dropout层加在哪里_tensorflow1.0 dropout层
"""Please note, this code is only for python 3+. If you are using python 2+, please modify the code accordingly."""import tensorflow as tffrom sklearn.datasets import load_digitsfrom sklearn.model_sel
"""
Please note, this code is only for python 3+. If you are using python 2+, please modify the code accordingly.
"""
import tensorflow as tf
from sklearn.datasets import load_digits
from sklearn.model_selection import train_test_split
from sklearn.preprocessing import LabelBinarizer
# load data
digits = load_digits()
X = digits.data
y = digits.target
y = LabelBinarizer().fit_transform(y)
X_train, X_test, y_train, y_test = train_test_split(X, y, test_size=.3)
def add_layer(inputs, in_size, out_size, layer_name, activation_function=None, ):
# add one more layer and return the output of this layer
Weights = tf.Variable(tf.random_normal([in_size, out_size]))
biases = tf.Variable(tf.zeros([1, out_size]) + 0.1, )
Wx_plus_b = tf.matmul(inputs, Weights) + biases
# here to dropout
Wx_plus_b = tf.nn.dropout(Wx_plus_b, keep_prob)
if activation_function is None:
outputs = Wx_plus_b
else:
outputs = activation_function(Wx_plus_b, )
return outputs
def compute_accuracy(v_xs,v_ys,v_keep_prob):
global prediction
y_pre = sess.run(prediction,feed_dict={xs:v_xs,keep_prob:v_keep_prob})
correct_prediction = tf.equal(tf.argmax(y_pre,1),tf.argmax(v_ys,1))
accuracy = tf.reduce_mean(tf.cast(correct_prediction,tf.float32))
result = sess.run(accuracy,feed_dict={xs:v_xs,ys:v_ys,keep_prob:v_keep_prob})
return result
# define placeholder for inputs to network
keep_prob = tf.placeholder(tf.float32)
xs = tf.placeholder(tf.float32, [None, 64]) # 8x8
ys = tf.placeholder(tf.float32, [None, 10])
# add output layer
l1 = add_layer(xs, 64, 50, ‘l1‘, activation_function=tf.nn.tanh)
prediction = add_layer(l1, 50, 10, ‘l2‘, activation_function=tf.nn.softmax)
# the loss between prediction and real data
cross_entropy = tf.reduce_mean(-tf.reduce_sum(ys * tf.log(prediction),
reduction_indices=[1])) # loss
train_step = tf.train.GradientDescentOptimizer(0.5).minimize(cross_entropy)
sess = tf.Session()
sess.run(tf.initialize_all_variables())
for i in range(500):
# here to determine the keeping probability
sess.run(train_step, feed_dict={xs: X_train, ys: y_train, keep_prob: 0.5})
if i % 50 == 0:
print(compute_accuracy(X_train, y_train,1),compute_accuracy(X_test, y_test,1))
原文:https://www.cnblogs.com/LiuXinyu12378/p/12495403.html
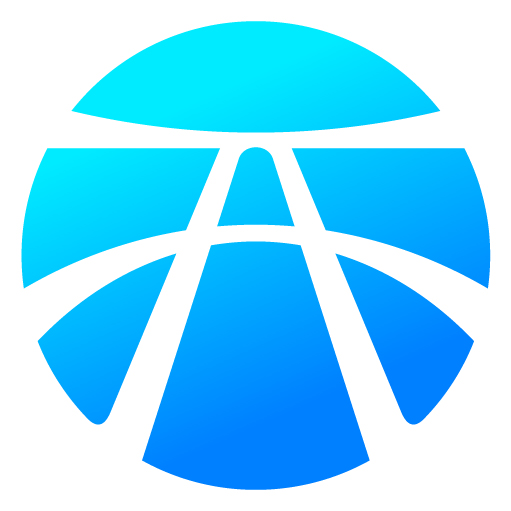
开放原子开发者工作坊旨在鼓励更多人参与开源活动,与志同道合的开发者们相互交流开发经验、分享开发心得、获取前沿技术趋势。工作坊有多种形式的开发者活动,如meetup、训练营等,主打技术交流,干货满满,真诚地邀请各位开发者共同参与!
更多推荐
所有评论(0)