java 1.7支持spark吗,Spark社区可能放弃Spark 1.7而直接发布Spark 2.x
I’m starting a new thread since the other one got intermixed with feature requests. Please refrain from making feature request in this thread. Not that we shouldn’t be adding features, but we can alwa
I’m starting a new thread since the other one got intermixed with feature requests. Please refrain from making feature request in this thread. Not that we shouldn’t be adding features, but we can always add features in 1.7, 2.1, 2.2, ...
First - I want to propose a premise for how to think about Spark 2.0 and major releases in Spark, based on discussion with several members of the community: a major release should be low overhead and minimally disruptive to the Spark community. A major release should not be very different from a minor release and should not be gated based on new features. The main purpose of a major release is an opportunity to fix things that are broken in the current API and remove certain deprecated APIs (examples follow).
For this reason, I would *not* propose doing major releases to break substantial API's or perform large re-architecting that prevent users from upgrading. Spark has always had a culture of evolving architecture incrementally and making changes - and I don't think we want to change this model. In fact, we’ve released many architectural changes on the 1.X line.
If the community likes the above model, then to me it seems reasonable to do Spark 2.0 either after Spark 1.6 (in lieu of Spark 1.7) or immediately after Spark 1.7. It will be 18 or 21 months since Spark 1.0. A cadence of major releases every 2 years seems doable within the above model.
Under this model, here is a list of example things I would propose doing in Spark 2.0, separated into APIs and Operation/Deployment:
APIs
1. Remove interfaces, configs, and modules (e.g. Bagel) deprecated in Spark 1.x.
2. Remove Akka from Spark’s API dependency (in streaming), so user applications can use Akka (SPARK-5293). We have gotten a lot of complaints about user applications being unable to use Akka due to Spark’s dependency on Akka.
3. Remove Guava from Spark’s public API (JavaRDD Optional).
4. Better class package structure for low level developer API’s. In particular, we have some DeveloperApi (mostly various listener-related classes) added over the years. Some packages include only one or two public classes but a lot of private classes. A better structure is to have public classes isolated to a few public packages, and these public packages should have minimal private classes for low level developer APIs.
5. Consolidate task metric and accumulator API. Although having some subtle differences, these two are very similar but have completely different code path.
6. Possibly making Catalyst, Dataset, and DataFrame more general by moving them to other package(s). They are already used beyond SQL, e.g. in ML pipelines, and will be used by streaming also.
Operation/Deployment
1. Scala 2.11 as the default build. We should still support Scala 2.10, but it has been end-of-life.
2. Remove Hadoop 1 support.
3. Assembly-free distribution of Spark: don’t require building an enormous assembly jar in order to run Spark.
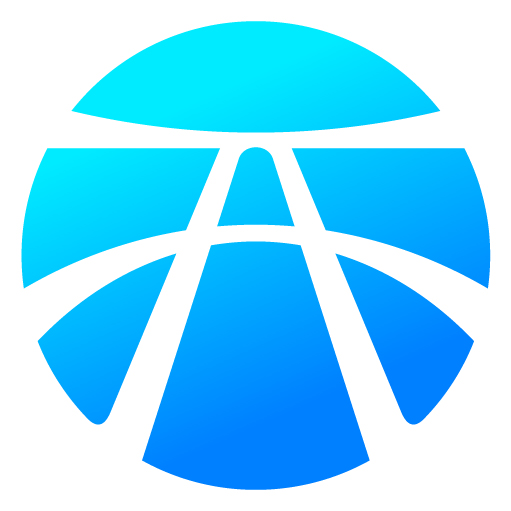
开放原子开发者工作坊旨在鼓励更多人参与开源活动,与志同道合的开发者们相互交流开发经验、分享开发心得、获取前沿技术趋势。工作坊有多种形式的开发者活动,如meetup、训练营等,主打技术交流,干货满满,真诚地邀请各位开发者共同参与!
更多推荐
所有评论(0)