Python 学习Pandas, 第 6、7课
关注微信公共号:小程在线关注CSDN博客:程志伟的博客完整脚本在公共号上有链接学习Pandas, 第 6课group by 应用Python 3.7.6 (default, Jan 8 2020, 20:23:39) [MSC v.1916 64 bit (AMD64)]Type "copyright", "credits" or "license" for more information.IP
关注微信公共号:小程在线
关注CSDN博客:程志伟的博客
完整脚本在公共号上有链接
学习Pandas, 第 6课
group by 应用
Python 3.7.6 (default, Jan 8 2020, 20:23:39) [MSC v.1916 64 bit (AMD64)]
Type "copyright", "credits" or "license" for more information.
IPython 7.12.0 -- An enhanced Interactive Python.
import pandas as pd
import sys
print('Python version ' + sys.version)
print('Pandas version ' + pd.__version__)
Python version 3.7.6 (default, Jan 8 2020, 20:23:39) [MSC v.1916 64 bit (AMD64)]
Pandas version 1.0.1
# 数据集
d = {'one':[1,1,1,1,1],
'two':[2,2,2,2,2],
'letter':['a','a','b','b','c']}
# 创建一个 dataframe
df = pd.DataFrame(d)
df
Out[2]:
one two letter
0 1 2 a
1 1 2 a
2 1 2 b
3 1 2 b
4 1 2 c
# 创建一个 groupby 对象
one = df.groupby('letter')
# 在分组上应用 sum() 函数
one.sum()
Out[3]:
one two
letter
a 2 4
b 2 4
c 1 2
不想把用来分组的列名字作为索引
letterone = df.groupby(['letter','one']).sum()
letterone
Out[4]:
two
letter one
a 1 4
b 1 4
c 1 2
letterone.index
Out[5]:
MultiIndex([('a', 1),
('b', 1),
('c', 1)],
names=['letter', 'one'])
letterone = df.groupby(['letter','one'], as_index=False).sum()
letterone
Out[6]:
letter one two
0 a 1 4
1 b 1 4
2 c 1 2
letterone.index
Out[7]: Int64Index([0, 1, 2], dtype='int64')
学习Pandas 第7课
计算离群值
import pandas as pd
import sys
print('Python version ' + sys.version)
print('Pandas version ' + pd.__version__)
Python version 3.7.6 (default, Jan 8 2020, 20:23:39) [MSC v.1916 64 bit (AMD64)]
Pandas version 1.0.1
# 创建一个 dataframe, 用日期作为索引
States = ['NY', 'NY', 'NY', 'NY', 'FL', 'FL', 'GA', 'GA', 'FL','FL']
data = [1.0, 2, 3, 4, 5, 6, 7, 8, 9, 10]
idx = pd.date_range('1/1/2012', periods=10, freq='MS')
df1 = pd.DataFrame(data, index=idx, columns=['Revenue'])
df1['State'] = States
# 创建第二个 dataframe
data2 = [10.0, 10.0, 9, 9, 8, 8, 7, 7, 6, 6]
idx2 = pd.date_range('1/1/2013', periods=10, freq='MS')
df2 = pd.DataFrame(data2, index=idx2, columns=['Revenue'])
df2['State'] = States
# 把两个 dataframe 合并起来
df = pd.concat([df1,df2])
df
Out[10]:
Revenue State
2012-01-01 1.0 NY
2012-02-01 2.0 NY
2012-03-01 3.0 NY
2012-04-01 4.0 NY
2012-05-01 5.0 FL
2012-06-01 6.0 FL
2012-07-01 7.0 GA
2012-08-01 8.0 GA
2012-09-01 9.0 FL
2012-10-01 10.0 FL
2013-01-01 10.0 NY
2013-02-01 10.0 NY
2013-03-01 9.0 NY
2013-04-01 9.0 NY
2013-05-01 8.0 FL
2013-06-01 8.0 FL
2013-07-01 7.0 GA
2013-08-01 7.0 GA
2013-09-01 6.0 FL
2013-10-01 6.0 FL
计算离群值的方法
注意: 均值(average)和标准差(Standard Deviation)只对高斯分布(gaussian distribution)有意义。
# 方法 1
# 原始的 df 拷贝一份
newdf = df.copy()
newdf['x-Mean'] = abs(newdf['Revenue'] - newdf['Revenue'].mean())
newdf['1.96*std'] = 1.96*newdf['Revenue'].std()
newdf['Outlier'] = abs(newdf['Revenue'] - newdf['Revenue'].mean()) > 1.96*newdf['Revenue'].std()
newdf
Out[11]:
Revenue State x-Mean 1.96*std Outlier
2012-01-01 1.0 NY 5.75 5.200273 True
2012-02-01 2.0 NY 4.75 5.200273 False
2012-03-01 3.0 NY 3.75 5.200273 False
2012-04-01 4.0 NY 2.75 5.200273 False
2012-05-01 5.0 FL 1.75 5.200273 False
2012-06-01 6.0 FL 0.75 5.200273 False
2012-07-01 7.0 GA 0.25 5.200273 False
2012-08-01 8.0 GA 1.25 5.200273 False
2012-09-01 9.0 FL 2.25 5.200273 False
2012-10-01 10.0 FL 3.25 5.200273 False
2013-01-01 10.0 NY 3.25 5.200273 False
2013-02-01 10.0 NY 3.25 5.200273 False
2013-03-01 9.0 NY 2.25 5.200273 False
2013-04-01 9.0 NY 2.25 5.200273 False
2013-05-01 8.0 FL 1.25 5.200273 False
2013-06-01 8.0 FL 1.25 5.200273 False
2013-07-01 7.0 GA 0.25 5.200273 False
2013-08-01 7.0 GA 0.25 5.200273 False
2013-09-01 6.0 FL 0.75 5.200273 False
2013-10-01 6.0 FL 0.75 5.200273 False
# 方法 2
# 分组的方法
# 原始的 df 拷贝一份
newdf = df.copy()
State = newdf.groupby('State')
newdf['Outlier'] = State.transform( lambda x: abs(x-x.mean()) >1.96*x.std() )
newdf['x-Mean'] = State.transform( lambda x: abs(x-x.mean()) )
newdf['1.96*std'] = State.transform( lambda x: 1.96*x.std() )
newdf
Out[12]:
Revenue State Outlier x-Mean 1.96*std
2012-01-01 1.0 NY False 5.00 7.554813
2012-02-01 2.0 NY False 4.00 7.554813
2012-03-01 3.0 NY False 3.00 7.554813
2012-04-01 4.0 NY False 2.00 7.554813
2012-05-01 5.0 FL False 2.25 3.434996
2012-06-01 6.0 FL False 1.25 3.434996
2012-07-01 7.0 GA False 0.25 0.980000
2012-08-01 8.0 GA False 0.75 0.980000
2012-09-01 9.0 FL False 1.75 3.434996
2012-10-01 10.0 FL False 2.75 3.434996
2013-01-01 10.0 NY False 4.00 7.554813
2013-02-01 10.0 NY False 4.00 7.554813
2013-03-01 9.0 NY False 3.00 7.554813
2013-04-01 9.0 NY False 3.00 7.554813
2013-05-01 8.0 FL False 0.75 3.434996
2013-06-01 8.0 FL False 0.75 3.434996
2013-07-01 7.0 GA False 0.25 0.980000
2013-08-01 7.0 GA False 0.25 0.980000
2013-09-01 6.0 FL False 1.25 3.434996
2013-10-01 6.0 FL False 1.25 3.434996
# 方法 2
# 多个条件分组
# 原始 df 拷贝一份
newdf = df.copy()
StateMonth = newdf.groupby(['State', lambda x: x.month])
newdf['Outlier'] = StateMonth.transform( lambda x: abs(x-x.mean()) > 1.96*x.std() )
newdf['x-Mean'] = StateMonth.transform( lambda x: abs(x-x.mean()) )
newdf['1.96*std'] = StateMonth.transform( lambda x: 1.96*x.std())
newdf
Out[13]:
Revenue State Outlier x-Mean 1.96*std
2012-01-01 1.0 NY False 4.5 12.473364
2012-02-01 2.0 NY False 4.0 11.087434
2012-03-01 3.0 NY False 3.0 8.315576
2012-04-01 4.0 NY False 2.5 6.929646
2012-05-01 5.0 FL False 1.5 4.157788
2012-06-01 6.0 FL False 1.0 2.771859
2012-07-01 7.0 GA False 0.0 0.000000
2012-08-01 8.0 GA False 0.5 1.385929
2012-09-01 9.0 FL False 1.5 4.157788
2012-10-01 10.0 FL False 2.0 5.543717
2013-01-01 10.0 NY False 4.5 12.473364
2013-02-01 10.0 NY False 4.0 11.087434
2013-03-01 9.0 NY False 3.0 8.315576
2013-04-01 9.0 NY False 2.5 6.929646
2013-05-01 8.0 FL False 1.5 4.157788
2013-06-01 8.0 FL False 1.0 2.771859
2013-07-01 7.0 GA False 0.0 0.000000
2013-08-01 7.0 GA False 0.5 1.385929
2013-09-01 6.0 FL False 1.5 4.157788
2013-10-01 6.0 FL False 2.0 5.543717
# 方法 3
# 分组的方法
# 原始 df 拷贝一份
newdf = df.copy()
State = newdf.groupby('State')
def s(group):
group['x-Mean'] = abs(group['Revenue'] - group['Revenue'].mean())
group['1.96*std'] = 1.96*group['Revenue'].std()
group['Outlier'] = abs(group['Revenue'] - group['Revenue'].mean()) > 1.96*group['Revenue'].std()
return group
Newdf2 = State.apply(s)
Newdf2
Out[14]:
Revenue State x-Mean 1.96*std Outlier
2012-01-01 1.0 NY 5.00 7.554813 False
2012-02-01 2.0 NY 4.00 7.554813 False
2012-03-01 3.0 NY 3.00 7.554813 False
2012-04-01 4.0 NY 2.00 7.554813 False
2012-05-01 5.0 FL 2.25 3.434996 False
2012-06-01 6.0 FL 1.25 3.434996 False
2012-07-01 7.0 GA 0.25 0.980000 False
2012-08-01 8.0 GA 0.75 0.980000 False
2012-09-01 9.0 FL 1.75 3.434996 False
2012-10-01 10.0 FL 2.75 3.434996 False
2013-01-01 10.0 NY 4.00 7.554813 False
2013-02-01 10.0 NY 4.00 7.554813 False
2013-03-01 9.0 NY 3.00 7.554813 False
2013-04-01 9.0 NY 3.00 7.554813 False
2013-05-01 8.0 FL 0.75 3.434996 False
2013-06-01 8.0 FL 0.75 3.434996 False
2013-07-01 7.0 GA 0.25 0.980000 False
2013-08-01 7.0 GA 0.25 0.980000 False
2013-09-01 6.0 FL 1.25 3.434996 False
2013-10-01 6.0 FL 1.25 3.434996 False
# 方法 3
# 多个条件分组
# 原始 df 拷贝一份
newdf = df.copy()
StateMonth = newdf.groupby(['State', lambda x: x.month])
def s(group):
group['x-Mean'] = abs(group['Revenue'] - group['Revenue'].mean())
group['1.96*std'] = 1.96*group['Revenue'].std()
group['Outlier'] = abs(group['Revenue'] - group['Revenue'].mean()) > 1.96*group['Revenue'].std()
return group
Newdf2 = StateMonth.apply(s)
Newdf2
Out[15]:
Revenue State x-Mean 1.96*std Outlier
2012-01-01 1.0 NY 4.5 12.473364 False
2012-02-01 2.0 NY 4.0 11.087434 False
2012-03-01 3.0 NY 3.0 8.315576 False
2012-04-01 4.0 NY 2.5 6.929646 False
2012-05-01 5.0 FL 1.5 4.157788 False
2012-06-01 6.0 FL 1.0 2.771859 False
2012-07-01 7.0 GA 0.0 0.000000 False
2012-08-01 8.0 GA 0.5 1.385929 False
2012-09-01 9.0 FL 1.5 4.157788 False
2012-10-01 10.0 FL 2.0 5.543717 False
2013-01-01 10.0 NY 4.5 12.473364 False
2013-02-01 10.0 NY 4.0 11.087434 False
2013-03-01 9.0 NY 3.0 8.315576 False
2013-04-01 9.0 NY 2.5 6.929646 False
2013-05-01 8.0 FL 1.5 4.157788 False
2013-06-01 8.0 FL 1.0 2.771859 False
2013-07-01 7.0 GA 0.0 0.000000 False
2013-08-01 7.0 GA 0.5 1.385929 False
2013-09-01 6.0 FL 1.5 4.157788 False
2013-10-01 6.0 FL 2.0 5.543717 False
# 原始的 df 拷贝一份
newdf = df.copy()
State = newdf.groupby('State')
newdf['Lower'] = State['Revenue'].transform( lambda x: x.quantile(q=.25) - (1.5*(x.quantile(q=.75)-x.quantile(q=.25))) )
newdf['Upper'] = State['Revenue'].transform( lambda x: x.quantile(q=.75) + (1.5*(x.quantile(q=.75)-x.quantile(q=.25))) )
newdf['Outlier'] = (newdf['Revenue'] < newdf['Lower']) | (newdf['Revenue'] > newdf['Upper'])
newdf
Out[16]:
Revenue State Lower Upper Outlier
2012-01-01 1.0 NY -7.000 19.000 False
2012-02-01 2.0 NY -7.000 19.000 False
2012-03-01 3.0 NY -7.000 19.000 False
2012-04-01 4.0 NY -7.000 19.000 False
2012-05-01 5.0 FL 2.625 11.625 False
2012-06-01 6.0 FL 2.625 11.625 False
2012-07-01 7.0 GA 6.625 7.625 False
2012-08-01 8.0 GA 6.625 7.625 True
2012-09-01 9.0 FL 2.625 11.625 False
2012-10-01 10.0 FL 2.625 11.625 False
2013-01-01 10.0 NY -7.000 19.000 False
2013-02-01 10.0 NY -7.000 19.000 False
2013-03-01 9.0 NY -7.000 19.000 False
2013-04-01 9.0 NY -7.000 19.000 False
2013-05-01 8.0 FL 2.625 11.625 False
2013-06-01 8.0 FL 2.625 11.625 False
2013-07-01 7.0 GA 6.625 7.625 False
2013-08-01 7.0 GA 6.625 7.625 False
2013-09-01 6.0 FL 2.625 11.625 False
2013-10-01 6.0 FL 2.625 11.625 False
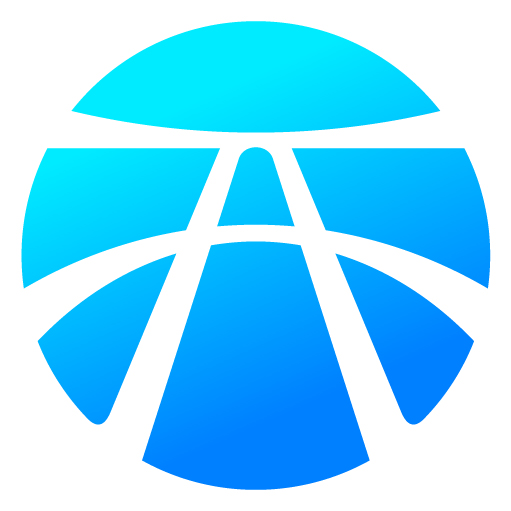
开放原子开发者工作坊旨在鼓励更多人参与开源活动,与志同道合的开发者们相互交流开发经验、分享开发心得、获取前沿技术趋势。工作坊有多种形式的开发者活动,如meetup、训练营等,主打技术交流,干货满满,真诚地邀请各位开发者共同参与!
更多推荐
所有评论(0)