.shape) == 4:
return np.mean(grads, axis=(2, 3))
# 3D image
elif len(grads.shape) == 5:
return np.mean(grads, axis=(2, 3, 4))
得到权重后,对激活值进行加权,如下:
# 2D conv
if len(activations.shape) == 4:
weighted_activations = weights[:, :, None, None] * activations
# 3D conv
elif len(activations.shape) == 5:
weighted_activations = weights[:, :, None, None, None] * activations
然后,对加权的值在通道维度进行求和,得到最终的激活图,如果指定了平滑,还会使用平衡方法:
if eigen_smooth:
cam = get_2d_projection(weighted_activations)
else:
cam = weighted_activations.sum(axis=1)
最后取第一维最大的作为最终的激活图,并将激活图变成跟输入数据一样的大小:
cam = np.maximum(cam, 0)
scaled = scale_cam_image(cam, target_size)
得到所有目标层的激活图后,将它们在通过维度进行拼接,然后取平均值,得到最终的结果:
cam_per_target_layer = np.concatenate(cam_per_target_layer, axis=1)
cam_per_target_layer = np.maximum(cam_per_target_layer, 0)
result = np.mean(cam_per_target_layer, axis=1)
ActivationsAndGradients类
它负责抓取目标层的激活值和梯度,代码在:activations_and_gradients.py
在BaseCAM中通过如下方式创建:
self.activations_and_grads = ActivationsAndGradients(self.model, target_layers, reshape_transform)
其中model
是网络模型,target_layers
是目标层,是一个nn.Module
类列表。
在该类中,首先注册目标层的钩子函数:
for target_layer in target_layers:
self.handles.append(
target_layer.register_forward_hook(self.save_activation))
# Because of https://github.com/pytorch/pytorch/issues/61519,
# we don't use backward hook to record gradients.
self.handles.append(
target_layer.register_forward_hook(self.save_gradient))
其中register_forward_hook
的用法如下:
hook_handle = layer.register_forward_hook(hook_fn)
- layer:你想要添加 hook 的模型层(如卷积层、线性层等)。
- hook_fn:自定义的 hook 函数,用于在前向传播过程中处理数据。
hook_fn
的格式如下:hook_fn
是一个带有三个参数的函数:
def hook_fn(module, input, output):
# module 是当前的层
# input 是层的输入,通常是一个元组
# output 是层的输出
pass
- module:当前的层对象。
- input:传递给该层的输入数据(作为元组)。
- output:该层的输出数据。
它使用的保存梯度的函数如下:
def save_gradient(self, module, input, output):
if not hasattr(output, "requires_grad") or not output.requires_grad:
# You can only register hooks on tensor requires grad.
return
# Gradients are computed in reverse order
def _store_grad(grad):
if self.reshape_transform is not None:
grad = self.reshape_transform(grad)
self.gradients = [grad.cpu().detach()] + self.gradients
output.register_hook(_store_grad)
def save_activation(self, module, input, output):
activation = output
if self.reshape_transform is not None:
activation = self.reshape_transform(activation)
self.activations.append(activation.cpu().detach())
其中register_hook
函数允许你为张量注册一个钩子函数,该钩子函数会在计算梯度时被调用。
它执行通过一个call
函数:
def __call__(self, x):
self.gradients = []
self.activations = []
return self.model(x)
官方代码使用
官方的教程看这里:pytorch-gradcam-book
CLIP特征可视化——非官方代码
CLIP分为视觉编码器和文本编码器,其中视觉编码器有ResNet和ViT,这里以ResNet为例,可视化它的特征。
首先创建钩子函数,提取激活值和梯度:
class Hook:
"""Attaches to a module and records its activations and gradients."""
def __init__(self, module: nn.Module):
self.data = None
self.hook = module.register_forward_hook(self.save_grad)
def save_grad(self, module, input, output):
self.data = output
output.requires_grad_(True)
output.retain_grad()
def __enter__(self):
return self
def __exit__(self, exc_type, exc_value, exc_traceback):
self.hook.remove()
@property
def activation(self) -> torch.Tensor:
return self.data
@property
def gradient(self) -> torch.Tensor:
return self.data.grad
然后实现GradCAM,思路也十分简单,先计算梯度,然后根据梯度得到权重,再和激活值进行加权求和,从而得到激活图:
def gradCAM(
model: nn.Module,
input: torch.Tensor,
target: torch.Tensor,
layer: nn.Module
) -> torch.Tensor:
# 梯度归0
if input.grad is not None:
input.grad.data.zero_()
#
requires_grad = {}
for name, param in model.named_parameters():
requires_grad[name] = param.requires_grad
param.requires_grad_(False)
# 添加钩子函数
assert isinstance(layer, nn.Module)
with Hook(layer) as hook:
# 前向和后向传播
output = model(input)
output.backward(target)
grad = hook.gradient.float()
act = hook.activation.float()
# 在空间维度进行平均池化来得到权重
alpha = grad.mean(dim=(2, 3), keepdim=True)
# 通道维度加权求和
gradcam = torch.sum(act * alpha, dim=1, keepdim=True)
# 去除负值,只想要正值
gradcam = torch.clamp(gradcam, min=0)
# resize
gradcam = F.interpolate(
gradcam,
input.shape[2:],
mode='bicubic',
align_corners=False)
# 存储梯度设置
for name, param in model.named_parameters():
param.requires_grad_(requires_grad[name])
return gradcam
然后定义一些功能函数:
def normalize(x: np.ndarray) -> np.ndarray:
# Normalize to [0, 1].
x = x - x.min()
if x.max() > 0:
x = x / x.max()
return x
# Modified from: https://github.com/salesforce/ALBEF/blob/main/visualization.ipynb
def getAttMap(img, attn_map, blur=True):
if blur:
attn_map = filters.gaussian_filter(attn_map, 0.02*max(img.shape[:2]))
attn_map = normalize(attn_map)
cmap = plt.get_cmap('jet')
attn_map_c = np.delete(cmap(attn_map), 3, 2)
attn_map = 1*(1-attn_map**0.7).reshape(attn_map.shape + (1,))*img + \
(attn_map**0.7).reshape(attn_map.shape+(1,)) * attn_map_c
return attn_map
def viz_attn(img, attn_map, blur=True):
_, axes = plt.subplots(1, 2, figsize=(10, 5))
axes[0].imshow(img)
axes[1].imshow(getAttMap(img, attn_map, blur))
for ax in axes:
ax.axis("off")
plt.show()
def load_image(img_path, resize=None):
image = Image.open(img_path).convert("RGB")
if resize is not None:
image = image.resize((resize, resize))
return np.asarray(image).astype(np.float32) / 255.
最后将这些集成:
image_url = 'https://images2.minutemediacdn.com/image/upload/c_crop,h_706,w_1256,x_0,y_64/f_auto,q_auto,w_1100/v1554995050/shape/mentalfloss/516438-istock-637689912.jpg'
image_caption = 'the cat'
clip_model = "RN50" #["RN50", "RN101", "RN50x4", "RN50x16"]
saliency_layer = "layer4" #["layer4", "layer3", "layer2", "layer1"]
blur = True
device = "cuda" if torch.cuda.is_available() else "cpu"
model, preprocess = clip.load(clip_model, device=device, jit=False)
# 下载图片
image_path = 'image.png'
urllib.request.urlretrieve(image_url, image_path)
# 预处理
image_input = preprocess(Image.open(image_path)).unsqueeze(0).to(device)
image_np = load_image(image_path, model.visual.input_resolution)
text_input = clip.tokenize([image_caption]).to(device)
# 计算热力图
attn_map = gradCAM(
model.visual,
image_input,
model.encode_text(text_input).float(),
getattr(model.visual, saliency_layer)
)
attn_map = attn_map.squeeze().detach().cpu().numpy()
viz_attn(image_np, attn_map, blur)
最终的效果为:
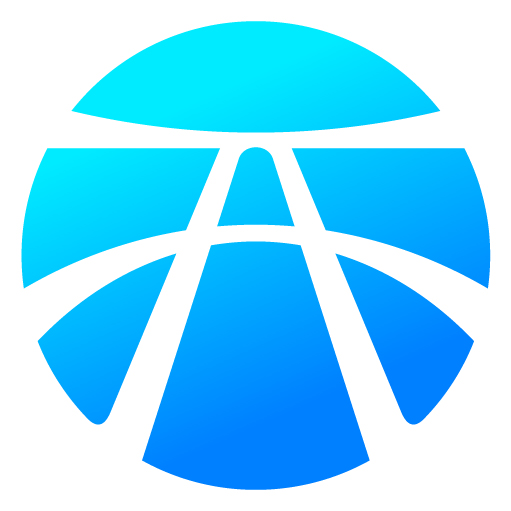
开放原子开发者工作坊旨在鼓励更多人参与开源活动,与志同道合的开发者们相互交流开发经验、分享开发心得、获取前沿技术趋势。工作坊有多种形式的开发者活动,如meetup、训练营等,主打技术交流,干货满满,真诚地邀请各位开发者共同参与!
更多推荐
所有评论(0)