[目标检测]-cv常用模块ghostbottleneck原理讲解与pytorch实现
1.ghostnet简介Ghostnet是华为诺亚方舟实验室今年在CVPR2020上新发表的文章《GhostNet: More Features from Cheap Operations》中提出的一种新型的网络结构,他的核心思想就是设计一种分阶段的卷积计算模块,在少量的非线性的卷积得到的特征图基础上,在进行一次线性卷积,从而获取更多的特征图,而新的到的特征图,就被叫做之前特征图的‘ghost’,
1.ghostnet简介
Ghostnet是华为诺亚方舟实验室今年在CVPR2020上新发表的文章《GhostNet: More Features from Cheap Operations》中提出的一种新型的网络结构,他的核心思想就是设计一种分阶段的卷积计算模块,在少量的非线性的卷积得到的特征图基础上,在进行一次线性卷积,从而获取更多的特征图,而新的到的特征图,就被叫做之前特征图的‘ghost’,以此来实现消除冗余特征,获取更加轻量的模型。
论文题名:《GhostNet: More Features from Cheap Operations》
arxiv: https://arxiv.org/abs/1911.11907
github :https://github.com/huawei-noah/ghostnet
2.整体设计思想
文章作者在观察ResNet50第一个残差块输出的特征图时,发现有许多输出特征很相似,图中的红、绿、蓝框的特征图,作者认为很相似,基本只要进行简单的线性变换就能得到,而不需要进行复杂的非线性变换得到。所以可以先通过一个非线性变化得到其中一个特征图,针对这个特征图做线性变化,得到原特征图的幽灵特征图。
注解
:这里说的非线性卷积操作是卷积-批归一化-非线性激活全套组合,而所谓的线性变换或者廉价操作均指普通卷积,不含批归一化和非线性激活。
3.模块设计
操作的前后对比图
a).就是直接普通的进行非线性
b).先进行一次普通的卷积(即卷积-批归一化-非线性激活),只不过它的输出特征图数量要少很多,目的就是减少计算量,然后在这个基础上通过线性变换(即卷积)。
假设图a和图b中输入层的通道数是c,输出层的通道数是n。图 b中输入层的通道数是c,第一阶段普通卷积的输出通道数是m,第二阶段Ghost module中包含1个idetity mapping和
个线性操作,每个线性操作的核大小为 d*d。该模块涉及到2个非常重要的参数,s和d 分别是线性变换的次数以及线性变换过程中卷积核的大小,本文给出的结果是这两者分别取2和3最合适.
4.理论性能
假设输入张量为从chw,分别为输入通道、特征图高和宽,经过一次卷积后为n*h’*w’,卷积核大小为k,线性变换卷积核大小为d,经过S次变换。那么之前a,b的计算量对比为。
理论speed of ratio计算公式为
n/s
是第一次变换时的输出通道数目
s-1
是因为恒等映射不需要进行计算,但它也算做第二变换中的一部分,Ghost 模块之所以能省计算量,就在这里。
最后,k
和d
均取3,主要是3x3卷积在网络设计中是最常用的。
理论compression ratio计算公式为
5.ghostmodule实现
class GhostModule(nn.Module):
def __init__(self, inp, oup, kernel_size=1, ratio=2, dw_size=3, stride=1, relu=True):
super(GhostModule, self).__init__()
self.oup = oup
init_channels = math.ceil(oup / ratio)
new_channels = init_channels*(ratio-1)
self.primary_conv = nn.Sequential(
nn.Conv2d(inp, init_channels, kernel_size, stride, kernel_size//2, bias=False),
nn.BatchNorm2d(init_channels),
nn.ReLU(inplace=True) if relu else nn.Sequential(),
)
self.cheap_operation = nn.Sequential(
nn.Conv2d(init_channels, new_channels, dw_size, 1, dw_size//2, groups=init_channels, bias=False),
nn.BatchNorm2d(new_channels),
nn.ReLU(inplace=True) if relu else nn.Sequential(),
)
def forward(self, x):
x1 = self.primary_conv(x)
x2 = self.cheap_operation(x1)
out = torch.cat([x1,x2], dim=1)
return out[:,:self.oup,:,:]
这部分github上就有,可以直接去看完整的。
6.ghostbottleneck
本文重点来了,这是一个可复用模块可以拿到现有的网络中替换掉bottleneck模块,从而减少计算了,降低模型体积。
- 类似于ResNet中的基本残差块。由两个堆叠的Ghost模块组成。第一个Ghost模块用作扩展层,增加了通道数。这里将输出通道数与输入通道数之比称为expansion ratio。第二个Ghost模块减少通道数,以与shortcut路径匹配。然后,使用shortcut连接这两个Ghost模块的输入和输出。
- Ghost Bottleneck中第二个Ghost module不使用ReLU其他层在每层之后都应用了批量归一化(BN)和ReLu激活函数(主要借鉴了MobileNetV2的思想)
- Ghost Bottleneck中对于stride = 2的情况,两个Ghost module之间通过一个stride = 2的深度卷积进行连接。
7.ghostbottleneck代码实现
class GhostBottleneck(nn.Module):
# Ghost Bottleneck https://github.com/huawei-noah/ghostnet
def __init__(self, c1, c2, k, s): # 如果s==1 则c1=c2 才能shortcut
super(GhostBottleneck, self).__init__()
c_ = c2 // 2
# print(c1, c2, c_, k, s)
self.conv = nn.Sequential(GhostConv(c1, c_, 1, 1), # pw
DWConv(c_, c_, k, s, act=False) if s == 2 else nn.Identity(), # dw
GhostConv(c_, c2, 1, 1, act=False)) # pw-linear
self.shortcut = nn.Sequential(DWConv(c1, c1, k, s, act=False),
Conv(c1, c2, 1, 1, act=False)) if s == 2 else nn.Identity()
def forward(self, x):
# print(self.conv(x))
# print(self.shortcut(x))
return self.conv(x) + self.shortcut(x)
class GhostConv(nn.Module):
# Ghost Convolution https://github.com/huawei-noah/ghostnet
def __init__(self, c1, c2, k=1, s=1, g=1, act=True): # ch_in, ch_out, kernel, stride, groups
super(GhostConv, self).__init__()
c_ = c2 // 2 # hidden channels
self.cv1 = Conv(c1, c_, k, s, None, g, act)
self.cv2 = Conv(c_, c_, 5, 1, None, c_, act)
# self.cv1 = Conv(c1, c_, k, s, g, act)
# self.cv2 = Conv(c_, c_, 5, 1, c_, act)
def forward(self, x):
y = self.cv1(x)
return torch.cat([y, self.cv2(y)], 1)
def DWConv(c1, c2, k=1, s=1, act=True):
# Depthwise convolution
return Conv(c1, c2, k, s, g=math.gcd(c1, c2), act=act)
class Conv(nn.Module):
# Standard convolution
def __init__(self, c1, c2, k=1, s=1, p=None, g=1, act=True): # ch_in, ch_out, kernel, stride, padding, groups
super(Conv, self).__init__()
self.conv = nn.Conv2d(c1, c2, k, s, autopad(k, p), groups=g, bias=False)
self.bn = nn.BatchNorm2d(c2)
self.act = nn.LeakyReLU(0.1, inplace=True) if act else nn.Identity()
def forward(self, x):
return self.act(self.bn(self.conv(x)))
def fuseforward(self, x):
return self.act(self.conv(x))
8.实验
我自己将ghostbottleneck写入到yolov5替换掉了bottleneckcps结构,FLOPs远低于之前版本,训练速度更快,差不多提升了20%左右,但是精度下降了1个点,目前仍然在调代码,我觉得完全可以实现,在相同map下,FLOPs性能超过yolov5原本的网络结构。个人看法欢迎讨论
下图为5l模型修改后的参数情况
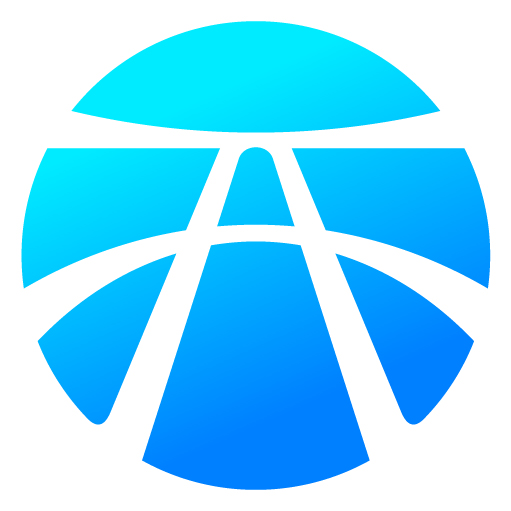
开放原子开发者工作坊旨在鼓励更多人参与开源活动,与志同道合的开发者们相互交流开发经验、分享开发心得、获取前沿技术趋势。工作坊有多种形式的开发者活动,如meetup、训练营等,主打技术交流,干货满满,真诚地邀请各位开发者共同参与!
更多推荐
所有评论(0)