基于opencv的红绿灯检测(python)
基于opencv的红绿灯检测(python)背景交通信号灯的检测与识别是无人驾驶与辅助驾驶必不可少的一部分,其识别精度直接关乎智能驾驶的安全。一般而言,在实际的道路场景中采集的交通信号灯图像具有复杂的背景,且感兴趣的信号灯区域只占很少的一部分。针对这些难点,国内外的众多研究者提出了相应的解决方案。总的来说,大多基于传统的图像处理方法;但目前也有用强学习能力的卷积神经网络去进行识别,但这类方法往往需
基于opencv的红绿灯检测(python)
目录
背景
交通信号灯的检测与识别是无人驾驶与辅助驾驶必不可少的一部分,其识别精度直接关乎智能驾驶的安全。一般而言,在实际的道路场景中采集的交通信号灯图像具有复杂的背景,且感兴趣的信号灯区域只占很少的一部分。针对这些难点,国内外的众多研究者提出了相应的解决方案。总的来说,大多基于传统的图像处理方法;但目前也有用强学习能力的卷积神经网络去进行识别,但这类方法往往需要大量的训练样本避免过拟合的风险。截至目前的大多数方法都是在各种颜色空间中利用信号灯颜色的先验进行分割得到兴趣区域,然后再通过信号灯所特有的形状特征和角点特征等进行进一步的判定。比如,Masako Omachi等人提出在RGB色彩空间分割交通信号灯,使用HOUGH变换检测分割出的信号灯所在的圆形区域;徐成等提出在Lab色彩空间分割交通信号灯,使用模板匹配的方法识别交通信号灯的状态;谷明琴等则在HSV色彩空间中使用颜色直方图统计图像的H分量,确定交通信号灯的类型。本项目将基于传统的图像处理算法来进行交通信号灯的识别,重点介绍核心技术。
正如前面所述,道路场景的多变性与复杂性,使得如何快速、准确地检测与识别交通信号灯,并且有效滤除图像中的干扰区域是交通信号灯检测与识别的关键。在图像处理算法设计中,为了提高算法的准确性与时效性,一般只关注局部感兴趣区域而不是整个图像区域。鉴于此,提出一种基于颜色分割与特征匹配相结合的方法,主要分为如下三个步骤:
1) 颜色分割
为了消除噪声,光照等因素的干扰,首先对采集的图像进行直方图均衡化。即:对每一个通道(R,G,B)数据进行直方图均衡化,再合并为一个3通道图像。颜色特征是交通信号灯重要而显著的特征之一。要对交通信号灯进行颜色分割,首先要选择合适的色彩空间。RGB色彩空间中的R、G、B这3个分量之间相关性较高,受光照影响较大,不利于颜色分割。因此,对RGB这3个通道数据进行归一化,即R=R./(R+G+B),G=G./(R+G+B),B=B./(R+G+B),然后,统计了不同环境条件下拍摄的交通信号灯的红色、绿色的R,G,B值,确定交通信号灯的颜色阈值。
2) 感兴趣区域提取
该步骤的主要目的为对分割的红色通道图像和绿色通道图像,进行联通区域的标定,并提取区域的基本几何特征,比如长度,宽度,长宽比,面积(即白色像素个数)。
3) 信号灯区域判定与识别
该步骤在前一步骤的基础上根据信号灯的特有特征过滤出真正的信号灯区域。本技术使用了3个先验知识:信号灯面积;信号灯形状;信号灯的黑色边框。
这篇博客介绍的是通过色彩空间识别交通灯。
1、导入相关库
import cv2
import os
import glob
import random
import numpy as np
import matplotlib.pyplot as plt
import matplotlib.image as mpimg
%matplotlib inline
import cv2:导入opencv相关库。
import os:我们在python下写程序,需要对文件以及文件夹或者其他的进行一系列的操作。但是,我们怎么在python中对文件进行操作呢?这就引入了os模块了。
import glob:glob是python自带的一个文件操作相关模块,用它可以查找符合自己目的文件。
import random:随机模块
import numpy as np:numpy科学数据库
import matplotlib.pyplot as plt:画图所需的matplotlib。
import matplotlib.image as mpimg:用mpimg导入图片得到的即为三通道数组,直接用plt显示即可。
%matplotlib inline: 可以在编译器里直接使用,功能是可以内嵌绘图,并且可以省略掉plt.show()这一步。
2、数据集的准备与读取
数据采用的是MIT自动驾驶课程的图像,总共三类:红绿黄,1187张图片,其中,723张红色交通灯图片,429张绿色交通灯图片,35张黄色交通灯图片,图片分为测试集和训练集。
获取数据集地址:
# Image data directories
IMAGEDIR_TRAINING = "E:\\car\\traffic_light_classify-master\\traffic_light_classify-master\\traffic_light_images\\training\\"
IMAGE_DIR_TEST = "E:\\car\\traffic_light_classify-master\\traffic_light_classify-master\\traffic_light_images\\test\\"
加载图像数据及图像标签
image_dir:图像存储位置的说明
#load data
def load_dataset(image_dir):
im_list =[]
image_types= ['red','yellow','green']
#遍历每个颜色文件夹
for im_type in image_types:
file_lists = glob.glob(os.path.join(image_dir,im_type,'*'))
print(len(file_lists))
for file in file_lists:
im = mpimg.imread(file)
if not im is None:
im_list.append((im,im_type))
return im_list
IMAGE_LIST = load_dataset(IMAGEDIR_TRAINING)
glob.glob();返回所有匹配的文件路径列表(list);该方法需要一个参数用来指定匹配的路径字符串(字符串可以为绝对路径也可以为相对路径),其返回的文件名只包括当前目录里的文件名,不包括子文件夹里的文件。
os.path.join()函数:连接两个或更多的路径名组件
结果:
723
35
429
3、数据可视化
第2部分已经进行了数据的读取,第三部分将用于查看并显示图像,并且为图像添加标题和标注。
fig,ax = plt.subplots(1,3,figsize=(5,2))
#red
img_red = IMAGE_LIST[0][0]
ax[0].imshow(img_red)
ax[0].annotate(IMAGE_LIST[0][1],xy=(2,5),color='blue',fontsize='10')
ax[0].axis('off')
ax[0].set_title(img_red.shape,fontsize=10)
#yellow
img_yellow= IMAGE_LIST[724][0]
plt.imshow(img_yellow)
ax[1].imshow(img_yellow)
ax[1].annotate(IMAGE_LIST[730][1],xy=(2,5),color='yellow',fontsize='10')
ax[1].axis('off')
ax[1].set_title(img_yellow.shape,fontsize=10)
#green
img_green= IMAGE_LIST[800][0]
plt.imshow(img_green)
ax[2].imshow(img_green)
ax[2].annotate(IMAGE_LIST[800][1],xy=(2,5),color='green',fontsize='10')
ax[2].axis('off')
ax[2].set_title(img_green.shape,fontsize=10)
plt.show()
.annotate:给图像进行标注
.set_title:给图像设计标题
结果:
3、图像标准化输入和输出
- 调整图像大小为32x32x3
- one_hot编码:red:[1,0,0],yellow:[0,1,0],green:[0,0,1]
代码通过几个函数实现:
def standardize(image_list):
standard_list = []
#Iterate through all the image-label pairs
for item in image_list:
image = item[0]
label = item[1]
standardized_im = standardize_input(image)
one_hot_label = one_hot_encode(label)
standard_list.append((standardized_im,one_hot_label))
return standard_list
def standardize_input(image):
#Resize all images to be 32x32x3
standard_im = cv2.resize(image,(32,32))
return standard_im
def one_hot_encode(label):
if label=='red':
return [1,0,0]
elif label=='yellow':
return [0,1,0]
else:
return [0,0,1]
4、代码测试
实现完了上述标准化代码后,我们需要进一步确定我们的代码是正确的,因此接下来我们可以实现一个函数来实现上述代码功能的检验,Python中有一个自带的单元测试框架是unittest模块,用它来做单元测试,它里面封装好了一些校验返回的结果方法和一些用例执行前的初始化操作。
关于unittest介绍可以参考链接博客https://www.cnblogs.com/feng0815/p/8045850.html
import unittest
from IPython.display import Markdown,display
def printmd(string):
display(Markdown(string))
def print_fail():
printmd('<span style=="color: red;">Test Failed</span>')
def print_pass():
printmd('<span style="color:green;">Test Passed</span>')
class Tests(unittest.TestCase):
def test_one_hot(self,one_hot_function):
try:
self.assertEqual([1,0,0],one_hot_function('red'))
self.assertEqual([0,1,0],one_hot_function('yellow'))
self.assertEqual([0,0,1],one_hot_function('green'))
except self.failureException as e:
print_fail()
print('Your function did not return the excepted one-hot label')
print('\n'+str(e))
return
print_pass()
def test_red_aa_green(self,misclassified_images):
#Loop through each misclassified image and the labels
for im,predicted_label,true_label in misclassified_images:
if(true_label==[1,0,0]):
try:
self.assertNotEqual(true_label,[0,1,0])
except self.failureException as e:
print_fail()
print('Warning:A red light is classified as green.')
print('\n'+str(e))
return
print_pass()
tests = Tests()
tests.test_one_hot(one_hot_encode)
结果
Test Passed
测试通过过后进行标准化输入与输出:
Standardized_Train_List = standardize(IMAGE_LIST)
5、进行图像特征提取
- 将彩色RGB空间转换为HSV空间,并且进行显示
#Visualize
image_num = 0
test_im = Standardized_Train_List[image_num][0]
test_label = Standardized_Train_List[image_num][1]
#convert to hsv
hsv = cv2.cvtColor(test_im, cv2.COLOR_RGB2HSV)
# Print image label
print('Label [red, yellow, green]: ' + str(test_label))
h = hsv[:,:,0]
s = hsv[:,:,1]
v = hsv[:,:,2]
# Plot the original image and the three channels
fig,ax = plt.subplots(1,4,figsize=(20,10))
ax[0].imshow(test_im)
ax[0].set_title('Standardized image')
ax[1].imshow(h,cmap='gray')
ax[1].set_title('H channel')
ax[2].set_title('S channel')
ax[2].imshow(s, cmap='gray')
ax[3].set_title('V channel')
ax[3].imshow(v, cmap='gray')
结果:从图像结果来看读取的是一张红灯图像,对应的Label:
Label [red, yellow, green]: [1, 0, 0]
也可以测试绿灯:对应的Label:
Label [red, yellow, green]: [0, 0, 1]
- HSV色彩空间特征提取
在图像处理中使用较多的是 HSV 颜色空间,它比 RGB 更接近人们对彩色的感知经验。非常直观地表达颜色的色调、鲜艳程度和明暗程度,方便进行颜色的对比。在 HSV 颜色空间下,比 BGR 更容易跟踪某种颜色的物体,常用于分割指定颜色的物体。HSV 表达彩色图像的方式由三个部分组成:
- Hue(色调、色相):是色彩的基本属性,就是平常所说的颜色名称,如红色、黄色等。
- Saturation(饱和度、色彩纯净度):是指色彩的纯度,越高色彩越纯,低则逐渐变灰,取0-100%的数值。
- Value(明度):取0-100%。
- 求图像的平均亮度
def create_feature(rgb_image):
hsv = cv2.cvtColor(rgb_image,cv2.COLOR_RGB2HSV)
sum_brightness = np.sum(hsv[:,:,2])
area = 3232
avg_brightness = sum_brightness / area#Find the average
return avg_brightness
2、求色彩饱和度
def high_saturation_pixels(rgb_image,threshold=80):
high_sat_pixels = []
hsv = cv2.cvtColor(rgb,cv2.COLOR_RGB2HSV)
for i in range(32):
for j in range(32):
if hsv[i][j][1] > threshold:
high_sat_pixels.append(rgb_image[i][j])
if not high_sat_pixels:
return highest_sat_pixel(rgb_image)
sum_red = 0
sum_green = 0
for pixel in high_sat_pixels:
sum_red+=pixel[0]
sum_green+=pixel[1]
avg_red = sum_red / len(high_sat_pixels)
avg_green = sum_green / len(high_sat_pixels)*0.8
return avg_red,avg_green
def highest_sat_pixel(rgb_image):
hsv = cv2.cvtColor(rgb_image,cv2.COLOR_RGB2HSV)
s = hsv[:,:,1]
x,y = (np.unravel_index(np.argmax(s),s.shape))
if rgb_image[x,y,0] > rgb_image[x,y,1]*0.9:
return 1,0 #red has a higher content
return 0,1
def estimate_label(rgb_image,display=False):
return red_green_yellow(rgb_image,display)
def findNoneZero(rgb_image):
rows=rgb_image.shape[0]
cols=rgb_image.shape[1]
counter = 0
for row in range(rows):
for col in range(cols):
pixels = rgb_image[row,col]
if sum(pixels)!=0:
counter = counter+1
return counter
3、确定红色、黄色、绿色的阈值
使用HSV和实验确定每张图像中的红、绿、黄内容确定阈值。返回基于值的分类。
这里是通过利用HSV色彩空间对图像进行算数操作:
cv2.bitwise_and() 函数:
cv2.bitwise_and()是对二进制数据进行“与”操作,即对图像(灰度图像或彩色图像均可)每个像素值进行二进制“与”操作,1&1=1,1&0=0,0&1=0,0&0=0
OutputArray dst = cv2.bitwise_and(InputArray src1, InputArray src2, InputArray mask=noArray());//dst = src1 & src2
利用掩膜(mask)进行“与”操作,即掩膜图像白色区域是对需要处理图像像素的保留,黑色区域是对需要处理图像像素的剔除,其余按位操作原理类似只是效果不同而已
def red_green_yellow(rgb_image,display):
'''
Determines the red , green and yellow content in each image using HSV and experimentally
determined thresholds. Returns a Classification based on the values
'''
hsv = cv2.cvtColor(rgb_image,cv2.COLOR_RGB2HSV)
sum_saturation = np.sum(hsv[:,:,1])# Sum the brightness values
area = 3232
avg_saturation = sum_saturation / area #find average
sat_low = int(avg_saturation*1.3)#均值的1.3倍
val_low = 140
#Green
lower_green = np.array([70,sat_low,val_low])
upper_green = np.array([100,255,255])
green_mask = cv2.inRange(hsv,lower_green,upper_green)
green_result = cv2.bitwise_and(rgb_image,rgb_image,mask = green_mask)
#Yellow
lower_yellow = np.array([10,sat_low,val_low])
upper_yellow = np.array([60,255,255])
yellow_mask = cv2.inRange(hsv,lower_yellow,upper_yellow)
yellow_result = cv2.bitwise_and(rgb_image,rgb_image,mask=yellow_mask)
# Red
lower_red = np.array([150,sat_low,val_low])
upper_red = np.array([180,255,255])
red_mask = cv2.inRange(hsv,lower_red,upper_red)
red_result = cv2.bitwise_and(rgb_image,rgb_image,mask = red_mask)
if display==True:
fig,ax = plt.subplots(1,5,figsize=(20,10))
ax[0].set_title('rgb image')
ax[0].imshow(rgb_image)
ax[1].set_title('red result')
ax[1].imshow(red_result)
ax[2].set_title('yellow result')
ax[2].imshow(yellow_result)
ax[3].set_title('green result')
ax[3].imshow(green_result)
ax[4].set_title('hsv image')
ax[4].imshow(hsv)
plt.show()
sum_green = findNoneZero(green_result)
sum_red = findNoneZero(red_result)
sum_yellow = findNoneZero(yellow_result)
if sum_red >= sum_yellow and sum_red>=sum_green:
return [1,0,0]#Red
if sum_yellow>=sum_green:
return [0,1,0]#yellow
return [0,0,1]#green
6、选择图像进行测试
img_test = [(img_red,'red'),(img_yellow,'yellow'),(img_green,'green')]
standardtest = standardize(img_test)
for img in standardtest:
predicted_label = estimate_label(img[0],display = True)
print('Predict label :',predicted_label)
print('True label:',img[1])
结果:
7、验证测试集图像准确率
关于准确的验证流程主要包括:加载测试集、标准化输入输出测试集、对测试集图像进行混洗、遍历所有测试图像对每个图像进行分类,并与真实的标签进行比较,从分类器获得预测的标签,比较真实标签和预测标签,最后计算出图像准确率。
针对于部分函数,建议读者去查阅相关资料,应该也不是很难。
code:
TEST_IMAGE_LIST = load_dataset(IMAGE_DIR_TEST)
STANDARDIZED_TEST_LIST = standardize(TEST_IMAGE_LIST)
random.shuffle(STANDARDIZED_TEST_LIST)
def get_misclassified_images(test_images,display=False):
misclassified_images_labels = []
for image in test_images:
im = image[0]
true_label = image[1]
assert (len(true_label)==3),'This true_label is not the excepted length (3).'
predicted_label = estimate_label(im,display=False)
assert(len(predicted_label)==3),'This predicted_label is not the excepted length (3).'
if(predicted_label!=true_label):
misclassified_images_labels.append((im,predicted_label,true_label))
return misclassified_images_labels
MISCLASSIFIED = get_misclassified_images(STANDARDIZED_TEST_LIST,display=False)
total = len(STANDARDIZED_TEST_LIST)
num_correct = total-len(MISCLASSIFIED)
accuracy = num_correct / total
print('Accuracy:'+str(accuracy))
print('Number of misclassfied images = '+str(len(MISCLASSIFIED))+' out of '+str(total))
测试集图像结果:
181
9
107
Accuracy:0.9090909090909091
Number of misclassfied images = 27 out of 297
完整代码链接:红绿灯检测代码
致谢
https://blog.csdn.net/weixin_41115751/article/details/84568029
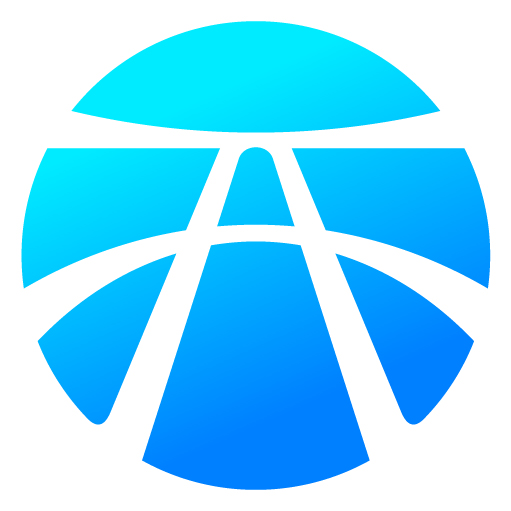
开放原子开发者工作坊旨在鼓励更多人参与开源活动,与志同道合的开发者们相互交流开发经验、分享开发心得、获取前沿技术趋势。工作坊有多种形式的开发者活动,如meetup、训练营等,主打技术交流,干货满满,真诚地邀请各位开发者共同参与!
更多推荐
所有评论(0)