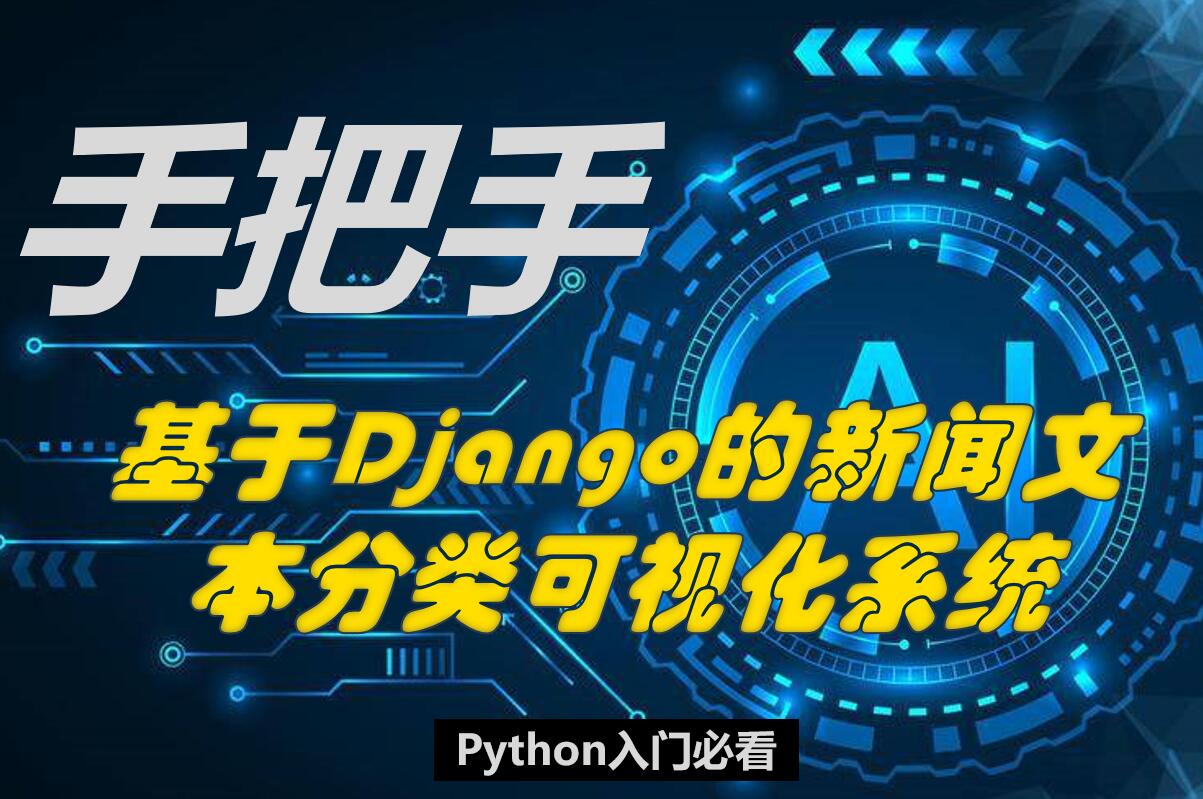
手把手教你:基于Django的新闻文本分类可视化系统(文本分类由bert实现)
本文主要介绍如何使用python语言,基于bert的文本分类和Django的网站设计实现一个:基于Django和bert的新闻文本分类可视化系统,如果有毕业设计或者课程设计需求的同学可以参考本文。本项目同时使用了深度学习框架TensorFlow 1.X的版本,IDE为pycharm。本次任务是一个较为复杂的新闻文本分类的任务,首先需要使用bert模型对新闻文本进行分类,然后使用Django构建一个
系列文章
一、项目简介
本文主要介绍如何使用python语言,基于bert的文本分类和Django的网站设计实现一个:基于Django和bert的新闻文本分类可视化系统,如果有毕业设计或者课程设计需求的同学可以参考本文。本项目同时使用了深度学习框架TensorFlow 1.X的版本,IDE为pycharm。完整代码在最下方,想要先看源码的同学可以移步本文最下方进行下载。
博主也参考过文本分类相关模型的文章,但大多是理论大于方法。很多同学肯定对原理不需要过多了解,只需要搭建出一个可视化系统即可。
也正是因为我发现网上大多的帖子只是针对原理进行介绍,功能实现的相对很少。
如果您有以上想法,那就找对地方了!
不多废话,直接进入正题!
二、任务介绍
本次任务是一个较为复杂的新闻文本分类的任务,首先需要使用bert模型对新闻文本进行分类,然后使用Django构建一个文本分类结果查询的可视化系统。
我们的任务是要构建一个模型,任意输入一篇新闻文章,可以将新闻文本分为以下几类:
label: ['体育', '财经', '房产', '家居', '教育', '科技', '时尚', '时政', '游戏', '娱乐']
三.界面简介
系统完成后界面如下:
- 页面一:一个文本输入界面,可以将需要分类的新闻文本写入对话框。
- 页面二:根据输入的文本调用后台模型进行预测并分类,我这里任意找了一个娱乐新闻,可以看到文本被正确分类了。
四.数据简介
本次使用的数据为标注后的文本,共计10类:['体育', '财经', '房产', '家居', '教育', '科技', '时尚', '时政', '游戏', '娱乐']
,见下图:
五、代码功能介绍
1.依赖环境集IDE
本项目使用的是anaconda的jupyter notebook编译环境,如不清楚如何使用的同学可以参考csdn上其他博主的基础教程,这里就不进行赘述。
tensorflow 1.9.0以上
sklearn
pandas
python3
2.数据处理
- 我们先通过脚本将数据集分为:
train.tsv
、dev.tsv
、test.tsv
、pre_test.tsv
,四部分
class TextProcessor(object):
"""按照InputExample类形式载入对应的数据集"""
"""load train examples"""
def get_train_examples(self, data_dir):
return self._create_examples(
self._read_file(os.path.join(data_dir, "train.tsv")), "train")
"""load dev examples"""
def get_dev_examples(self, data_dir):
return self._create_examples(
self._read_file(os.path.join(data_dir, "dev.tsv")), "dev")
"""load test examples"""
def get_test_examples(self, data_dir):
return self._create_examples(
self._read_file(os.path.join(data_dir, "test.tsv")), "test")
"""load pre examples"""
def get_pre_examples(self, data_dir):
return self._create_examples(
self._read_file(os.path.join(data_dir, "pre_test.tsv")), "test")
"""set labels"""
def get_labels(self):
return ['体育', '财经', '房产', '家居', '教育', '科技', '时尚', '时政', '游戏', '娱乐']
"""read file"""
def _read_file(self, input_file):
with codecs.open(input_file, "r",encoding='utf-8') as f:
lines = []
for line in f.readlines():
try:
line=line.split('\t')
assert len(line)==2
lines.append(line)
except:
pass
np.random.shuffle(lines)
return lines
"""create examples for the data set """
def _create_examples(self, lines, set_type):
examples = []
for (i, line) in enumerate(lines):
guid = "%s-%s" % (set_type, i)
text_a = tokenization.convert_to_unicode(line[1])
label = tokenization.convert_to_unicode(line[0])
examples.append(
InputExample(guid=guid, text_a=text_a, text_b=None, label=label))
return examples
3.模型构建及训练
- 然后打开控制台,运行
python text_run.py train
对模型进行训练。 - 这里附上模型训练代码:
def train():
"""训练bert模型"""
tensorboard_dir = os.path.join(config.output_dir, "tensorboard/textcnn")
save_dir = os.path.join(config.output_dir, "checkpoints/textcnn")
if not os.path.exists(tensorboard_dir):
os.makedirs(tensorboard_dir)
if not os.path.exists(save_dir):
os.makedirs(save_dir)
save_path = os.path.join(save_dir, 'best_validation')
start_time = time.time()
tf.logging.info("*****************Loading training data*****************")
train_examples = TextProcessor().get_train_examples(config.data_dir)
trian_data = convert_examples_to_features(train_examples, label_list, config.seq_length, tokenizer)
tf.logging.info("*****************Loading dev data*****************")
dev_examples = TextProcessor().get_dev_examples(config.data_dir)
dev_data = convert_examples_to_features(dev_examples, label_list, config.seq_length, tokenizer)
tf.logging.info("Time cost: %.3f seconds...\n" % (time.time() - start_time))
tf.logging.info("Building session and restore bert_model...\n")
session = tf.Session()
saver = tf.train.Saver()
session.run(tf.global_variables_initializer())
tf.summary.scalar("loss", model.loss)
tf.summary.scalar("accuracy", model.acc)
merged_summary = tf.summary.merge_all()
writer = tf.summary.FileWriter(tensorboard_dir)
writer.add_graph(session.graph)
optimistic_restore(session, config.init_checkpoint)
tf.logging.info('Training and evaluating...\n')
best_acc = 0
last_improved = 0 # record global_step at best_val_accuracy
flag = False
for epoch in range(config.num_epochs):
batch_train = batch_iter(trian_data, config.batch_size)
start = time.time()
tf.logging.info('Epoch:%d' % (epoch + 1))
for batch_ids, batch_mask, batch_segment, batch_label in batch_train:
feed_dict = feed_data(batch_ids, batch_mask, batch_segment, batch_label, config.keep_prob)
_, global_step, train_summaries, train_loss, train_accuracy = session.run([model.optim, model.global_step,
merged_summary, model.loss,
model.acc], feed_dict=feed_dict)
if global_step % config.print_per_batch == 0:
end = time.time()
val_loss, val_accuracy = evaluate(session, dev_data)
merged_acc = (train_accuracy + val_accuracy) / 2
if merged_acc > best_acc:
saver.save(session, save_path)
best_acc = merged_acc
last_improved = global_step
improved_str = '*'
else:
improved_str = ''
tf.logging.info(
"step: {},train loss: {:.3f}, train accuracy: {:.3f}, val loss: {:.3f}, val accuracy: {:.3f},training speed: {:.3f}sec/batch {}".format(
global_step, train_loss, train_accuracy, val_loss, val_accuracy,
(end - start) / config.print_per_batch, improved_str))
start = time.time()
if global_step - last_improved > config.require_improvement:
tf.logging.info("No optimization over 1500 steps, stop training")
flag = True
break
if flag:
break
config.lr *= config.lr_decay
4.模型测试
- 训练完成后我们使用
python text_run.py test
对模型进行测试。 - 这里附上模型测试代码:
def test():
"""testing"""
save_dir = os.path.join(config.output_dir, "checkpoints/textcnn")
save_path = os.path.join(save_dir, 'best_validation')
if not os.path.exists(save_dir):
tf.logging.info("maybe you don't train")
exit()
tf.logging.info("*****************Loading testing data*****************")
test_examples = TextProcessor().get_test_examples(config.data_dir)
test_data = convert_examples_to_features(test_examples, label_list, config.seq_length, tokenizer)
input_ids, input_mask, segment_ids = [], [], []
for features in test_data:
input_ids.append(features['input_ids'])
input_mask.append(features['input_mask'])
segment_ids.append(features['segment_ids'])
config.is_training = False
session = tf.Session()
session.run(tf.global_variables_initializer())
saver = tf.train.Saver()
saver.restore(sess=session, save_path=save_path)
tf.logging.info('Testing...')
test_loss, test_accuracy = evaluate(session, test_data)
msg = 'Test Loss: {0:>6.2}, Test Acc: {1:>7.2%}'
tf.logging.info(msg.format(test_loss, test_accuracy))
batch_size = config.batch_size
data_len = len(test_data)
num_batch = int((data_len - 1) / batch_size) + 1
y_test_cls = [features['label_ids'] for features in test_data]
y_pred_cls = np.zeros(shape=data_len, dtype=np.int32)
for i in range(num_batch):
start_id = i * batch_size
end_id = min((i + 1) * batch_size, data_len)
feed_dict = {
model.input_ids: np.array(input_ids[start_id:end_id]),
model.input_mask: np.array(input_mask[start_id:end_id]),
model.segment_ids: np.array(segment_ids[start_id:end_id]),
model.keep_prob: 1.0,
}
y_pred_cls[start_id:end_id] = session.run(model.y_pred_cls, feed_dict=feed_dict)
'''
输出测试矩阵
'''
# evaluate
tf.logging.info("Precision, Recall and F1-Score...")
tf.logging.info(metrics.classification_report(y_test_cls, y_pred_cls, target_names=label_list))
tf.logging.info("Confusion Matrix...")
cm = metrics.confusion_matrix(y_test_cls, y_pred_cls)
tf.logging.info(cm)
- 模型分类效果测试情况如下,这里用sklearn的混淆矩阵输出:
- 可以看到1w篇文章,10种类别测试
准确率
可以达到:92%,平均loss
在:0.54,这个loss不算低,因为博主时间有限所以跑的epoch
不多,有兴趣的同学可以继续跑,准确率
至少应该可以达到 96% 以上。
5.Django展示界面构建
由于展示界面代码较多,这里就不一一进行展示,感兴趣的同学可以在文章下方找到完整代码下载地址。
- 这里就附上加载较为关键的后端代码。
- 加载并初始化模型:
def get_model():
"""
模型初始化
"""
g_config = TextConfig()
save_dir = os.path.join(g_config.output_dir, "checkpoints/textcnn")
save_path = os.path.join(save_dir, 'best_validation')
g_start_time = time.time()
tf.logging.set_verbosity(tf.logging.INFO)
g_label_list = TextProcessor().get_labels()
g_tokenizer = tokenization.FullTokenizer(vocab_file=g_config.vocab_file, do_lower_case=False)
# 初始化模型
g_model = TextCNN(g_config)
g_end_time = time.time()
g_config.is_training = False
session = tf.Session()
session.run(tf.global_variables_initializer())
saver = tf.train.Saver()
saver.restore(sess=session, save_path=save_path)
print("模型初始化时间:", g_end_time - g_start_time)
return g_model, g_label_list, g_tokenizer, session
- 输入文本结果预测:
def get_pre(final_model, label_list, tokenizer,session):
"""
结果预测
"""
config = TextConfig()
save_dir = os.path.join(config.output_dir, "checkpoints/textcnn")
save_path = os.path.join(save_dir, 'best_validation')
if not os.path.exists(save_dir):
tf.logging.info("训练路径模型不存在,请检查:‘result/checkpoints/textcnn/’,"
"路径下是否有保存模型:best_validation.data-00000-of-00001")
exit()
tf.logging.info("*****************读取预测文件*****************")
test_examples = TextProcessor().get_pre_examples(config.data_dir)
test_data = convert_examples_to_features(test_examples, label_list, config.seq_length, tokenizer)
input_ids, input_mask, segment_ids = [], [], []
for features in test_data:
input_ids.append(features['input_ids'])
input_mask.append(features['input_mask'])
segment_ids.append(features['segment_ids'])
# config.is_training = False
# session = tf.Session()
# session.run(tf.global_variables_initializer())
# saver = tf.train.Saver()
# saver.restore(sess=session, save_path=save_path)
print('开始预测...')
# test_loss, test_accuracy = evaluate(session, test_data)
# msg = 'Test Loss: {0:>6.2}, Test Acc: {1:>7.2%}'
# tf.logging.info(msg.format(test_loss, test_accuracy))
batch_size = config.batch_size
data_len = len(test_data)
num_batch = int((data_len - 1) / batch_size) + 1
y_test_cls = [features['label_ids'] for features in test_data]
y_pred_cls = np.zeros(shape=data_len, dtype=np.int32)
for i in range(num_batch):
start_id = i * batch_size
end_id = min((i + 1) * batch_size, data_len)
feed_dict = {
final_model.input_ids: np.array(input_ids[start_id:end_id]),
final_model.input_mask: np.array(input_mask[start_id:end_id]),
final_model.segment_ids: np.array(segment_ids[start_id:end_id]),
final_model.keep_prob: 1.0,
}
y_pred_cls[start_id:end_id] = session.run(final_model.y_pred_cls, feed_dict=feed_dict)
pre_label = y_pred_cls[0]
print("预测index结果为:", pre_label)
return pre_label
六、代码下载地址
由于项目代码量和数据集较大,感兴趣的同学可以直接下载代码,使用过程中如遇到任何问题可以在评论区进行评论,我都会一一解答。
代码下载:
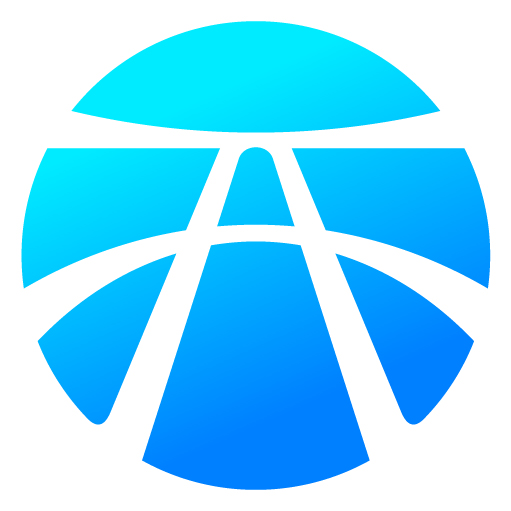
开放原子开发者工作坊旨在鼓励更多人参与开源活动,与志同道合的开发者们相互交流开发经验、分享开发心得、获取前沿技术趋势。工作坊有多种形式的开发者活动,如meetup、训练营等,主打技术交流,干货满满,真诚地邀请各位开发者共同参与!
更多推荐
所有评论(0)