COCO数据集标注框的读取及badcase analyse
目的:查看badcase的错误的时候的框大小值。本地调试程序至关重要,不然每次都要用服务器print,非常耗时耗力。macOS上PyCharm本地配置Anaconda环境博主代码地址:https://github.com/Xingxiangrui/multi_label_badcase_analyse/blob/master/badcase_analyse.py目录一、coc...
目的:查看badcase的错误的时候的框大小值。
本地调试程序至关重要,不然每次都要用服务器print,非常耗时耗力。
博主代码地址:https://github.com/Xingxiangrui/multi_label_badcase_analyse/blob/master/badcase_analyse.py
目录
一、coco的标签
1.1 coco标注的类型
COCO通过大量使用Amazon Mechanical Turk来收集数据。COCO数据集现在有3种标注类型:object instances(目标实例), object keypoints(目标上的关键点), 和image captions(看图说话),使用JSON文件存储。
$ ls
captions_train2014.json instances_train2014.json person_keypoints_train2014.json
captions_val2014.json instances_val2014.json person_keypoints_val2014.json
1.2 每种json内部
三种文件共享object instances(目标实例)、object keypoints(目标上的关键点)、image captions(看图说话)这3种类型共享这些基本类型:info、image、license
{
"info": info,
"licenses": [license],
"images": [image],
"annotations": [annotation],
}
info{
"year": int,
"version": str,
"description": str,
"contributor": str,
"url": str,
"date_created": datetime,
}
license{
"id": int,
"name": str,
"url": str,
}
image{
"id": int,
"width": int,
"height": int,
"file_name": str,
"license": int,
"flickr_url": str,
"coco_url": str,
"date_captured": datetime,
}
1.3 Object Instance
目标实例,这里面有相应的bbox信息,
分为下面这些段落
{
"info": info,
"licenses": [license],
"images": [image],
"annotations": [annotation],
"categories": [category]
}
其中的annotations为:
annotation{
"id": int,
"image_id": int,
"category_id": int,
"segmentation": RLE or [polygon],
"area": float,
"bbox": [x,y,width,height],
"iscrowd": 0 or 1,
}
包括了bbox,即标注框的坐标,以及area,标注区域的面积。
二、标签的加载
https://blog.csdn.net/banjuanshu/article/details/78370225
2.1 标签的加载
加载之后,会像dict一样。
with open(self.area_annotation_document) as f:
print('loading:',self.area_annotation_document)
instances_val = json.load(f)
print('loading done.')
其中annotations是对图片中物体标注的数据集,categories是所有物体的分类集,images是原始图片的信息
2.2 coco类别标签
category id
正常网络预测是80类,编写程序print出相应的预测。
def coco_categories_names(self):
# load json names id
with open(self.area_annotation_document) as f:
print('loading:',self.area_annotation_document)
instances_val = json.load(f)
print('loading done.')
coco_categories=instances_val['categories']
# load names id
with open('sk_spectral_cluster/coco_names.pkl', 'rb') as f:
print("loading coco_names.pkl")
names = pickle.load(f)
print('names',names)
print('coco json categories id',coco_categories)
网络预测结果对应的names
names ['person', 'bicycle', 'car', 'motorcycle', 'airplane', 'bus', 'train',
'truck', 'boat', 'traffic light', 'fire hydrant', 'stop sign', 'parking meter',
'bench', 'bird', 'cat', 'dog', 'horse', 'sheep', 'cow', 'elephant', 'bear',
'zebra', 'giraffe', 'backpack', 'umbrella', 'handbag', 'tie', 'suitcase',
'frisbee', 'skis', 'snowboard', 'sports ball', 'kite', 'baseball bat', 'baseball
glove', 'skateboard', 'surfboard', 'tennis racket', 'bottle', 'wine glass', 'cup',
'fork', 'knife', 'spoon', 'bowl', 'banana', 'apple', 'sandwich', 'orange',
'broccoli', 'carrot', 'hot dog', 'pizza', 'donut', 'cake', 'chair', 'couch',
'potted plant', 'bed', 'dining table', 'toilet', 'tv', 'laptop', 'mouse', 'remote',
'keyboard', 'cell phone', 'microwave', 'oven', 'toaster', 'sink', 'refrigerator', 'book', 'clock', 'vase', 'scissors', 'teddy bear', 'hair drier', 'toothbrush']
coco labels,与前面的names顺序一致,但是id一共有90类。
coco json categories id [{'supercategory': 'person', 'id': 1, 'name': 'person'}, {'supercategory': 'vehicle', 'id': 2, 'name': 'bicycle'}, {'supercategory':
'vehicle', 'id': 3, 'name': 'car'}, {'supercategory': 'vehicle', 'id': 4, 'name': 'motorcycle'}, {'supercategory': 'vehicle', 'id': 5, 'name': 'airplane'},
{'supercategory': 'vehicle', 'id': 6, 'name': 'bus'}, {'supercategory': 'vehicle', 'id': 7, 'name': 'train'}, {'supercategory': 'vehicle', 'id': 8, 'name': 'truck'},
{'supercategory': 'vehicle', 'id': 9, 'name': 'boat'}, {'supercategory': 'outdoor', 'id': 10, 'name': 'traffic light'}, {'supercategory': 'outdoor', 'id': 11, 'name':
'fire hydrant'}, {'supercategory': 'outdoor', 'id': 13, 'name': 'stop sign'}, {'supercategory': 'outdoor', 'id': 14, 'name': 'parking meter'}, {'supercategory':
'outdoor', 'id': 15, 'name': 'bench'}, {'supercategory': 'animal', 'id': 16, 'name': 'bird'}, {'supercategory': 'animal', 'id': 17, 'name': 'cat'},
{'supercategory': 'animal', 'id': 18, 'name': 'dog'}, {'supercategory': 'animal', 'id': 19, 'name': 'horse'}, {'supercategory': 'animal', 'id': 20, 'name': 'sheep'},
{'supercategory': 'animal', 'id': 21, 'name': 'cow'}, {'supercategory': 'animal', 'id': 22, 'name': 'elephant'}, {'supercategory': 'animal', 'id': 23, 'name':
'bear'}, {'supercategory': 'animal', 'id': 24, 'name': 'zebra'}, {'supercategory': 'animal', 'id': 25, 'name': 'giraffe'}, {'supercategory': 'accessory', 'id': 27,
'name': 'backpack'}, {'supercategory': 'accessory', 'id': 28, 'name': 'umbrella'}, {'supercategory': 'accessory', 'id': 31, 'name': 'handbag'}, {'supercategory':
'accessory', 'id': 32, 'name': 'tie'}, {'supercategory': 'accessory', 'id': 33, 'name': 'suitcase'}, {'supercategory': 'sports', 'id': 34, 'name': 'frisbee'},
{'supercategory': 'sports', 'id': 35, 'name': 'skis'}, {'supercategory': 'sports', 'id': 36, 'name': 'snowboard'}, {'supercategory': 'sports', 'id': 37, 'name':
'sports ball'}, {'supercategory': 'sports', 'id': 38, 'name': 'kite'}, {'supercategory': 'sports', 'id': 39, 'name': 'baseball bat'}, {'supercategory':
'sports', 'id': 40, 'name': 'baseball glove'}, {'supercategory': 'sports', 'id': 41, 'name': 'skateboard'}, {'supercategory': 'sports', 'id': 42, 'name':
'surfboard'}, {'supercategory': 'sports', 'id': 43, 'name': 'tennis racket'}, {'supercategory': 'kitchen', 'id': 44, 'name': 'bottle'}, {'supercategory':
'kitchen', 'id': 46, 'name': 'wine glass'}, {'supercategory': 'kitchen', 'id': 47, 'name': 'cup'}, {'supercategory': 'kitchen', 'id': 48, 'name': 'fork'},
{'supercategory': 'kitchen', 'id': 49, 'name': 'knife'}, {'supercategory': 'kitchen', 'id': 50, 'name': 'spoon'}, {'supercategory': 'kitchen', 'id': 51,
'name': 'bowl'}, {'supercategory': 'food', 'id': 52, 'name': 'banana'}, {'supercategory': 'food', 'id': 53, 'name': 'apple'}, {'supercategory': 'food',
'id': 54, 'name': 'sandwich'}, {'supercategory': 'food', 'id': 55, 'name': 'orange'}, {'supercategory': 'food', 'id': 56, 'name': 'broccoli'},
{'supercategory': 'food', 'id': 57, 'name': 'carrot'}, {'supercategory': 'food', 'id': 58, 'name': 'hot dog'}, {'supercategory': 'food', 'id': 59, 'name': 'pizza'},
{'supercategory': 'food', 'id': 60, 'name': 'donut'}, {'supercategory': 'food', 'id': 61, 'name': 'cake'}, {'supercategory': 'furniture', 'id': 62, 'name':
'chair'}, {'supercategory': 'furniture', 'id': 63, 'name': 'couch'}, {'supercategory': 'furniture', 'id': 64, 'name': 'potted plant'}, {'supercategory':
'furniture', 'id': 65, 'name': 'bed'}, {'supercategory': 'furniture', 'id': 67, 'name': 'dining table'}, {'supercategory': 'furniture', 'id': 70, 'name':
'toilet'}, {'supercategory': 'electronic', 'id': 72, 'name': 'tv'}, {'supercategory': 'electronic', 'id': 73, 'name': 'laptop'}, {'supercategory':
'electronic', 'id': 74, 'name': 'mouse'}, {'supercategory': 'electronic', 'id': 75, 'name': 'remote'}, {'supercategory': 'electronic', 'id': 76, 'name': 'keyboard'},
{'supercategory': 'electronic', 'id': 77, 'name': 'cell phone'}, {'supercategory': 'appliance', 'id': 78, 'name': 'microwave'}, {'supercategory': 'appliance', 'id':
79, 'name': 'oven'}, {'supercategory': 'appliance', 'id': 80, 'name': 'toaster'}, {'supercategory': 'appliance', 'id': 81, 'name': 'sink'}, {'supercategory':
'appliance', 'id': 82, 'name': 'refrigerator'}, {'supercategory': 'indoor', 'id': 84, 'name': 'book'}, {'supercategory': 'indoor', 'id': 85, 'name': 'clock'},
{'supercategory': 'indoor', 'id': 86, 'name': 'vase'}, {'supercategory': 'indoor', 'id': 87, 'name': 'scissors'}, {'supercategory': 'indoor', 'id': 88, 'name': 'teddy
bear'}, {'supercategory': 'indoor', 'id': 89, 'name': 'hair drier'}, {'supercategory': 'indoor', 'id': 90, 'name': 'toothbrush'}]
结果打出来发现coco的标签有跳跃的现象:
38 coco_json: id 43 names tennis racket
39 names: bottle
39 coco_json: id 44 names bottle
40 names: wine glass
40 coco_json: id 46 names wine glass
41 names: cup
41 coco_json: id 47 names cup
可以再多创建一个映射,从网络预测输出到coco的category id的映射。
# load json names id
with open(self.area_annotation_document) as f:
print('loading:',self.area_annotation_document)
instances_val = json.load(f)
print('loading done.')
coco_categories=instances_val['categories']
# load names id
with open('sk_spectral_cluster/coco_names.pkl', 'rb') as f:
print("loading coco_names.pkl")
names = pickle.load(f)
predict_id_to_json_id={}
for idx in range(len(names)):
# print(idx,'names:', names[idx])
# print(idx,'coco_json:','id',coco_categories[idx]['id'],'names',coco_categories[idx]['name'])
predict_id_to_json_id[idx]=coco_categories[idx]['id']
三、创建直方图以及写入
3.1 创建直方图
https://blog.csdn.net/xjl271314/article/details/80295935
打算先用plt.hist,再用plt.savefig函数
3.2 plt.hist
https://blog.csdn.net/denny2015/article/details/50581784
n, bins, patches = plt.hist(arr, bins=10, normed=0, facecolor='black', edgecolor='black',alpha=1,histtype='bar')
hist的参数非常多,但常用的就这六个,只有第一个是必须的,后面四个可选
- arr: 需要计算直方图的一维数组
- bins: 直方图的柱数,可选项,默认为10
- normed: 是否将得到的直方图向量归一化。默认为0
- facecolor: 直方图颜色
- edgecolor: 直方图边框颜色
- alpha: 透明度
- histtype: 直方图类型,‘bar’, ‘barstacked’, ‘step’, ‘stepfilled’
返回值 :
- n: 直方图向量,是否归一化由参数normed设定
- bins: 返回各个bin的区间范围
- patches: 返回每个bin里面包含的数据,是一个list
3.3 创建直方图
直接根据list创建直方图即可。
def hist_try(self):
area_list=[235939.0035, 64153.92420000001, 797.3219000000005, 40377.498349999994, 825.0993000000005, 122283.17504999999, 110586.77519999997, 23235.967249999994, 7660.271999999997, 2845.3616000000015, 5078.0296, 39.01750000000018, 13050.5062, 1644.8198499999994, 9904.229450000003, 2675.0394999999994, 201191.88094999996, 41419.92445, 1120.6729999999989, 27064.562149999998, 6759.543450000003, 1151.2815999999998, 5645.972500000001, 70239.99469999998, 2468.0781500000003, 74.00769999999972, 274.57424999999995, 4085.4411499999997, 54892.3609, 20344.5, 4100.955550000003, 306.8878499999999, 598.1379500000002, 98200.96145, 1548.7608999999993, 48371.46090000002, 93.5650500000001, 996.3833999999999, 5999.726849999999, 65061.527849999984, 1343.6522999999995, 2444.3842, 20702.087949999997, 41612.6938, 8716.784449999997, 35314.11059999999, 1057.09515, 679.1063999999998, 32340.461000000007, 107332.58170000001, 564.0283000000005, 308.6485500000002, 6411.314050000004, 16892.101950000004, 2804.765700000001, 4544.119050000003, 848.3638999999996, 22555.577249999988, 704.9074499999999, 2714.924900000001, 400.17665000000056, 3047.8062999999997, 672.6902500000001, 1154.583900000002, 159.42344999999992, 2678.627699999999, 53877.39709999998, 6252.4588, 13623.237050000003, 1205.1451499999996, 3420.360850000001, 64.22899999999954, 1196.9678999999999, 5284.887600000001, 21816.5393, 40446.13375, 1009.3892000000008, 758.1965500000001, 13942.263000000003, 26605.372250000004, 1544.6851499999987, 11569.050200000003, 27346.60955, 7509.192800000006, 7443.993800000002, 187.2916000000001, 430.38455000000033, 1731.0626999999993, 22365.95915, 6107.7645999999995, 4146.846700000001, 5192.632750000001, 177.79995000000005, 2192.3364500000007, 30382.73005, 5084.077300000002, 24334.4352, 13273.328150000001, 19199.403049999997, 258.82960000000026, 171.6086499999996, 2579.294149999999, 10643.136300000002, 5276.648400000001, 130754.4461, 25967.8492, 98401.47165, 5083.535600000001, 620.5996500000009, 200.7367, 109093.38190000002, 14135.86125, 3862.85095, 1893.2924500000001, 1645.1986999999992, 3865.672500000002, 967.6888999999992, 2119.0998500000005, 1230.5245000000007, 1788.7488000000008, 693.7150500000005, 1072.0571000000002, 8818.327500000001, 1024.2146999999995, 25633.0359, 23320.623299999996, 673.1012000000009, 2222.3002000000015, 61014.46195, 1798.2479000000003, 84.1216999999999, 12116.474599999998, 4210.0095999999985, 314.3817499999999, 447.9805499999999, 228.29715000000022, 857.4145500000011, 519.8693000000002, 1650.575849999999, 5387.092499999999, 3240.4637500000003, 277831.7656500001, 6361.4247, 221.1024500000001, 15152.461450000003, 74614.90974999998, 4723.702600000005, 1759.2794500000005, 7691.003450000003, 449.2458000000002, 4290.22515, 49.50310000000002, 2441.497700000003, 95.18614999999988, 4379.470049999998, 2092.353499999998, 331.8804999999998, 3466.876549999998, 661.835500000002, 120996.0934, 629.6973000000003, 10204.53555, 3189.4067999999993, 1626.1462500000007, 311.83700000000005, 6595.661949999999, 766.3864500000003, 5832.719049999997, 622.8069000000003, 7538.644450000001, 90435.91854999997, 691.8538499999994, 19856.873300000003, 535.360100000001, 626.5313499999994, 160407.01739999998, 1773.1797999999994, 23848.098950000003, 412.3286000000004, 9071.85025, 77140.08305, 28072.59055000001, 3928.6560000000018, 928.5198500000002, 150.85995000000014, 11993.837499999998, 1933.3202499999993, 10484.912499999999, 104.0705, 2030.9136499999997, 995.7477499999995, 12849.491750000001, 2338.2236, 87.6514499999999, 24800.063850000002, 25153.20225, 1055.6573000000005, 694.9957499999987, 17828.574000000004, 76.1506500000003, 2596.9522000000006, 21308.426099999993, 3866.2829500000007, 3592.980500000001, 125.80120000000004, 296.64504999999923, 147.27640000000002, 16730.887099999993, 894.561599999999, 9031.985350000003, 2691.973500000001, 266.99935000000016, 2960.777250000001, 6210.0092, 433.22290000000044, 59792.20055, 4647.302199999999, 4789.46715, 3166.24635, 982.3338500000001, 5318.79285, 1205.97105, 30287.507150000005, 2542.6319000000003, 18771.75479999999, 988.8504000000003, 12833.495099999998, 6200.59555, 362.6848000000004, 1644.2095499999996, 4357.1161, 1328.4182000000008, 4486.79725, 40020.7544, 97421.5079, 12820.688849999999, 2030.1669000000004, 2788.8766499999983, 6761.3549, 1066.2487, 24087.15335, 466.37290000000036, 16821.8598, 149.65445000000034, 1246.0752999999997, 2882.825749999999, 26858.9004, 528.0869999999999, 19324.892450000003, 15629.004099999998, 98778.0215, 519.7051000000002, 43175.931500000006, 515.3512000000003, 1439.2336, 7132.1326, 1231.4716000000012, 486.8542000000008, 1815.2694999999983, 12663.202550000007, 59650.16855, 4806.4469500000005, 4913.544050000002, 8088.978949999999, 495.26579999999933, 11617.636250000003, 4081.1772499999997, 160451.48539999995, 16713.16865, 18227.059300000008, 26672.41565000001, 1043.1385999999998, 425.01955000000004, 130230.89709999993, 628.2395999999999, 22252.702399999995, 484.91110000000015, 210.3083500000005, 26.957550000000033, 5888.0771, 2141.0891, 220863.50235000002, 5737.141799999999, 3993.392899999999, 1562.9962500000004, 49597.06825, 128177.13039999997, 28657.39415000001, 1132.2413499999998, 26731.31054999999, 1059.2594, 4745.592499999999, 1920.1159499999983, 127.58095000000012, 12190.44565, 555.7345500000004, 9237.729949999997, 46.56134999999999, 236.1499999999998, 4298.163950000001, 216726.06719999996, 62.61789999999998, 2463.3468, 58960.8349, 5199.69665, 9304.32905, 9313.074099999998, 255.92869999999982, 69.30369999999995, 6764.494600000001, 6947.59405, 9963.741200000004, 2287.71385, 109.46640000000002, 2293.3109999999997, 16593.583899999998, 24518.62785, 355.55969999999905, 20953.521099999998, 2855.7088999999996, 3858.7823000000003, 8844.460499999997]
plt.hist(area_list, bins=256, normed=0, facecolor='black', edgecolor='black', alpha=1, histtype = 'bar')
plt.savefig(self.histo_img_path)
3.4 plt图像存储
plt.hist(salary, group, histtype='bar', rwidth=0.8)
plt.legend()
plt.xlabel('salary-group')
plt.ylabel('salary')
plt.title(u'测试例子——直方图', FontProperties=font)
用相应的文件,进行输入与测试。
if not os.path.isdir(self.histo_img_dir):
os.makedirs(self.histo_img_dir)
area_list=[235939.0035, 64153.92420000001, 797.3219000000005, 40377.498349999994, 825.0993000000005, 122283.17504999999, 110586.77519999997, 23235.967249999994, 7660.271999999997, 2845.3616000000015, 5078.0296, 39.01750000000018, 13050.5062, 1644.8198499999994, 9904.229450000003, 2675.0394999999994, 201191.88094999996, 41419.92445, 1120.6729999999989, 27064.562149999998, 6759.543450000003, 1151.2815999999998, 5645.972500000001, 70239.99469999998, 2468.0781500000003, 74.00769999999972, 274.57424999999995, 4085.4411499999997, 54892.3609, 20344.5, 4100.955550000003, 306.8878499999999, 598.1379500000002, 98200.96145, 1548.7608999999993, 48371.46090000002, 93.5650500000001, 996.3833999999999, 5999.726849999999, 65061.527849999984, 1343.6522999999995, 2444.3842, 20702.087949999997, 41612.6938, 8716.784449999997, 35314.11059999999, 1057.09515, 679.1063999999998, 32340.461000000007, 107332.58170000001, 564.0283000000005, 308.6485500000002, 6411.314050000004, 16892.101950000004, 2804.765700000001, 4544.119050000003, 848.3638999999996, 22555.577249999988, 704.9074499999999, 2714.924900000001, 400.17665000000056, 3047.8062999999997, 672.6902500000001, 1154.583900000002, 159.42344999999992, 2678.627699999999, 53877.39709999998, 6252.4588, 13623.237050000003, 1205.1451499999996, 3420.360850000001, 64.22899999999954, 1196.9678999999999, 5284.887600000001, 21816.5393, 40446.13375, 1009.3892000000008, 758.1965500000001, 13942.263000000003, 26605.372250000004, 1544.6851499999987, 11569.050200000003, 27346.60955, 7509.192800000006, 7443.993800000002, 187.2916000000001, 430.38455000000033, 1731.0626999999993, 22365.95915, 6107.7645999999995, 4146.846700000001, 5192.632750000001, 177.79995000000005, 2192.3364500000007, 30382.73005, 5084.077300000002, 24334.4352, 13273.328150000001, 19199.403049999997, 258.82960000000026, 171.6086499999996, 2579.294149999999, 10643.136300000002, 5276.648400000001, 130754.4461, 25967.8492, 98401.47165, 5083.535600000001, 620.5996500000009, 200.7367, 109093.38190000002, 14135.86125, 3862.85095, 1893.2924500000001, 1645.1986999999992, 3865.672500000002, 967.6888999999992, 2119.0998500000005, 1230.5245000000007, 1788.7488000000008, 693.7150500000005, 1072.0571000000002, 8818.327500000001, 1024.2146999999995, 25633.0359, 23320.623299999996, 673.1012000000009, 2222.3002000000015, 61014.46195, 1798.2479000000003, 84.1216999999999, 12116.474599999998, 4210.0095999999985, 314.3817499999999, 447.9805499999999, 228.29715000000022, 857.4145500000011, 519.8693000000002, 1650.575849999999, 5387.092499999999, 3240.4637500000003, 277831.7656500001, 6361.4247, 221.1024500000001, 15152.461450000003, 74614.90974999998, 4723.702600000005, 1759.2794500000005, 7691.003450000003, 449.2458000000002, 4290.22515, 49.50310000000002, 2441.497700000003, 95.18614999999988, 4379.470049999998, 2092.353499999998, 331.8804999999998, 3466.876549999998, 661.835500000002, 120996.0934, 629.6973000000003, 10204.53555, 3189.4067999999993, 1626.1462500000007, 311.83700000000005, 6595.661949999999, 766.3864500000003, 5832.719049999997, 622.8069000000003, 7538.644450000001, 90435.91854999997, 691.8538499999994, 19856.873300000003, 535.360100000001, 626.5313499999994, 160407.01739999998, 1773.1797999999994, 23848.098950000003, 412.3286000000004, 9071.85025, 77140.08305, 28072.59055000001, 3928.6560000000018, 928.5198500000002, 150.85995000000014, 11993.837499999998, 1933.3202499999993, 10484.912499999999, 104.0705, 2030.9136499999997, 995.7477499999995, 12849.491750000001, 2338.2236, 87.6514499999999, 24800.063850000002, 25153.20225, 1055.6573000000005, 694.9957499999987, 17828.574000000004, 76.1506500000003, 2596.9522000000006, 21308.426099999993, 3866.2829500000007, 3592.980500000001, 125.80120000000004, 296.64504999999923, 147.27640000000002, 16730.887099999993, 894.561599999999, 9031.985350000003, 2691.973500000001, 266.99935000000016, 2960.777250000001, 6210.0092, 433.22290000000044, 59792.20055, 4647.302199999999, 4789.46715, 3166.24635, 982.3338500000001, 5318.79285, 1205.97105, 30287.507150000005, 2542.6319000000003, 18771.75479999999, 988.8504000000003, 12833.495099999998, 6200.59555, 362.6848000000004, 1644.2095499999996, 4357.1161, 1328.4182000000008, 4486.79725, 40020.7544, 97421.5079, 12820.688849999999, 2030.1669000000004, 2788.8766499999983, 6761.3549, 1066.2487, 24087.15335, 466.37290000000036, 16821.8598, 149.65445000000034, 1246.0752999999997, 2882.825749999999, 26858.9004, 528.0869999999999, 19324.892450000003, 15629.004099999998, 98778.0215, 519.7051000000002, 43175.931500000006, 515.3512000000003, 1439.2336, 7132.1326, 1231.4716000000012, 486.8542000000008, 1815.2694999999983, 12663.202550000007, 59650.16855, 4806.4469500000005, 4913.544050000002, 8088.978949999999, 495.26579999999933, 11617.636250000003, 4081.1772499999997, 160451.48539999995, 16713.16865, 18227.059300000008, 26672.41565000001, 1043.1385999999998, 425.01955000000004, 130230.89709999993, 628.2395999999999, 22252.702399999995, 484.91110000000015, 210.3083500000005, 26.957550000000033, 5888.0771, 2141.0891, 220863.50235000002, 5737.141799999999, 3993.392899999999, 1562.9962500000004, 49597.06825, 128177.13039999997, 28657.39415000001, 1132.2413499999998, 26731.31054999999, 1059.2594, 4745.592499999999, 1920.1159499999983, 127.58095000000012, 12190.44565, 555.7345500000004, 9237.729949999997, 46.56134999999999, 236.1499999999998, 4298.163950000001, 216726.06719999996, 62.61789999999998, 2463.3468, 58960.8349, 5199.69665, 9304.32905, 9313.074099999998, 255.92869999999982, 69.30369999999995, 6764.494600000001, 6947.59405, 9963.741200000004, 2287.71385, 109.46640000000002, 2293.3109999999997, 16593.583899999998, 24518.62785, 355.55969999999905, 20953.521099999998, 2855.7088999999996, 3858.7823000000003, 8844.460499999997]
plt.hist(area_list, bins=512, normed=0, facecolor='black', edgecolor='black', alpha=1, histtype = 'bar')
plt.legend()
plt.xlabel('badcase size')
plt.ylabel('badcase numbers')
plt.title('class '+str(1)+'badcase histogram')
img_name=self.histo_img_dir+'class1.jpg'
plt.savefig(img_name)
plt.hist(area_list, bins=10, normed=0, facecolor='black', edgecolor='black', alpha=1, histtype = 'bar')
plt.legend()
plt.xlabel('badcase size')
plt.ylabel('badcase numbers')
plt.title('class '+str(2)+'badcase histogram')
img_name=self.histo_img_dir+'class2.jpg'
plt.savefig(img_name)
存储之后,务必记得删掉相应的plt.close('all'),不然生成的直方图非常类似。
3.4 直方图的值
可以print出一些大致查看一下。
[683.9160000000005, 2442.46605, 14484.758699999993, 3386.5678499999995, 14479.344549999996, 13515.022249999998, 7965.106, 5678.810250000002, 2327.091099999999, 13631.614450000001, 2796.608800000001, 34886.04705, 325.50250000000017, 34519.20375, 1698.1252500000007, 48642.866050000026,
71689.66249999999, 1773.4906499999995, 492.9880500000001, 109111.37125000001, 1019.5727999999996, 1242.2530000000004, 4899.727649999999, 8881.890700000002, 930.5868999999998, 848.3562, 2310.67225, 603.83655, 1731.6071000000013, 24791.8876, 1082.5951500000006, 6472.634000000001, 94612.16660000001, 691.79215, 4489.496849999999, 5564.19405, 1092.7272499999997, 22771.6285, 1747.8156000000008, 1473.8869999999995, 11573.610550000003, 5180.9365, 72986.89145000002, 210.46294999999998, 3841.575599999999, 497.43615000000017, 5046.022349999997, 1744.5338500000003, 1092.3092000000001, 1286.9624500000007, 434.8083500000001,
8087.406949999999, 1670.8321500000004, 4459.141700000001, 1989.5381500000003, 592.2093, 846.8612499999999, 389.14055000000036, 30712.36465000001, 14510.547649999999, 87553.47919999997, 16214.509000000002, 52923.730299999996, 34677.3077, 32366.462899999988, 602.9749500000005, 241.78099999999992,
1190.1633999999995, 2205.7668000000003, 1239.5366, 1396.8755, 9500.852600000002, 20389.856850000004, 3651.285800000002, 95486.64685000003, 6979.759900000002, 8463.49585, 28137.6279, 12057.38095, 8713.739650000001, 6117.859549999999, 11247.828699999998, 4633.14075, 2064.767450000002, 964.5043000000002, 613.1057,
1979.5362500000003, 2753.947499999998, 20320.293299999983, 3211.1752999999985, 1601.1844000000003, 55942.1499, 16455.539300000004, 23301.4676, 1834.5064000000004, 4534.887249999999, 1219.8842499999998, 1868.28045, 1552.0870000000004, 5627.17175,
10954.283500000005, 91.5470000000001, 1237.39605, 2055.6168, 2427.7046000000005, 2742.52815, 7729.81905, 61379.4444, 2242.7182000000007, 776.7938500000002, 25998.50349999999, 11309.197699999997, 19003.994399999996, 500.52919999999864,
553.5479500000002, 337.13875000000047, 3546.14375, 834.4200999999995, 2907.019050000001, 4721.70215, 24307.453050000004, 30627.230450000003, 64338.71099999998, 68099.72225000002, 1321.9788999999992, 4787.533700000001, 21151.617250000007, 9178.8901, 4339.022200000003, 2396.86255, 802.7199499999999,
16676.49185, 1824.0607499999999, 1507.88895, 587.7183999999996, 2571.5704, 2102.1658500000003, 12234.506650000001, 772.6651500000014, 4435.3801, 5508.0989000000045, 3142.5754, 1811.7970500000013, 3051.871, 3727.1215999999986, 18412.6569, 499.1101, 19067.370849999992, 12836.944349999998, 5653.223150000002, 2481.26605, 1582.50975, 2423.39585, 15213.736899999994, 28413.067950000004,
576.3890499999994, 201.07575000000003, 11694.643699999997, 11040.114499999998, 543.3011500000001, 44412.159199999995, 544.8582000000001, 673.0482999999999, 14934.810599999999, 4994.224450000001, 516.8612000000005, 7971.805999999999, 61756.51254999999, 522.83385, 451.03904999999986, 7023.577050000001,
7265.986849999999, 61958.96604999999, 5680.64175, 9141.920350000002, 15217.30065, 602.6508999999999, 50731.444700000015, 3063.1288500000023, 41144.99094999999, 20797.686599999997, 867.4010000000002, 26819.547, 796.9999499999998, 10562.143299999998, 3913.147049999999]
[1724.1692499999997, 366.15034999999966, 15802.880550000003, 1225.0612, 5476.302099999996, 610.3301500000001, 429.36165000000045, 733.2061000000003, 1944.4293500000003, 22528.827299999997, 2047.4176500000003, 4414.537699999999, 47382.45214999999, 2869.1595500000008, 43092.030199999994, 1618.52535, 367.96160000000003, 5022.433500000001, 1605.3408, 346.34710000000075, 5044.8439499999995, 1321.19305, 3159.83075, 2618.5579500000026, 8377.59445, 10347.806049999994, 38315.6847, 833.8229499999998, 620.6976499999996,
6355.030249999999, 302.02639999999985, 1520.7268499999996, 1060.9626, 8535.399400000002, 990.3860999999995, 14585.555250000005, 181.53479999999993, 2383.0066000000006, 439.6647499999999, 170.79249999999968, 54813.32545, 1833.8056500000005, 5240.37735, 5066.245699999999, 42476.40844999998, 1260.8629999999998, 336.687600000001, 1609.8348999999998, 2977.436950000002, 1205.3774499999984, 693.5048000000007, 8646.50815, 482.7517999999998,
1578.913200000001]
[351.598449999999, 455.98845000000006, 126.78010000000019, 514.8395000000003, 406.4549999999999, 7550.0779, 84.9699000000001, 9.678950000000109, 35.56769999999989, 39.973200000000126, 394.5030499999988, 100.25860000000007, 37.878299999999896, 33.97390000000004, 248.32479999999987, 513.4488999999992, 139.4486499999998, 311.90315000000027, 428.46459999999956, 869.41345,
243.80120000000008, 110.08284999999972, 1920.022200000001, 1054.8499499999994, 15162.020400000001, 185.6614999999997, 63.12685000000007, 224.28239999999988, 345.07704999999976, 257.955849999999, 3818.948149999999, 38.15974999999986, 2438.069849999999, 247.61110000000124, 4717.834649999999, 2854.0712999999982, 13423.968249999994, 703.90825, 32.716900000000166, 322.78010000000023, 188.23489999999902, 776.5165999999997, 706.7350999999991, 174.32570000000058, 169.27564999999984, 421.2784000000006, 40.16000000000007, 171.9341000000002, 4082.148650000001, 49.973049999999944, 2478.508799999998, 12.463999999999809, 29.90400000000009, 32.42195000000007, 2013.7202000000023, 1111.2301499999999, 951.9321500000003, 5501.7800000000025, 19054.2669, 1063.02525, 1231.7002999999972, 108.1362, 2642.0498000000016, 362.2731, 14.572200000000047, 411.22345, 150.87795000000008, 94.12419999999995, 2965.7859999999982, 99.06964999999985, 1374.7440999999978, 447.42525000000023, 73.95509999999983, 17757.11055, 13.371199999999863, 26045.760599999998, 75.52944999999983, 319.91989999999953, 690.5670500000001, 651.0274999999993, 571.9453500000006, 19.095449999999886,
430.0609000000003, 472.3264499999994, 14.139350000000046, 3329.5093500000003, 8418.920500000004, 156.1894499999998, 4515.5091, 203.52659999999946, 182.8668000000001, 8054.924750000002, 62.799449999999986, 40.03914999999982, 15.188800000000015, 130.68259999999958, 343.4634499999999, 46.27455000000004, 180.10404999999986, 14429.936999999994, 19.00760000000006, 1212.6895999999997, 1272.8396999999989, 10439.520400000009, 4912.1141, 1462.5064, 83.44374999999991, 234.65625000000037, 54.64059999999992, 2391.116800000001, 116.79520000000005, 77.21045000000025, 1516.4389499999997, 73.30219999999991, 89.63220000000037, 136.05375000000046, 32780.061699999984, 106.98255000000017, 1452.5062499999997, 103.90935000000012, 319.4284000000001, 16.047649999999976, 6393.757299999998]
四、图片URL
4.1 标注的格式
标注之中,图片的名称的格式:
{"info": {"description": "COCO 2014 Dataset","url": "http://cocodataset.org","version": "1.0","year": 2014,"contributor": "COCO Consortium","date_created": "2017/
09/01"},"images": [{"license": 3,"file_name": "COCO_val2014_000000391895.jpg","coco_url": "http://images.cocodataset.org/val2014/COCO_val2014_000000391895.jpg","h
eight": 360,"width": 640,"date_captured": "2013-11-14 11:18:45","flickr_url": "http://farm9.staticflickr.com/8186/8119368305_4e622c8349_z.jpg","id": 391895},{"lic
ense": 4,"file_name": "COCO_val2014_000000522418.jpg","coco_url": "http://images.cocodataset.org/val2014/COCO_val2014_000000522418.jpg","height": 480,"width": 640
,"date_captured": "2013-11-14 11:38:44","flickr_url": "http://farm1.staticflickr.com/1/127244861_ab0c0381e7_z.jpg","id": 522418},{"license": 3,"file_name": "COCO_
val2014_000000184613.jpg","coco_url": "http://images.cocodataset.org/val2014/COCO_val2014_000000184613.jpg","height": 336,"width": 500,"date_captured": "2013-11-1
4 12:36:29","flickr_url": "http://farm3.staticflickr.com/2169/2118578392_1193aa04a0_z.jpg","id": 184613},{"license": 3,"file_name": "COCO_val2014_000000318219.jpg
","coco_url": "http://images.cocodataset.org/val2014/COCO_val2014_000000318219.jpg","height": 640,"width": 556,"date_captured": "2013-11-14 13:02:53","flickr_url"
: "http://farm5.staticflickr.com/4125/5094763076_813ea2751b_z.jpg","id": 318219},{"license": 3,"file_name": "COCO_val2014_000000554625.jpg","coco_url": "http://im
ages.cocodataset.org/val2014/COCO_val2014_000000554625.jpg","height": 640,"width": 426,"date_captured": "2013-11-14 16:03:19","flickr_url": "http://farm5.staticfl
ickr.com/4086/5094162993_8f59d8a473_z.jpg","id": 554625},{"license": 4,"file_name": "COCO_val2014_000000397133.jpg","coco_url": "http://images.cocodataset.org/val
2014/COCO_val2014_000000397133.jpg","height": 427,"width": 640,"date_captured": "2013-11-14 17:02:52","flickr_url": "http://farm7.staticflickr.com/6116/6255196340
_da26cf2c9e_z.jpg","id": 397133},{"license": 3,"file_name": "COCO_val2014_000000574769.jpg","coco_url": "http://images.cocodataset.org/val2014/COCO_val2014_000000
574769.jpg","height": 640,"width": 480,"date_captured": "2013-11-14 17:07:59","flickr_url": "http://farm8.staticflickr.com/7010/6728227647_3d5a0d55ee_z.jpg","id":
574769},{"license": 4,"file_name": "COCO_val2014_000000060623.jpg","coco_url": "http://images.cocodataset.org/val2014/COCO_val2014_000000060623.jpg","height": 42
7,"width": 640,"date_captured": "2013-11-14 17:24:15","flickr_url": "http://farm7.staticflickr.com/6080/6113512699_37b4c98473_z.jpg","id": 60623},{"license": 2,"f
ile_name": "COCO_val2014_000000309022.jpg","coco_url": "http://images.cocodataset.org/val2014/COCO_val2014_000000309022.jpg","height": 480,"width": 640,"date_capt
ured": "2013-11-14 17:28:23","flickr_url": "http://farm4.staticflickr.com/3790/10167396295_e63f2856d0_z.jpg","id": 309022},{"license": 2,"file_name": "COCO_val201
4_000000005802.jpg","coco_url": "http://images.cocodataset.org/val2014/COCO_val2014_000000005802.jpg","height": 479,"width": 640,"date_captured": "2013-11-14 17:2
8:25","flickr_url": "http://farm4.staticflickr.com/3810/9614287841_1b724dbbc5_z.jpg","id": 5802},{"license": 2,"file_name": "COCO_val2014_000000222564.jpg","coco_
url": "http://images.cocodataset.org/val2014/COCO_val2014_000000222564.jpg","height": 480,"width": 640,"date_captured": "2013-11-14 17:30:34","flickr_url": "http:
//farm8.staticflickr.com/7390/10166966765_c96225b556_z.jpg","id": 222564},{"license": 1,"file_name": "COCO_val2014_000000118113.jpg","coco_url": "http://images.co
codataset.org/val2014/COCO_val2014_000000118113.jpg","height": 640,"width": 480,"date_captured": "2013-11-14 17:44:50","flickr_url": "http://farm8.staticflickr.co
m/7030/6555665525_b242809dc2_z.jpg","id": 118113},
4.2 添加输出badcase的URL
coco_url,之前查找img_ID的后面增加查找URL的语句。
# loading json annotation
with open(self.area_annotation_document) as f:
print('loading:', self.area_annotation_document)
instances_val = json.load(f)
print('loading done.')
annotations_list = instances_val['annotations']
images_list = instances_val['images']
coco_categories = instances_val['categories']
# from predict idx to json category id list
predict_idx_to_json_id = {}
for idx in range(len(names)):
predict_idx_to_json_id[idx] = coco_categories[idx]['id']
# from image name find image id
def from_image_name_find_id_and_URL(file_name, images_list):
for image_idx in range(len(images_list)):
# if (image_idx%10000==0):
# print('from image_name find id:',image_idx,'/',len(images_list))
if file_name == images_list[image_idx]['file_name']:
img_id = images_list[image_idx]['id']
coco_url=images_list[image_idx]['coco_url']
break
return img_id,coco_url
4.3 ULR的存储
存入dict之中,
# generate label true predict negative area list dict
ltrue_pnegative_catagory_area_dict={}
coco_badcase_img_url_dict={}
for category_idx in range(self.class_num):
ltrue_pnegative_catagory_area_dict[category_idx] = []
coco_badcase_img_url_dict[category_idx]=[]
for idx in range(len(self.ture_negative_name_dict[category_idx])):
if idx%300==0:
print('category:',category_idx,'finding area:',idx,'/',len(self.ture_negative_name_dict[category_idx]))
img_id,coco_url=from_image_name_find_id_and_URL(file_name=self.ture_negative_name_dict[category_idx][idx], images_list=images_list)
ltrue_pnegative_catagory_area_dict[category_idx].append(from_id_and_class_find_area(img_id=img_id, class_id=category_idx, annotations_list=annotations_list))
coco_badcase_img_url_dict[category_idx].append(coco_url)
# save coco_url into dict and pkl
if not os.path.exists(self.badcase_coco_url_path):
with open(self.badcase_coco_url_path, 'wb') as f:
print('writing to', self.badcase_coco_url_path)
pickle.dump(coco_badcase_img_url_dict, f)
4.4 本地print
生成数组随机读出相应的图片
生成一个随机数
https://www.runoob.com/python3/python3-random-number.html
random.randint(a,b) 生成a,b之间的一个随机整数
序列乱序
>>> import random
>>> a=[1,2,3,4,5]
>>> a
[1, 2, 3, 4, 5]
>>> random.shuffle(a)
>>> a
[5, 4, 2, 1, 3]
生成1到n的列表
b=list(range(100))
>>> b
[0, 1, 2, 3, 4, 5, 6, 7, 8, 9, 10, 11, 12, 13, 14, 15, 16, 17, 18, 19, 20, 21, 22, 23, 24, 25, 26, 27, 28, 29, 30, 31, 32, 33, 34, 35, 36, 37, 38, 39, 40, 41, 42, 43,
44, 45, 46, 47, 48, 49, 50, 51, 52, 53, 54, 55, 56, 57, 58, 59, 60, 61, 62, 63, 64, 65, 66, 67, 68, 69, 70, 71, 72, 73, 74, 75, 76, 77, 78, 79, 80, 81, 82, 83, 84,
85, 86, 87, 88, 89, 90, 91, 92, 93, 94, 95, 96, 97, 98, 99]
"""
created by xingxiangrui on 2019.5.23
this program is to :
read badcase coco url and print some of them
"""
import torch.utils.data as data
import json
import os
import subprocess
from PIL import Image
import numpy as np
import torch
import pickle
from util import *
import pandas as pd
import matplotlib.pyplot as plt
import warnings
import random
class coco_url_print():
def __init__(self):
# super(self).__init__()
warnings.simplefilter("ignore")
self.read_and_write_dir='/Users/Desktop/code/chun_ML_GCN/badcase_analyse/cls_gat_hist/'
self.url_pkl_file_name='badcase_coco_url.pkl'
self.url_pkl_file_path=self.read_and_write_dir+self.url_pkl_file_name
self.output_category=24
self.output_num=3
# load and print coco url
def run_coco_url_print(self):
# loadint files
with open(self.url_pkl_file_path,'rb') as f:
print('loading ',self.url_pkl_file_path)
coco_url_dict=pickle.load(f)
random_idx=list(range(len(coco_url_dict[self.output_category])))
random.shuffle(random_idx)
print('category:', self.output_category,'output num:',self.output_num)
for output_idx in range(self.output_num):
print(coco_url_dict[self.output_category][random_idx[output_idx]])
print('program end...')
if __name__ == '__main__':
coco_url_print().run_coco_url_print()
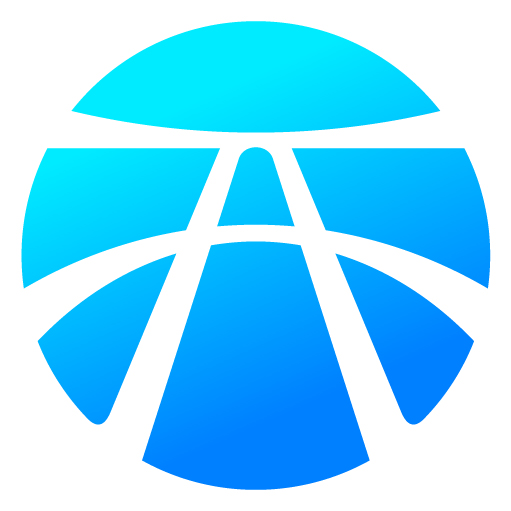
开放原子开发者工作坊旨在鼓励更多人参与开源活动,与志同道合的开发者们相互交流开发经验、分享开发心得、获取前沿技术趋势。工作坊有多种形式的开发者活动,如meetup、训练营等,主打技术交流,干货满满,真诚地邀请各位开发者共同参与!
更多推荐
所有评论(0)