基于pytorch的ConvGRU神经网络的实现与介绍
ConvGRU神经网络的介绍1.卷积神经网络介绍卷积神经网络(convlutional neural network)是一种具有局部连接,权重共享等特性的深层前馈神经网络特点:局部连接:在卷积层中每一个神经元都只和前一层中的某个局部窗口内的神经元相连,构成一个局部神经网络权重共享参数的卷积核w(l)w^{(l)}w(l)对于第l层的所有神经元都是相同的汇聚优点:平移、缩放、旋转不变性组成:目前卷积
基于pytorch的ConvGRU神经网络的实现与介绍
1.卷积神经网络介绍
- 卷积神经网络(convlutional neural network)是一种具有局部连接,权重共享等特性的深层前馈神经网络
-
特点:
- 局部连接:
- 在卷积层中每一个神经元都只和前一层中的某个局部窗口内的神经元相连,构成一个局部神经网络
- 权重共享
- 参数的卷积核 w ( l ) w^{(l)} w(l)对于第l层的所有神经元都是相同的
- 汇聚
- 局部连接:
-
优点:平移、缩放、旋转不变性
-
组成:目前卷积神经网络一般由卷积层、汇聚层和全连接层交叉堆叠而成
- 卷积层:
- 提取局部区域的特征,不同的卷积核相当于不同的特征提取器
- 特征映射:输入经过卷积提取到的特征,每个特征映射可以作为一类抽取的图像特征
- 卷积层:
-
程序实现:
- 在pytorch中卷积层的实现方式为加载torch.nn.nn中的函数进行实现
- 调用函数为nn.conv2D
- in_channels: 输入的通道数量
- out_channels:输出的通道数量
- kernel_size:卷积核尺寸
- stride:步长
- padding(补0):控制zero-padding的数目
- 输入输出说明:
- 输入的变量的维度应该为(batch_size, in_channels, width, length)
- 输出的为
-
import torch
import torch.nn as nn
conv = nn.Conv2d(in_channels=1,out_channels =16, kernel_size=3, stride =1)
inputs = torch.randn(1, 1, 64, 64) #(sampltNum, channels, width, length)
out = conv(inputs)
2.循环神经网络
-
GRU神经网络是一种循环神经网络,是LSTM的变种,在LSTM神经网络的基础上优化了cell结构减少了参数,加快了训练速度;
-
LSTM的计算公式为:
-
-
其中 f 为遗忘门,决定前一层传递过来的多大程度被遗忘掉
-
i 为输入门,控制当前计算的新状态多大程度更新到记忆细胞中
-
o 为输出门,控制当前输出有多大程度取决于当前的记忆单元
-
c 为记忆单元,可以看出细胞状态是有权重、输入、上一层的隐含层输入、上一层的记忆单元状态、输入门综合计算得到的
-
本层的隐含层状态则是由输出门与记忆细胞状态决定的
-
-
GRU的计算公式为:
*- GRU摒弃了LSTM中的记忆单元,并将输入门和遗忘门结合成了更新门(update gate)
- z 为更新门,决定有多少迁移一层的状态要更新当前神经元中
- h ^ \hat{h} h^为隐含层候选值,但是从最后一个函数可以看出,隐含层的候选值需要使用更新门来进行计算更新
-
LSTM和GRU的实现
- pytorch中提供了 LSTM和GRU的实现方式
- 参数
– input_size
– hidden_size
– num_layers
– bias
– batch_first
– dropout
– bidirectional - 输入
– input (seq_len, batch, input_size)
– h_0 (num_layers * num_directions, batch, hidden_size)
– c_0 (num_layers * num_directions, batch, hidden_size) - 输出
– output (seq_len, batch, num_directions * hidden_size)
– h_n (num_layers * num_directions, batch, hidden_size)
– c_n (num_layers * num_directions, batch, hidden_size)rnn = nn.LSTM(input_size=10, hidden_size=20, num_layers=2)#(input_size,hidden_size,num_layers) input = torch.randn(5, 3, 10)#(seq_len, batch, input_size) h0 = torch.randn(2, 3, 20) #(num_layers,batch,output_size) c0 = torch.randn(2, 3, 20) #(num_layers,batch,output_size) output, (hn, cn) = rnn(input, (h0, c0))
- 参数
- pytorch中提供了 LSTM和GRU的实现方式
3. ConvGRU介绍
ConvGRU是根据施博士的ConvLSTM进行了修改,将LSTM转化为了GRU进行计算。ConvLSTM是使用卷积核代替LSTM中的全连接层,即将全连接变为局部连接,使用GRU进行对比,基于torch进行计算,传统的GRU用torch表示前向传播过程为:
import torch
import torch.nn as nn
import torch.nn.functional as F
def GRU_forward(x, h_t_1):
"""GRU流程
args:
x: input
h_t_1: 上一层的隐含层输出值
shape:
x: [1, feature_size]
h_t_1: [hidden_size, hidden_size]
"""
linear_x_z = nn.Linear(10, 5) #(feature_size, hidden_size)
linear_h_z = nn.Linear(5, 5) #(hidden_size, hidden_size)
linear_x_r = nn.Linear(10, 5)
linear_h_r = nn.Linear(5, 5)
z_t = F.sigmoid(linear_x_z(x) + linear_h_z(h_t_1))
r_t = F.sigmoid(linear_x_r(x) + linear_h_r(h_t_1))
linear = nn.Linear(10,5)
linear_u = nn.Linear(5,5)
h_hat_t = F.tanh(linear(x) + linear_u(torch.mul(r_t, h_t_1)))
h_t = torch.mul((1 - z_t), h_t_1) + torch.mul(z_t, h_hat_t)
linear_out = nn.Linear(5, 1) #(hidden_size, out_size)
y = linear_out(h_t)
return y, h_t
### example ###
x = torch.randn(1,10)
h_t_1 = torch.randn(5,5)
y, h = GRU_forward(x, h_t_1)
而在ConvGRU中上述的linear层全部会转化为conv层且输入变量会发生变化,传统GRU中输入的为二维变量,而在ConvGRU中输入的为三维变量,convGRU的前向传播过程如下:
def convGru_forward(x, h_t_1):
"""GRU卷积流程
args:
x: input
h_t_1: 上一层的隐含层输出值
shape:
x: [1, channels, width, lenth]
"""
conv_x_z = nn.Conv2d(
in_channels=1, out_channels=4, kernel_size=1, stride=1)
conv_h_z = nn.Conv2d(
in_channels=4, out_channels=4, kernel_size=1, stride=1)
z_t = F.sigmoid(conv_x_z(x) + conv_h_z(h_t_1))
conv_x_r = nn.Conv2d(
in_channels=1, out_channels=4, kernel_size=1, stride=1)
conv_h_r = nn.Conv2d(
in_channels=4, out_channels=4, kernel_size=1, stride=1)
r_t = F.sigmoid((conv_x_r(x) + conv_h_r(h_t_1)))
conv = nn.Conv2d(
in_channels=1, out_channels=4, kernel_size=1, stride=1)
conv_u = nn.Conv2d(
in_channels=4, out_channels=4, kernel_size=1, stride=1)
h_hat_t = F.tanh(conv(x) + conv_u(torch.mul(r_t, h_t_1)))
h_t = torch.mul((1 - z_t), h_t_1) + torch.mul(z_t, h_hat_t)
conv_out = nn.Conv2d(
in_channels=4, out_channels=1, kernel_size=1, stride=1) #(hidden_size, out_size)
y = conv_out(h_t)
return y, h_t
x = torch.randn(1, 1, 16,16)
h_t_1 = torch.randn(1, 4, 16, 16)
y_3, h_3 = convGru_forward(x, h_t_1)
print(y_3.size())
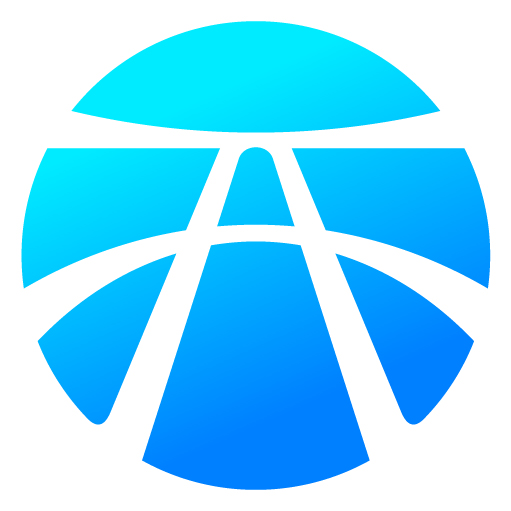
开放原子开发者工作坊旨在鼓励更多人参与开源活动,与志同道合的开发者们相互交流开发经验、分享开发心得、获取前沿技术趋势。工作坊有多种形式的开发者活动,如meetup、训练营等,主打技术交流,干货满满,真诚地邀请各位开发者共同参与!
更多推荐
所有评论(0)