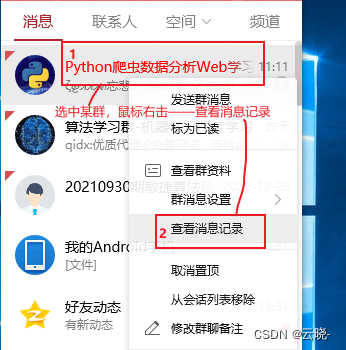
python分析QQ群聊天记录全过程,从获取到可视化
从选定的QQ群中获取记录,并对其进行处理及可视化。其中包括:共有多少人发过言,分别是谁谁发表的言论最多聊天密度周、日期、小时分布活跃天数最多的用户(在群里说话天数最多的用户)用户连续活跃天数(用户在群里连续说话天数)每个用户在群里连续说话最长天数以及时间段群里聊的最多的话题
随着社交媒体的兴起,QQ群成为了人们交流的重要平台,而提取这些数据可以帮助我们了解用户关注的重点和行为,那么如何获取QQ群聊天记录呢?如何对其进行处理并分析呢?
这是一套完整的流程,从选定的QQ群中获取记录,并对其进行处理及可视化。
其中包括:
共有多少人发过言,分别是谁
谁发表的言论最多
聊天密度周、日期、小时分布
活跃天数最多的用户(在群里说话天数最多的用户)
用户连续活跃天数(用户在群里连续说话天数)
每个用户在群里连续说话最长天数以及时间段
群里聊的最多的话题
目录
1 聊天记录获取
- 选中需要进行分析的群聊,鼠标右击——查看消息记录
- 在“消息管理器”中,在选中的群上面右击——导出消息记录
- 将消息记录保存为txt文件
2 聊天记录读取并查看
import pandas as pd
import numpy as np
#上下文管理语句
with open('E:\学习交流群.txt',mode = 'r',encoding='utf-8') as f:
txt = f.read() #返回的是字符串
txt #所有内容组成的字符串
3 数据处理
从源文件中我们可以看到内容中有以下几部分且比较直观,时间、用户名、QQ号以及聊天内容,而python读取的文件内容看起来极不方便。
3.1 使用正则提取信息
为了后面更好的分析探索数据,我们需要将读取到的字符串进行处理,存储为dataframe
import re
#正则表达式
re_pat = '[\d-]{10}\s[\d:]{8}\s.*[\)>]'#数字或者'-'共出现10位,\s(匹配任意的空白符)分开,数字或':'共8位,\s分开,任意字符以')'或'>'结尾
log_title_list = re.findall(re_pat,txt) #记录头
log_title_list
log_content_list = re.split(re_pat,txt) #记录内容,re_pat做分隔符,将文本进行分割
log_content_list
#查看两个列表量级是否一致
len(log_title_list)
len(log_content_list)
两个数据量并不一致,因为内容导出时,会有最前面的一行消息记录故需要做删除处理
#头与内容数量应保持一致,现发现内容多1,是因为多了第1行
log_content_list.pop(0) #删除第一行
len(log_content_list)
删除后,记录头与记录内容的数量已一致,将其存储到dataframe中
#将记录头及记录内容存储到dataframe中
df = pd.DataFrame()
df['title'] = log_title_list
df['content'] = log_content_list
df
3.2 拆分记录头并处理记录内容
3.2.1 将日期、用户名、QQ号分离出来
#分离日期、用户名、QQ号
df['time'] = df['title'].str.findall('\d{4}-\d{2}-\d{2}\s\d{2}:\d{2}:\d{2}').str.get(0)
df['name'] = df['title'].str.findall('\d{2}:\d{2}:\d{2}(.*)[\(<]').str.get(0) #提取用户名 从时间开始一直到'('为止,要的数据用'()'括起来
df['QQ'] = df['title'].str.findall('[\(<]([\dA-z\.@]*)[\)>]').str.get(0) #以'('或'<'开头,以')'或'>'结尾,中间部分可以是数字、字母、.、@的组合
3.2.2 处理内容
#删除空格
df['content'] = df['content'].str.replace('\n','')
3.2.3 删除无用内容
注意:删除、增加、拼接行数据后,需重置索引
- title列已经被拆分为“time”、“name”、“QQ”,在这里不保留原始数据,故将“title”列删除(可以根据实际情况选择保留与否)
#title列内容已被拆分,可以进行删除
df.drop(['title'],axis = 1,inplace = True)
df
- 删除无用的消息记录,比如系统提示信息等
# 单个的时候 != 多个的时候isin取反
df = df[~df['QQ'].isin(['1000000','10000'])]
df.reset_index(drop = True,inplace = True)
4 探索分析
4.1 共有多少人发过言,分别是谁
QQ号作为唯一标识,故通过QQ号来分析
df['QQ'].nunique() #去重后的计数
df['QQ'].unique() #去重后的数据列举出来
4.2 谁说话做多,说了什么
逻辑:消息出现一条则认为其说了一次话,按照QQ号对条数进行计数
#谁说话最多
# 也可以计数后直接使用 nlargest(1) 选出聊天内容最多的QQ号
#df['QQ'].value_counts().nlargest(1).index[0]
df['QQ'].value_counts()
#话痨说了什么
df[df['QQ'] =='1520034124']['content'].str.cat()
4.3 聊天密度分布
从周、日期、小时三个角度查看聊天情况
df.time #因为是正则匹配出来的,时间并不是标准的日期格式,需要进行处理
#转换为标准的时间
df['time'] = pd.to_datetime(df['time'])
4.3.1 聊天密度周分布
#若图表无法在notebook中显示出来,需要执行下面两行代码
#打开pylab模式,IPython中执行
%pylab
%matplotlib inline
df['weekday'] = df['time'].dt.weekday+1
df['weekday'].value_counts().sort_index().plot(kind='bar')
4.3.2 聊天密度日期分布
4.3.3 聊天密度小时分布
4.4 在群里说话天数最多的用户
逻辑:只要发过一条记录,就是在群里说话了,与当天发过多少消息无关,故需要“QQ”与“date”两列去重后统计次数
#QQ号与日期列去重
df.drop_duplicates(subset = ['QQ','date'])['QQ'].value_counts()
4.5 用户在群里连续说话天数
#数据 按照 'QQ','date' 两列 去重
df1 = df.drop_duplicates(subset = ['QQ','date'])[['QQ','date']]
df1.reset_index(drop = True,inplace = True)
# 将聊天时间列转换为日期类型
df1['date'] = pd.to_datetime(df1['date'])
# 对数据进行排序
df1 = df1.sort_values(by='date')
# 统计用户连续聊天的天数
result = df1.groupby('QQ')['date'].apply(lambda x: (x.diff().dt.days != 1).cumsum().value_counts())
result
4.6 每个用户在群里连续说话最长天数以及时间段
#数据 按照 'QQ','date' 两列 去重
df1 = df.drop_duplicates(subset = ['QQ','date'])[['QQ','date']]
# 将聊天时间列转换为日期类型
df1['date'] = pd.to_datetime(df1['date'])
# 对数据进行排序
df1.sort_values(by=['QQ','date'], inplace=True)
df1.reset_index(drop = True,inplace = True)
# 计算连续聊天的天数、起始时间和结束时间的函数
def calculate_consecutive_days(group):
if len(group) > 1:
#计算相邻日期之间的差异
consecutive_days = group['date'].diff().dt.days
#返回一个布尔值序列,指示日期差异是否不等于1
consecutive_days = consecutive_days.fillna(0) != 1
#计算布尔值序列的累积和
consecutive_days = consecutive_days.cumsum()
#最大连续天数
max_consecutive_days = consecutive_days.value_counts().max()
#最大连续天数对应id
id_max_consecutive_days = consecutive_days.value_counts().idxmax()
start_index = np.argmin(consecutive_days[consecutive_days.values == id_max_consecutive_days])
end_index = start_index + max_consecutive_days -1
start_date = group.loc[start_index]['date'].strftime('%Y-%m-%d')
end_date = group.loc[end_index]['date'].strftime('%Y-%m-%d')
return pd.Series([max_consecutive_days, start_date, end_date], index=['最长连续聊天天数', '起始时间', '结束时间'])
elif len(group) == 1: # 处理只有一次聊天记录的情况
date = group['date'].iloc[0].strftime('%Y-%m-%d')
return pd.Series([1, date, date], index=['最长连续聊天天数', '起始时间', '结束时间'])
else:
return pd.Series([0, np.nan, np.nan], index=['最长连续聊天天数', '起始时间', '结束时间'])
result = df1.groupby('QQ').apply(calculate_consecutive_days).reset_index()
result
4.6 群里聊的最多的话题
逻辑:先将聊天信息拼接起来,因为聊过过程中会有各种标题点符号等没有意义的词语,所以去除停用词之后,再进行分词,并用词云图展示
#内容拼接起来
content_txt = df['content'].str.cat(sep = '。')
#加载停用词
with open('stopwords.txt',encoding = 'utf-8') as file:
stopword = file.read()
import jieba
words = jieba.lcut(content_txt)
words = [word for word in words if (word not in stopword.split('\n'))&(len(word)>1)]
#大致看看是否已将无用信息去除完毕,若没有还需进行清洗
word_s = pd.Series(words).value_counts()
在这里可以发现,图片、表情等信息无法真实展现处理,而这些词对我们进行话题探索并没有什么意义,所以从拼接好的内容中,将“图片”、“表情”去除后,再进行分词而后分析。
content_txt = content_txt.replace('[图片]','').replace('[表情]','')
生成词云图pyecharts网址
#pycharts 里面提供的数据需要是 二维列表
data = word_s.reset_index().values.tolist()
#词云图
import pyecharts.options as opts
from pyecharts.charts import WordCloud
(
WordCloud()
.add(series_name="QQ", data_pair=data, word_size_range=[20, 66])
.set_global_opts(
title_opts=opts.TitleOpts(
title="QQ", title_textstyle_opts=opts.TextStyleOpts(font_size=23)
),
tooltip_opts=opts.TooltipOpts(is_show=True),
)
.render("QQ_wordcloud.html")
)
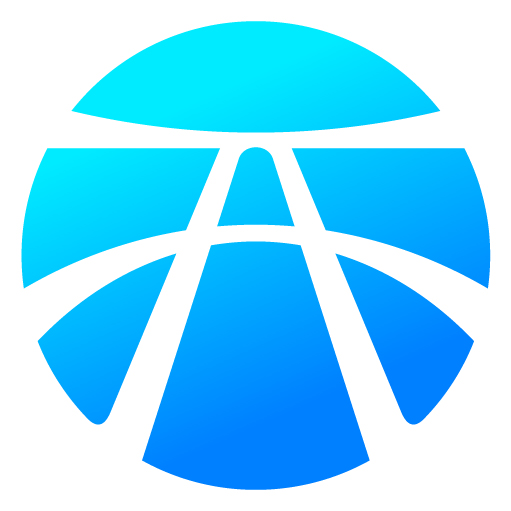
开放原子开发者工作坊旨在鼓励更多人参与开源活动,与志同道合的开发者们相互交流开发经验、分享开发心得、获取前沿技术趋势。工作坊有多种形式的开发者活动,如meetup、训练营等,主打技术交流,干货满满,真诚地邀请各位开发者共同参与!
更多推荐
所有评论(0)