表格解析算法——PaddlePaddle之RARE
百度paddlepaddlepaddleocr下pp-structure包含了版面分析及表格解析两项工作,本文是对表格解析的技术详述。代码:https://github.com/PaddlePaddle/PaddleOCR简要概览:PaddleOCR新发版v2.2:开源版面分析与轻量化表格识别_飞桨PaddlePaddle的博客-CSDN博客RARE百度paddlepaddle包含表格解析功能,被
百度paddlepaddle
paddleocr下pp-structure包含了版面分析及表格解析两项工作,本文是对表格解析的技术详述。
代码:
https://github.com/PaddlePaddle/PaddleOCR
简要概览:
PaddleOCR新发版v2.2:开源版面分析与轻量化表格识别_飞桨PaddlePaddle的博客-CSDN博客
RARE
百度paddlepaddle包含表格解析功能,被称为RERE算法。RARE算法原本用于进行文本识别,是一个img2seq任务,修改该网络head部分,分成表格描述和单元格定位两个任务,这两个任务共享了backbone的输出及head中一部分attention信息。“图片由表格结构和cell坐标预测模型拿到表格的结构信息和单元格的坐标信息”,最后输出表格的HTML描述。
一个完整的表格解析工程需要用到四个模型:版面分析模型、文本定位模型、文本识别模型、表格结构解析模型。
版面分析模型:飞桨用到了yolov2检测模型,对文档图片中的文本、表格、图片、标题与列表区域进行检测。当前主流是用分割做。
文本定位模型、文本识别模型:可使用其他定位识别模型。
表格结构解析模型:该技术的精髓所在。
如何进行训练:
python3 tools/train.py -c configs/table/table_mv3.yml
所有的模型训练都会用到这个train文件,可以视为一个主分支,根据配置文件调用不同的次分支。
# 统一化的处理配置、创建文件夹等
config, device, logger, vdl_writer = program.preprocess(is_train=True)
# 加载数据集、后处理、搭建模型、损失、优化器、执行训练等
main(config, device, logger, vdl_writer)
如何进行推理:
python3 ppstructure/table/predict_table.py
--det_model_dir=./inference/ch_PP-OCRv2_det_infer # 检测模型
--rec_model_dir=./inference/ch_PP-OCRv2_rec_infer # 识别模型
--table_model_dir=./inference/en_ppocr_mobile_v2.0_table_structure_infer # 表格结构识别模型
--image_dir=./doc/imgs/163558403291484de11ac8c.jpg # 测试图片
--rec_char_dict_path=./ppocr/utils/ppocr_keys_v1.txt # 识别词表,6623字符
--table_char_dict_path=./ppocr/utils/dict/table_structure_dict.txt # 表格结构词表,实际只用其中28个表格描述符
--det_limit_side_len=960 # 两个参数限制图像最短边为960,否则resize
--det_limit_type=min
--output ./output/table # 输出表格文件路径
推理中的det_limit_side_len与det_limit_type参数:
参数默认设置为`limit_type='max', det_limit_side_len=960`。表示网络输入图像的最长边不能超过960,如果超过这个值,会对图像做等宽比的resize操作,确保最长边为`det_limit_side_len`。
设置为`limit_type='min', det_limit_side_len=960` 则表示限制图像的最短边为960。
表格结构词表
table_structure_dict.txt 第0行是 277 28 1267 1186,第1行到277行为表格内字符,实际未用到,第278行开始28个为表格结构字符。限制了这个可解析表格的大小空间跨行跨列最大为10,没有跨1行或跨1列的字符。
词表中有28种表格结构符,模型为30分类,在分类中argmax=1,为<thead>,argmax=0、29 代表beg、end。
<thead>
<tr>
<td> 单元格开始
</td> 单元格结束
</tr>
</thead>
<tbody>
</tbody>
<td
colspan="5". # 横跨5列
>
colspan="2"
colspan="3"
rowspan="2"。 # 横跨2行
colspan="4"
colspan="6"
rowspan="3"
colspan="9"
colspan="10"
colspan="7"
rowspan="4"
rowspan="5"
rowspan="9"
colspan="8"
rowspan="8"
rowspan="6"
rowspan="7"
rowspan="10"
不包含的html描述:
<b>加粗文本
模型的结构
main函数调用build_model调用BaseModel,先后进行输入预处理(表格解析没有做这步)、backbone、neck(表格解析没有这一步)、head、输出
paddleocr/PaddleOCR-release-2.4/ppocr/modeling/architectures/__init__.py 调用BaseModel
配置文件模型参数为:
Architecture:
model_type: table
algorithm: TableAttn
Backbone:
name: MobileNetV3
scale: 1.0
model_name: large
Head:
name: TableAttentionHead
hidden_size: 256
l2_decay: 0.00001
loc_type: 2
max_text_length: 100
max_elem_length: 800
max_cell_num: 500
很多paper中经常把一个网络分为几个部分组成backbone、head、neck等深度学习中的术语解释_t20134297的博客-CSDN博客_深度学习neck
backbone:主干网络,经常是resnet、vgg这种成熟有预训练模型的结构
neck:放在backbone和head间,提取更好的特征
head:预测
bottleneck:瓶颈,输出维度小于输入维度,用于降维
backbone内部结构
为了轻量化,build_backbone为mobilenetv3,参考性不大
PaddleOCR-release-2.4/ppocr/modeling/backbones/rec_mobilenet_v3.py
Attention内部结构
PaddleOCR-release-2.4/ppocr/modeling/heads/table_att_head.py
self.head.out_channels= TableAttentionHead(
(structure_attention_cell): AttentionGRUCell(
(i2h): Linear(in_features=960, out_features=256, dtype=float32)
(h2h): Linear(in_features=256, out_features=256, dtype=float32)
(score): Linear(in_features=256, out_features=1, dtype=float32)
(rnn): GRUCell(990, 256)
)
(structure_generator): Linear(in_features=256, out_features=30, dtype=float32)
(loc_fea_trans): Linear(in_features=256, out_features=801, dtype=float32)
(loc_generator): Linear(in_features=1216, out_features=4, dtype=float32)
)
获取结构信息
第一步切片获取Attention:
(outputs, hidden), alpha = self.structure_attention_cell(hidden, fea, elem_onehots)
第二步将Attention结果进行cat:
output = paddle.concat(output_hiddens, axis=1)
第三步线性层获取结构信息:
structure_probs = self.structure_generator(output)
structure_probs = F.softmax(structure_probs)
输出维度为801*30
获取定位信息,比获取结构信息多了线性层:
第一步基于线性层处理出入特征:
loc_fea = self.loc_fea_trans(loc_fea)
第二步cat上面的Attention获得的output信息:
loc_concat = paddle.concat([output, loc_fea], axis=2)
第三步线性层获取坐标信息:
loc_preds = self.loc_generator(loc_concat)
loc_preds = F.sigmoid(loc_preds)
输出维度为801*4
坐标聚合
定位框和cell的对应关系基于下方2个度量计算,一个cell内多个定位框的排序按照先来后到排,推测是默认从上到下。
compute_iou函数计算Iou,distance函数计算角点距离
distances.append((distance(gt_box, pred_box), 1. - compute_iou(gt_box, pred_box)))
html转xlsx文件
后处理阶段build_post_process——TableLabelDecode
from tablepyxl import tablepyxl # tablepyxl将html读入excel
tablepyxl.document_to_xl(html_table, excel_path)
评估方式
理论上是用树编辑距离,但从build_metric——TableMetric来看,需要完全一致
for bno in range(batch_size):
all_num += 1
if (structure_probs[bno] == structure_labels[bno]).all():
correct_num += 1
损失
TableAttentionLoss由2部分组成
structure_loss :nn.CrossEntropyLoss
loc_loss:F.mse_loss 均方损失
可用loc_loss_giou:GIoU详解_景唯acr-CSDN博客_giou iou
损失权重:
structure_weight: 100.0
loc_weight: 10000.0
数据加载方式
main函数调用build_dataloader
加粗文本
在源码中,用识别模型的<b>及</b>,用的是识别模型的加粗文本识别能,但ch_PP-OCRv2_rec_infer并没有识别加粗文本的能力
HTML填充复原:
class TableSystem(object):
根据单元格开始字符所在的cell定位信息进行坐标聚合,从而进行文本聚合
if text in ['<td>', '<td']:
文本信息填充到单元格结束字符前
if '</td>' in tag:
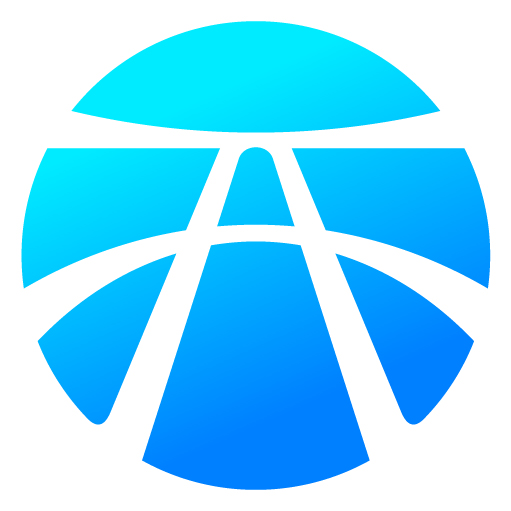
开放原子开发者工作坊旨在鼓励更多人参与开源活动,与志同道合的开发者们相互交流开发经验、分享开发心得、获取前沿技术趋势。工作坊有多种形式的开发者活动,如meetup、训练营等,主打技术交流,干货满满,真诚地邀请各位开发者共同参与!
更多推荐
所有评论(0)