【转】提取caffe前馈的中间结果+逐层可视化
转自:https://blog.csdn.net/thy_2014/article/details/51659300参考官方网址:http://nbviewer.jupyter.org/github/BVLC/caffe/blob/master/examples/00-classification.ipynb本文接着介绍如何在将caffe前馈时的中间结果显示出来。1 提取中间的...
转自: https://blog.csdn.net/thy_2014/article/details/51659300
参考官方网址:http://nbviewer.jupyter.org/github/BVLC/caffe/blob/master/examples/00-classification.ipynb
当caffe训练出一个模型,想用这个模型进行分类参见:使用caffe训练好的模型进行分类
本文接着介绍如何在将caffe前馈时的中间结果显示出来。
以下提到的代码并不能单独使用,需要加上使用caffe训练好的模型进行分类中的代码才能够正常运行。
1 提取中间的输出:
首先来看看怎么读出网络名称以及参数尺寸:
对于网络中的每层,典型的格式是:(batch_size, channel_dim, height, width)
这些写成了一个OrderedDict, net.blods.
-
-
# for each layer, show the output shape
-
for layer_name, blob
in net.blobs.iteritems():
-
print layer_name +
'\t' + str(blob.data.shape)
-
输出:
data (50, 3, 227, 227) conv1 (50, 96, 55, 55) pool1 (50, 96, 27, 27) norm1 (50, 96, 27, 27) conv2 (50, 256, 27, 27) pool2 (50, 256, 13, 13) norm2 (50, 256, 13, 13) conv3 (50, 384, 13, 13) conv4 (50, 384, 13, 13) conv5 (50, 256, 13, 13) pool5 (50, 256, 6, 6) fc6 (50, 4096) fc7 (50, 4096) fc8 (50, 1000) prob (50, 1000)再来看看参数尺寸:
这些参数写在另一个OrderedDict, net.params. 这里需要索引参数的结果,权重[0],偏置[1]
这些参数典型的格式是:
权重:(output_channels, input_channels, filter_height, filter_width)
偏置:(output_channels,)
-
-
for layer_name, param
in net.params.iteritems():
-
print layer_name +
'\t' + str(param[
0].data.shape), str(param[
1].data.shape)
-
输出:
conv1 (96, 3, 11, 11) (96,) conv2 (256, 48, 5, 5) (256,) conv3 (384, 256, 3, 3) (384,) conv4 (384, 192, 3, 3) (384,) conv5 (256, 192, 3, 3) (256,) fc6 (4096, 9216) (4096,) fc7 (4096, 4096) (4096,) fc8 (1000, 4096) (1000,)当处理4维数据的时候,定义一个 矩形热图显示函数非常有用:
-
def vis_square(data):
-
"""Take an array of shape (n, height, width) or (n, height, width, 3)
-
and visualize each (height, width) thing in a grid of size approx. sqrt(n) by sqrt(n)"""
-
-
# normalize data for display
-
data = (data - data.min()) / (data.max() - data.min())
-
-
# force the number of filters to be square
-
n = int(np.ceil(np.sqrt(data.shape[
0])))
-
padding = (((
0, n **
2 - data.shape[
0]),
-
(
0,
1), (
0,
1))
# add some space between filters
-
+ ((
0,
0),) * (data.ndim -
3))
# don't pad the last dimension (if there is one)
-
data = np.pad(data, padding, mode=
'constant', constant_values=
1)
# pad with ones (white)
-
-
# tile the filters into an image
-
data = data.reshape((n, n) + data.shape[
1:]).transpose((
0,
2,
1,
3) + tuple(range(
4, data.ndim +
1)))
-
data = data.reshape((n * data.shape[
1], n * data.shape[
3]) + data.shape[
4:])
-
-
plt.imshow(data); plt.axis(
'off')
来看看使用上面的函数来显示第1个卷尺层过滤器的数据:
-
-
# the parameters are a list of [weights, biases]
-
filters = net.params[
'conv1'][
0].data
-
vis_square(filters.transpose(
0,
2,
3,
1))
-
来看看第1个卷积层的输出数据(只显示了前36个):
-
feat = net.blobs[
'conv1'].data[
0, :
36]
-
vis_square(feat)
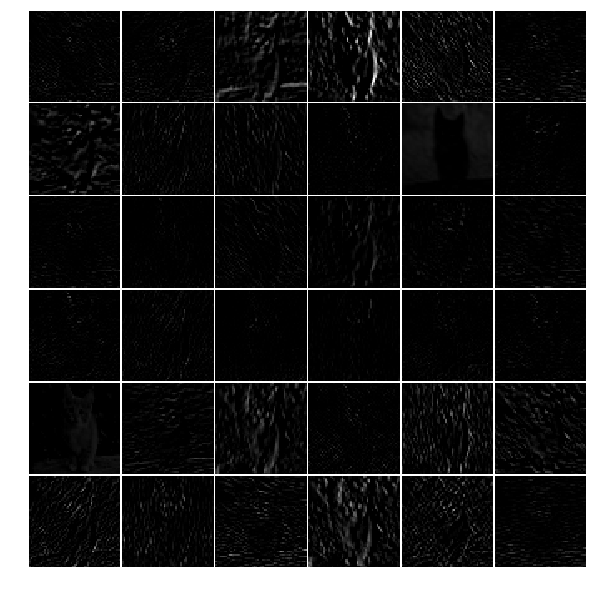
同样的道理,想要显示第5个卷积层相应的数据,第5个pooling层的数据,等都是一样的。
那么全连接层的数据怎么显示呢?
以fc6的输出为例:
-
feat = net.blobs[
'fc6'].data[
0]
-
plt.subplot(
2,
1,
1)
-
plt.plot(feat.flat)
-
plt.subplot(
2,
1,
2)
-
_ = plt.hist(feat.flat[feat.flat >
0], bins=
100)
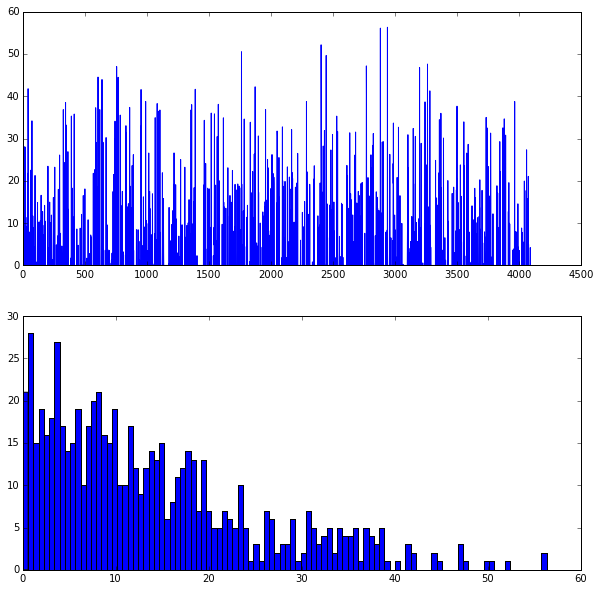
最后希望将所有分类的概率都画出来:
-
feat = net.blobs[
'prob'].data[
0]
-
plt.figure(figsize=(
15,
3))
-
plt.plot(feat.flat)
[<matplotlib.lines.Line2D at 0x7f09587dfb50>]
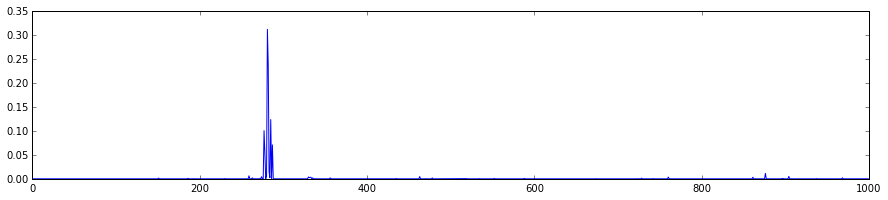
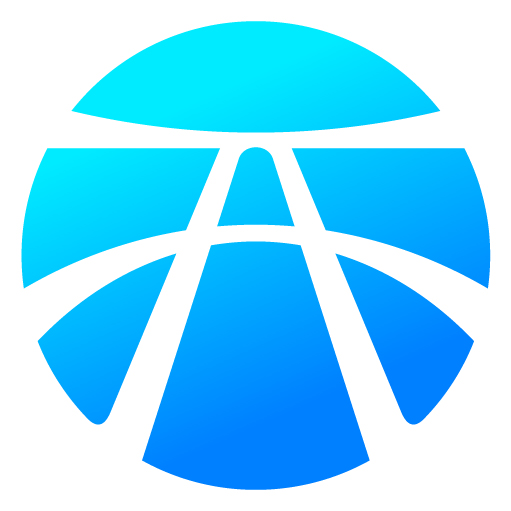
开放原子开发者工作坊旨在鼓励更多人参与开源活动,与志同道合的开发者们相互交流开发经验、分享开发心得、获取前沿技术趋势。工作坊有多种形式的开发者活动,如meetup、训练营等,主打技术交流,干货满满,真诚地邀请各位开发者共同参与!
更多推荐
所有评论(0)